Improving Spoken Language Understanding with Cross-Modal Contrastive Learning
Conference of the International Speech Communication Association (INTERSPEECH)(2022)
摘要
Spoken language understanding(SLU) is conventionally based on pipeline architecture with error propagation issues. To mitigate this problem, end-to-end(E2E) models are proposed to directly map speech input to desired semantic outputs. Meanwhile, others try to leverage linguistic information in addition to acoustic information by adopting a multi-modal architecture. In this work, we propose a novel multi-modal SLU method, named CMCL, which utilizes cross-modal contrastive learning to learn better multi-modal representation. In particular, a two-stream multi-modal framework is designed, and a contrastive learning task is performed across speech and text representations. Moreover, CMCL employs a multi-modal shared classification task combined with a contrastive learning task to guide the learned representation to improve the performance on the intent classification task. We also investigate the efficacy of employing cross-modal contrastive learning during pretraining. CMCL achieves 99.69% and 92.50% accuracy on FSC and Smartlights datasets, respectively, outperforming state-of-the-art comparative methods. Also, performances only decrease by 0.32% and 2.8%, respectively, when trained on 10% and 1% of the FSC dataset, indicating its advantage under few-shot scenarios.
更多查看译文
关键词
spoken language understanding, intent classification, multi-modal, contrastive learning, pretraining
AI 理解论文
溯源树
样例
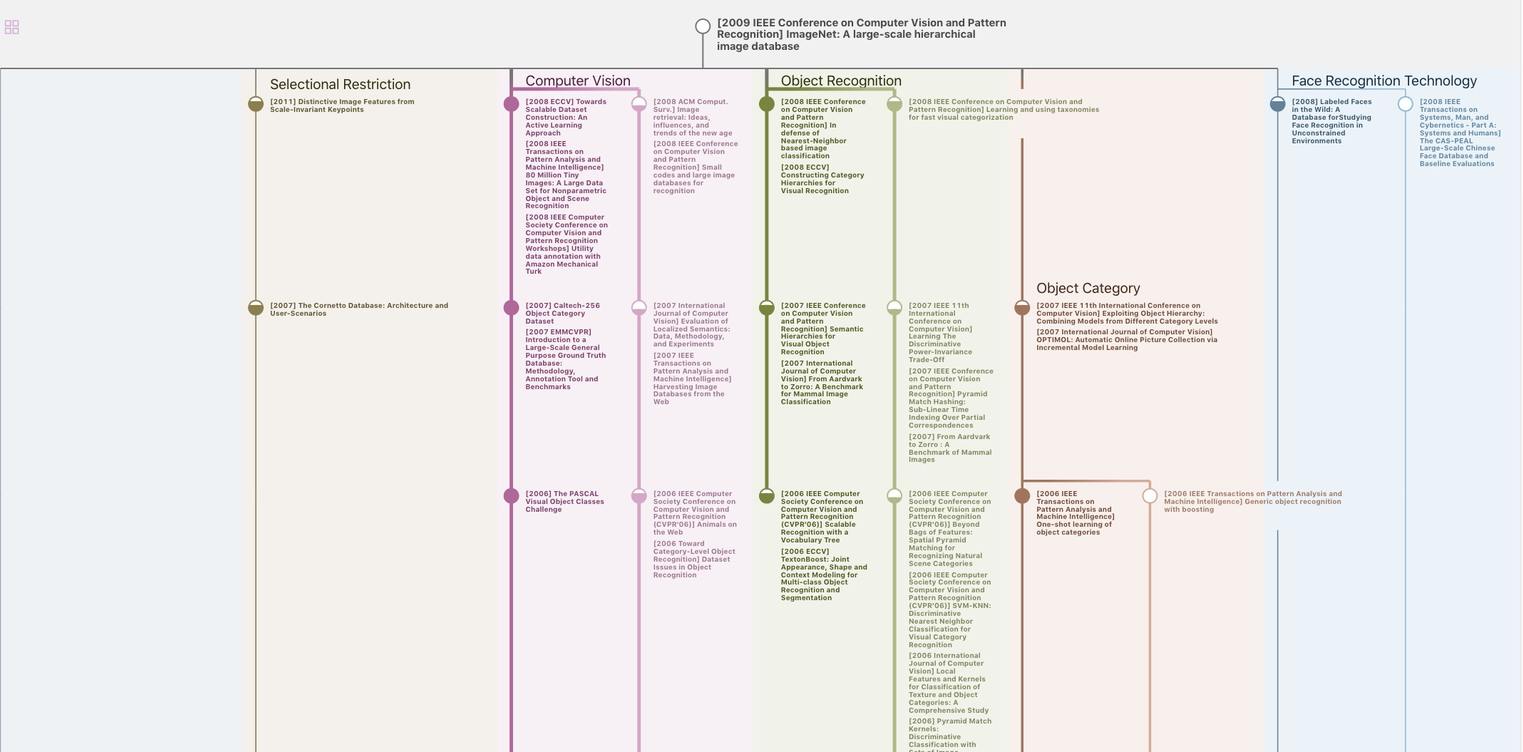
生成溯源树,研究论文发展脉络
Chat Paper
正在生成论文摘要