Biomedical Named Entity Recognition Using Transformers with biLSTM + CRF and Graph Convolutional Neural Networks
2022 International Conference on INnovations in Intelligent SysTems and Applications (INISTA)(2022)
摘要
One of the applications of Natural Language Processing (NLP) is to process free text data for extracting information. Information extraction has various forms like Named Entity Recognition (NER) for detecting the named entities in the free text. Biomedical named-entity extraction task is about extracting named entities like drugs, diseases, organs, etc. from texts in medical domain. In our study, we improve commonly used models in this domain, such as biLSTM+CRF model, using transformer based language models like BERT and its domain-specific variant BioBERT in the embedding layer. We conduct several experiments on several different benchmark biomedical datasets using a variety of combination of models and embeddings such as BioBERT+biLSTM+CRF, BERT+biLSTM+CRF, Fasttext+biLSTM+CRF, and Graph Convolutional Networks. Our results show a quite visible, 4% to 13%, improvements when baseline biLSTM+CRF model is initialized with pretrained language models such as BERT and especially with domain specific one like BioBERT on several datasets.
更多查看译文
关键词
Natural Language Processing,Named Entity Recognition,Biomedical,LSTM,CRF,GCN
AI 理解论文
溯源树
样例
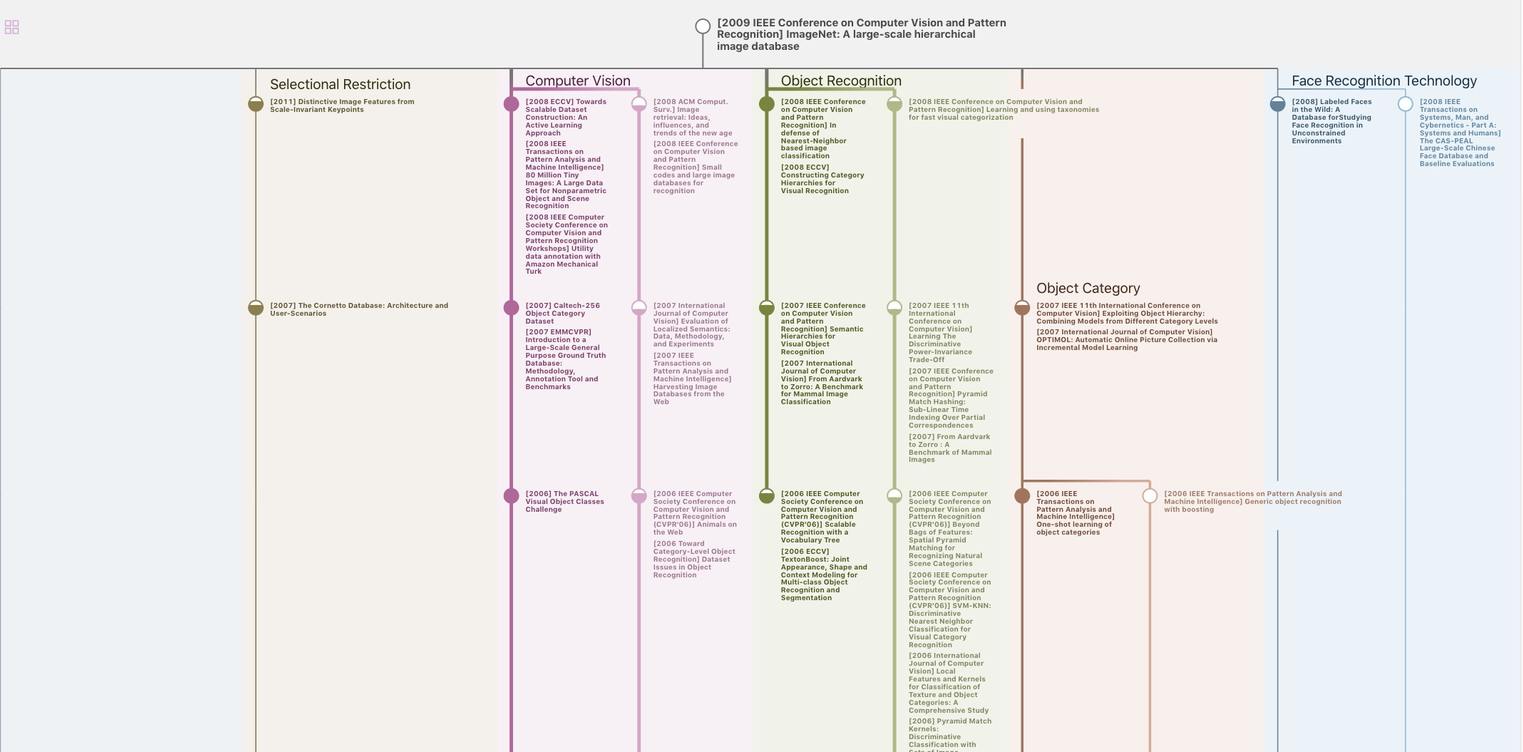
生成溯源树,研究论文发展脉络
Chat Paper
正在生成论文摘要