Data Augmentation for End-to-end Silent Speech Recognition for Laryngectomees
Conference of the International Speech Communication Association (INTERSPEECH)(2022)
摘要
Silent speech recognition (SSR) predicts textual information from silent articulation, which is an algorithm design in silent speech interfaces (SSIs). SSIs have the potential of recovering the speech ability of individuals who lost their voice but can still articulate (e.g., laryngectomees). Due to the logistic difficulties in articulatory data collection, current SSR studies suffer limited amount of dataset. Data augmentation aims to increase the training data amount by introducing variations into the existing dataset, but has rarely been investigated in SSR for laryngectomees. In this study, we investigated the effectiveness of multiple data augmentation approaches for SSR including consecutive and intermittent time masking, articulatory dimension masking, sinusoidal noise injection and randomly scaling. Different experimental setups including speaker-dependent, speaker-independent, and speaker-adaptive were used. The SSR models were end-to-end speech recognition models trained with connectionist temporal classification (CTC). Electromagnetic articulography (EMA) datasets collected from multiple healthy speakers and laryngectomees were used. The experimental results have demonstrated that the data augmentation approaches explored performed differently, but generally improved SSR performance. Especially, the consecutive time masking has brought significant improvement on SSR for both healthy speakers and laryngectomees.
更多查看译文
关键词
silent speech recognition, silent speech interface, data augmentation, alaryngeal speech
AI 理解论文
溯源树
样例
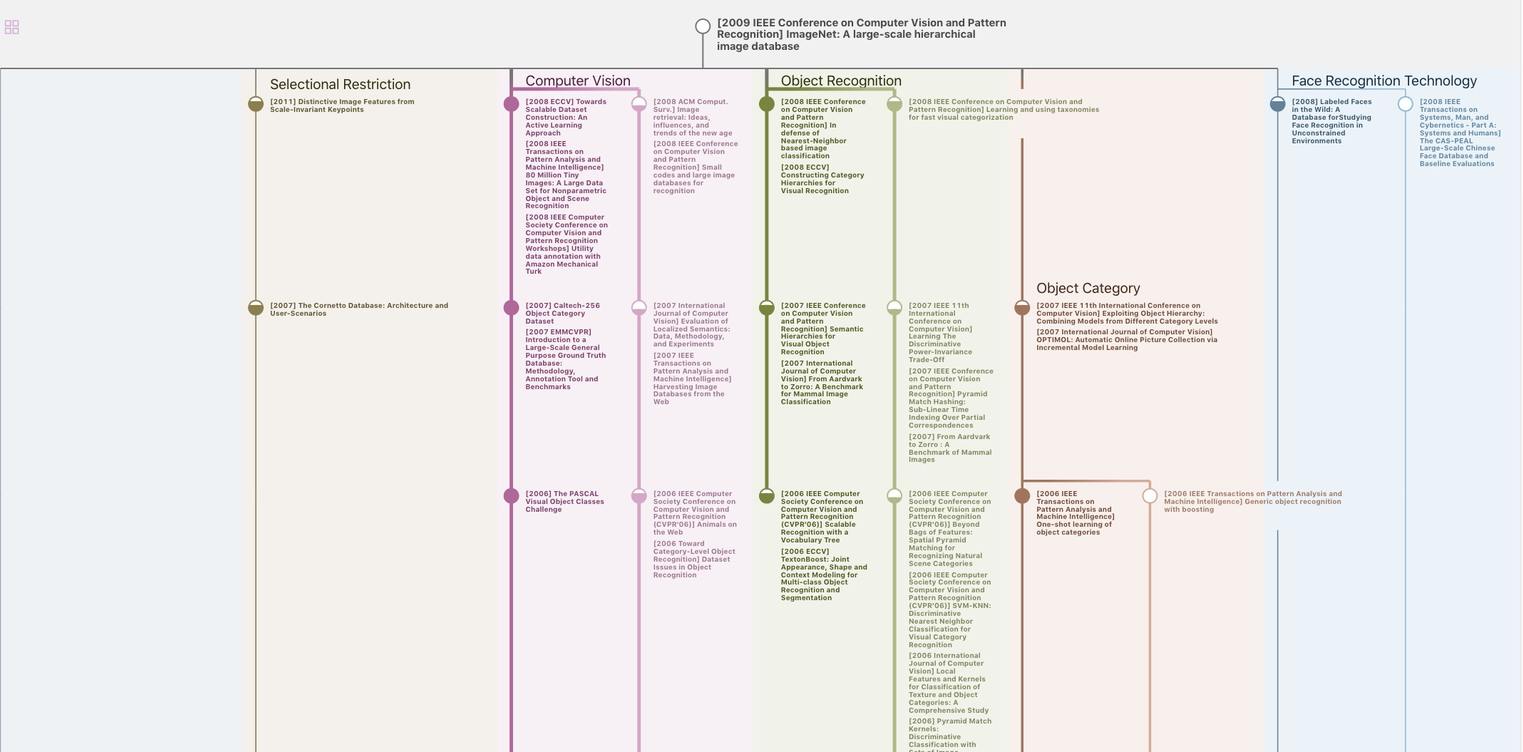
生成溯源树,研究论文发展脉络
Chat Paper
正在生成论文摘要