An Information Theoretic Approach forAttention-Driven Face Forgery Detection
European Conference on Computer Vision(2022)
摘要
Recently, Deepfake arises as a powerful tool to fool the existing real-world face detection systems, which has received wide attention in both academia and society. Most existing forgery face detection methods use heuristic clues to build a binary forgery detector, which mainly takes advantage of the empirical observation based on abnormal texture, blending clues, or high-frequency noise, etc.. However, heuristic clues only reflect certain aspects of the forgery, which might lead tomodel bias or sub-optimization. Our recent observations indicate that most of the forgery clues are hidden in the informative region, which can be measured quantitatively by the classic information maximization theory. Motivated by this, we make the first attempt to introduce the self-information metric to enhance the feature representation for forgery detection. The proposed metric can be formulated as a plug-and-play block, termed self-information attention (SIA) module, which can be integrated with most of the top-performance deep models to boost their detection performance. The SIA module can explicitly help the model locate the informative regions and recalibrate channel-wise feature responses, which improves both model's performance and generalizationwith few additional parameters. Extensive experiments on several large-scale benchmarks demonstrate the superiority of the proposed method against the state-of-the-art competitors.
更多查看译文
关键词
Face forgery detection, Information maximization, Attention mechanism
AI 理解论文
溯源树
样例
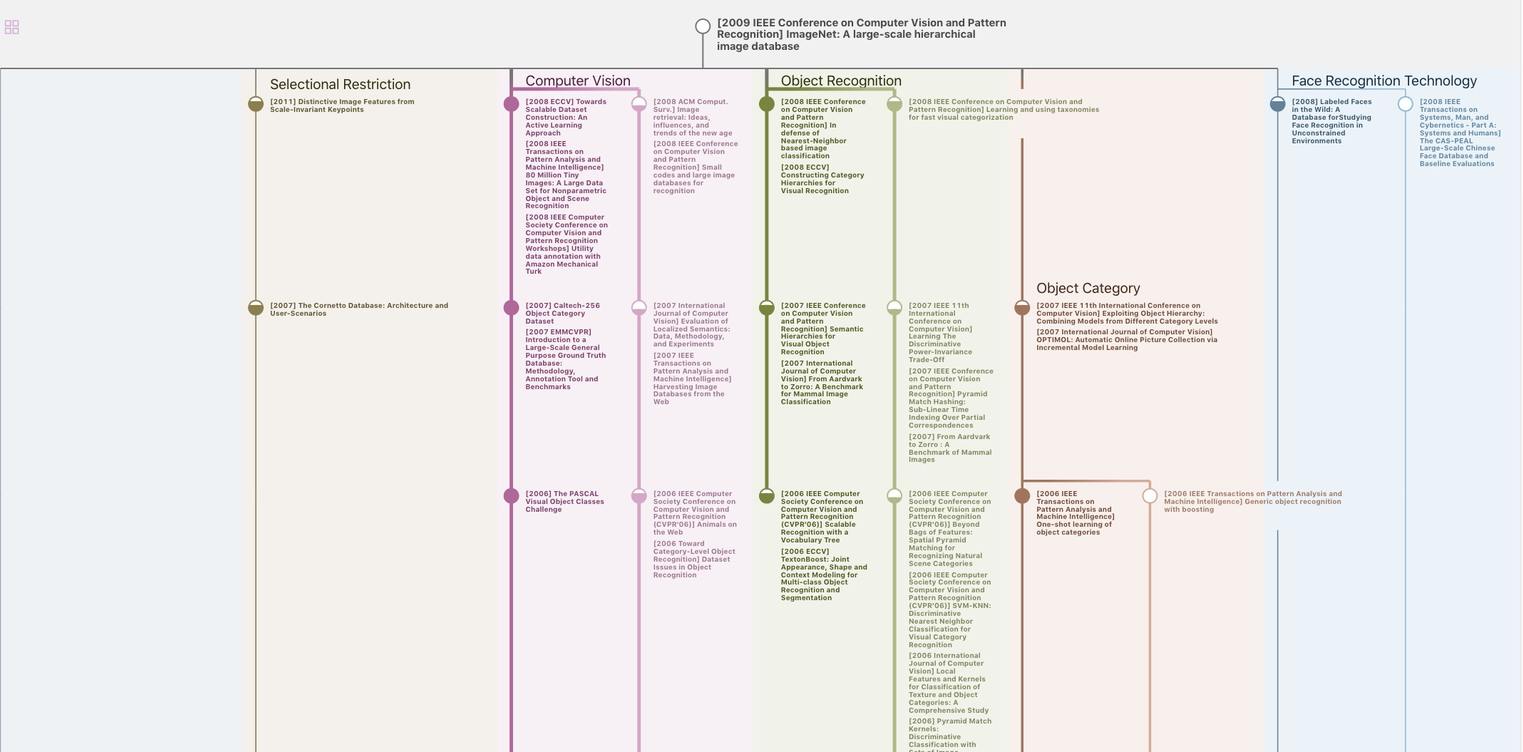
生成溯源树,研究论文发展脉络
Chat Paper
正在生成论文摘要