Boosting Reinforcement Learning of Robotic Assembly Tasks by Constraining the Actionspace in a Task-Specific Manner
ISR Europe 2022; 54th International Symposium on Robotics(2022)
摘要
Autonomous learning of robotic assembly tasks is a promising approach for the future of industrial manufacturing. The Reinforcement Learning (RL) framework provides a possibility to autonomous learning based on interaction with the environment but although much research has been done, poor trial efficiency is a problem for learning-based methods. Learning robust strategies requires many costly interactions with the environment, which severely limits the potential applications in an industrial context. We propose a grey-box learning approach that allows process experts to provide a partial behavioral description based on the Task Frame Formalism. The potential to speed up the learning progress by restricting the action space in a task-specific manner is demonstrated. We evaluate how much trial efficiency is increased by comparing different variations of constraints in a simulated Peg-in-Hole task. Moreover, we show that our method enables learning how to skillfully assemble a light bulb under positional uncertainties with comparatively few real-world trials. This shows the potential to automate industrial assembly processes efficiently.
更多查看译文
AI 理解论文
溯源树
样例
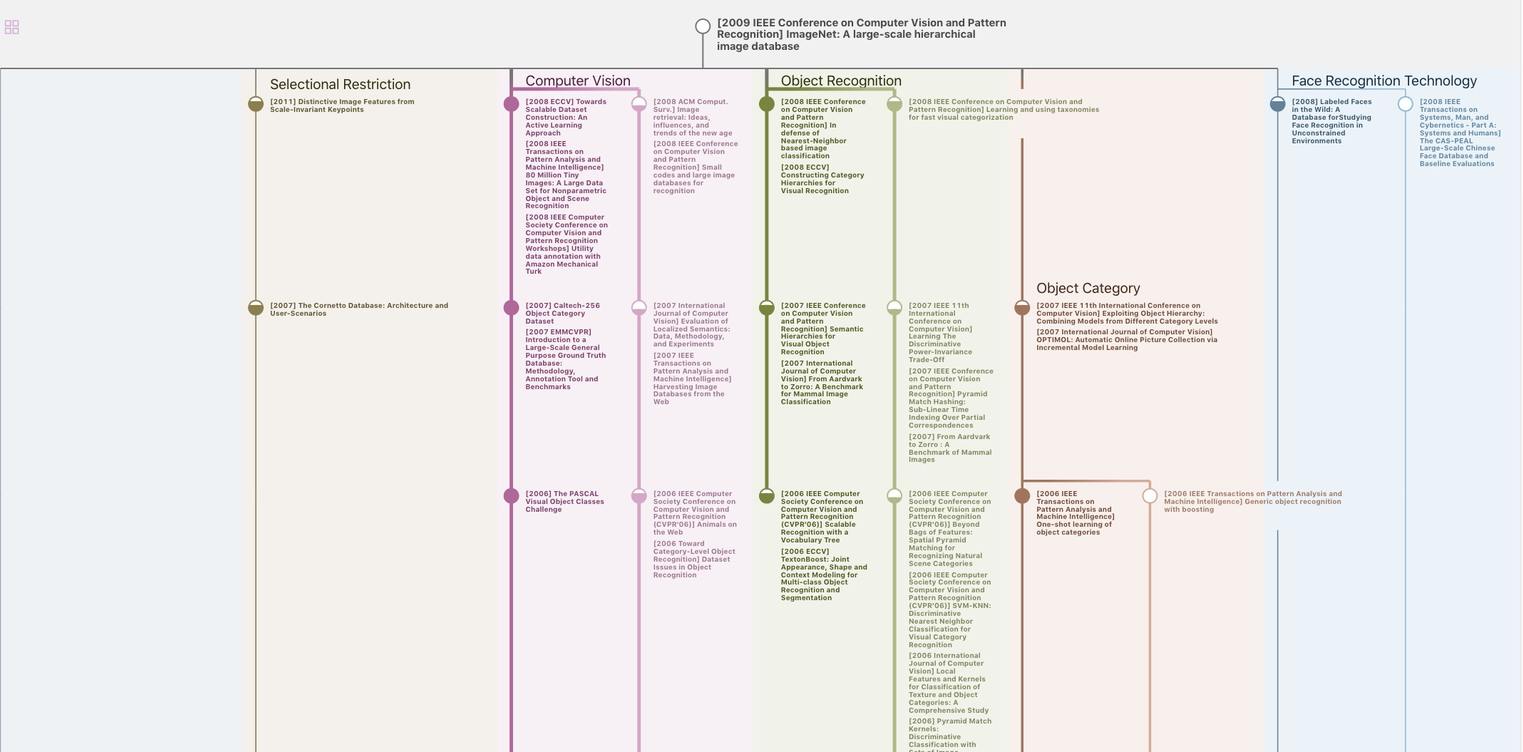
生成溯源树,研究论文发展脉络
Chat Paper
正在生成论文摘要