Distributed Inference Over Linear Models Using Alternating Gaussian Belief Propagation
IEEE INTERNET OF THINGS JOURNAL(2023)
摘要
We consider the problem of maximum-likelihood estimation in linear models represented by factor graphs and solved via the Gaussian belief propagation algorithm. Motivated by massive Internet of Things (IoT) networks and edge computing, we set the above problem in a clustered scenario, where the factor graph is divided into clusters and assigned for processing in a distributed fashion across a number of edge computing nodes. For these scenarios, we show that an alternating Gaussian belief propagation (AGBP) algorithm that alternates between inter- and intracluster iterations, demonstrates superior performance in terms of convergence properties compared to the existing solutions in the literature. We present a comprehensive framework and introduce appropriate metrics to analyze the AGBP algorithm across a wide range of linear models characterized by symmetric and nonsymmetric, square, and rectangular matrices. We extend the analysis to the case of dynamic linear models by introducing the dynamic arrival of new data over time. Using a combination of analytical and extensive numerical results, we show the efficiency and scalability of the AGBP algorithm, making it a suitable solution for large-scale inference in massive IoT networks.
更多查看译文
关键词
Heuristic algorithms,Inference algorithms,Maximum likelihood estimation,Internet of Things,Estimation,Signal processing algorithms,Computational modeling,Distributed systems,factor graphs,Gaussian belief propagation (GBP),Internet of Things (IoT) networks,linear models
AI 理解论文
溯源树
样例
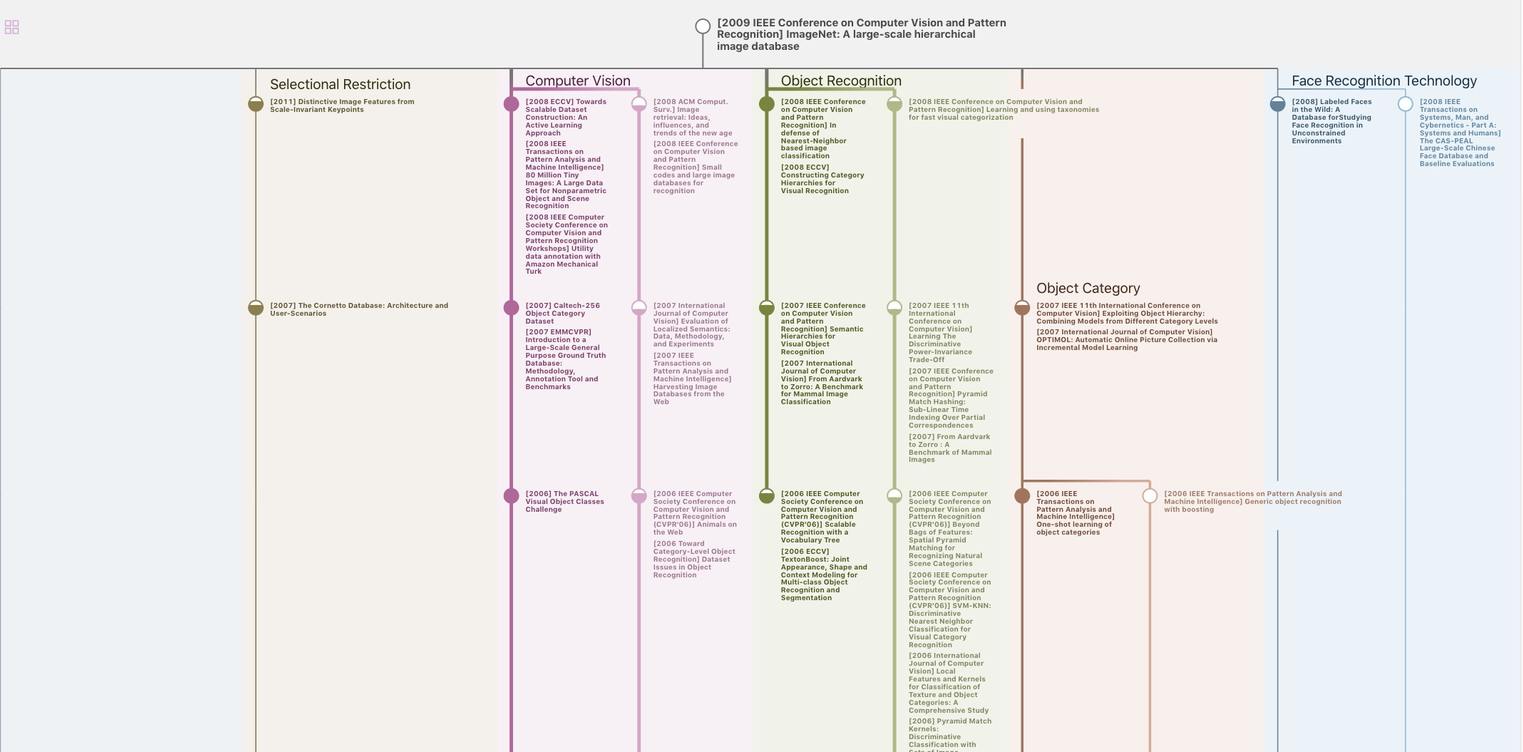
生成溯源树,研究论文发展脉络
Chat Paper
正在生成论文摘要