A Multistep Frank-Wolfe Method
arxiv(2022)
摘要
The Frank-Wolfe algorithm has regained much interest in its use in structurally constrained machine learning applications. However, one major limitation of the Frank-Wolfe algorithm is the slow local convergence property due to the zig-zagging behavior. We observe the zig-zagging phenomenon in the Frank-Wolfe method as an artifact of discretization, and propose multistep Frank-Wolfe variants where the truncation errors decay as $O(\Delta^p)$, where $p$ is the method's order. This strategy "stabilizes" the method, and allows tools like line search and momentum to have more benefits. However, our results suggest that the worst case convergence rate of Runge-Kutta-type discretization schemes cannot improve upon that of the vanilla Frank-Wolfe method for a rate depending on $k$. Still, we believe that this analysis adds to the growing knowledge of flow analysis for optimization methods, and is a cautionary tale on the ultimate usefulness of multistep methods.
更多查看译文
关键词
method,frank-wolfe
AI 理解论文
溯源树
样例
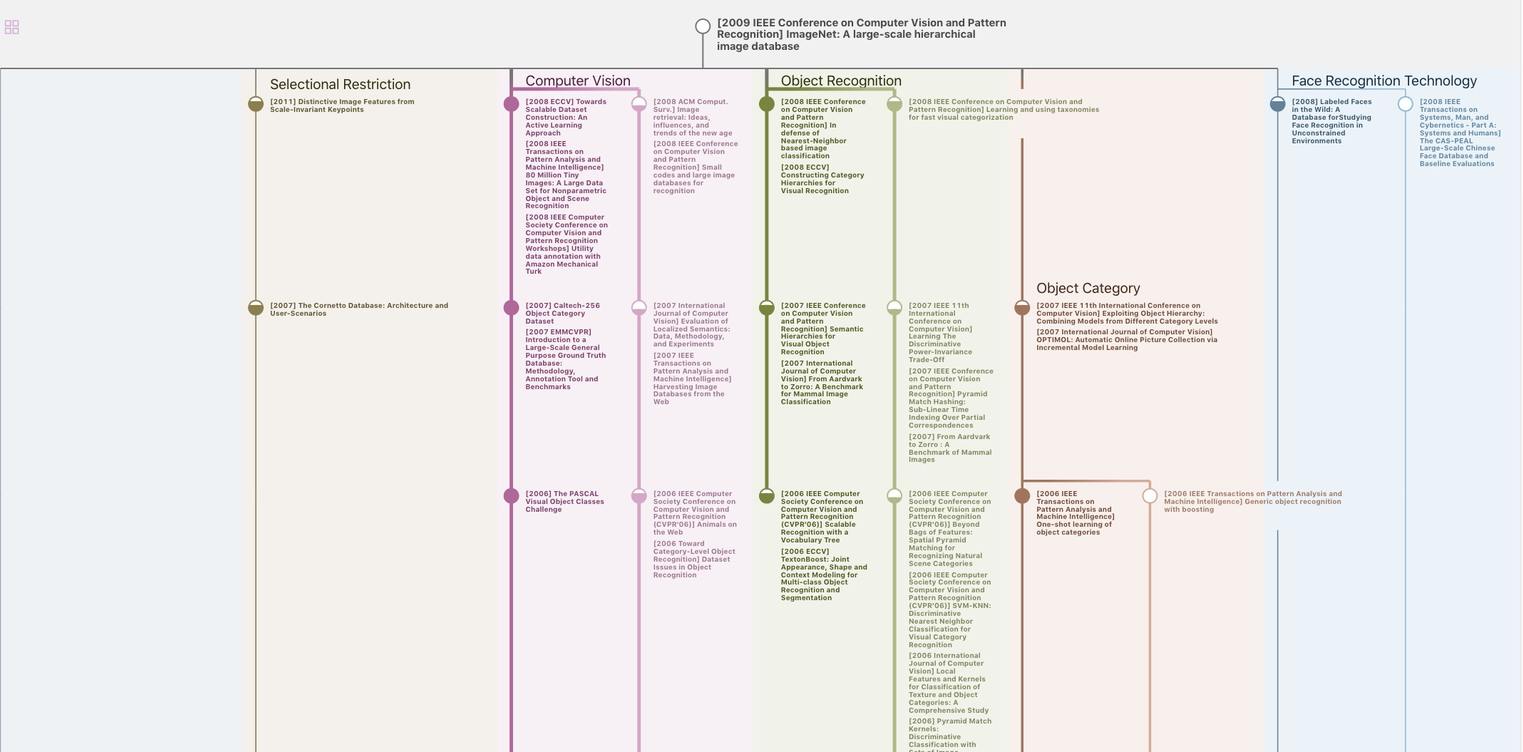
生成溯源树,研究论文发展脉络
Chat Paper
正在生成论文摘要