Simulate Human Thinking: Cognitive Knowledge Graph Reasoning for Complex Question Answering
ADVANCES IN KNOWLEDGE DISCOVERY AND DATA MINING, PAKDD 2022, PT I(2022)
摘要
Question answering over knowledge graph has attracted increasing attention. Though the previous algorithms have achieved competitive performance, they fail to solve problems like humans resulting in the bottleneck of reasoning. However, it is difficult for machines to simulate the question answering process of humans. In order to address this challenge, we propose a novel Cognitive Knowledge Graph Reasoning (CKGR) model based on the cognitive architecture for complex question answering. The CKGR processes information hierarchically with a three-level framework. To fully analyze the question, the first level is proposed to transform the question into features according to different aspects. Then, the relative knowledge graph (KG) regions are activated to simulate the human unconscious thinking process by a memory mapping module. Finally, the CKGR goes deeper to infer the correct answer over KG considering the both semantic and logical parsing of the questions. The CKGR successfully narrows the gap between humans and machines. Extensive experiments on three real-world datasets demonstrate that the proposed method achieves better performance compared with the state-of-the-art methods and provides the reasoning score to find the reasonable path for the answer.
更多查看译文
关键词
Question answering, Cognitive knowledge graph, Knowledge reasoning
AI 理解论文
溯源树
样例
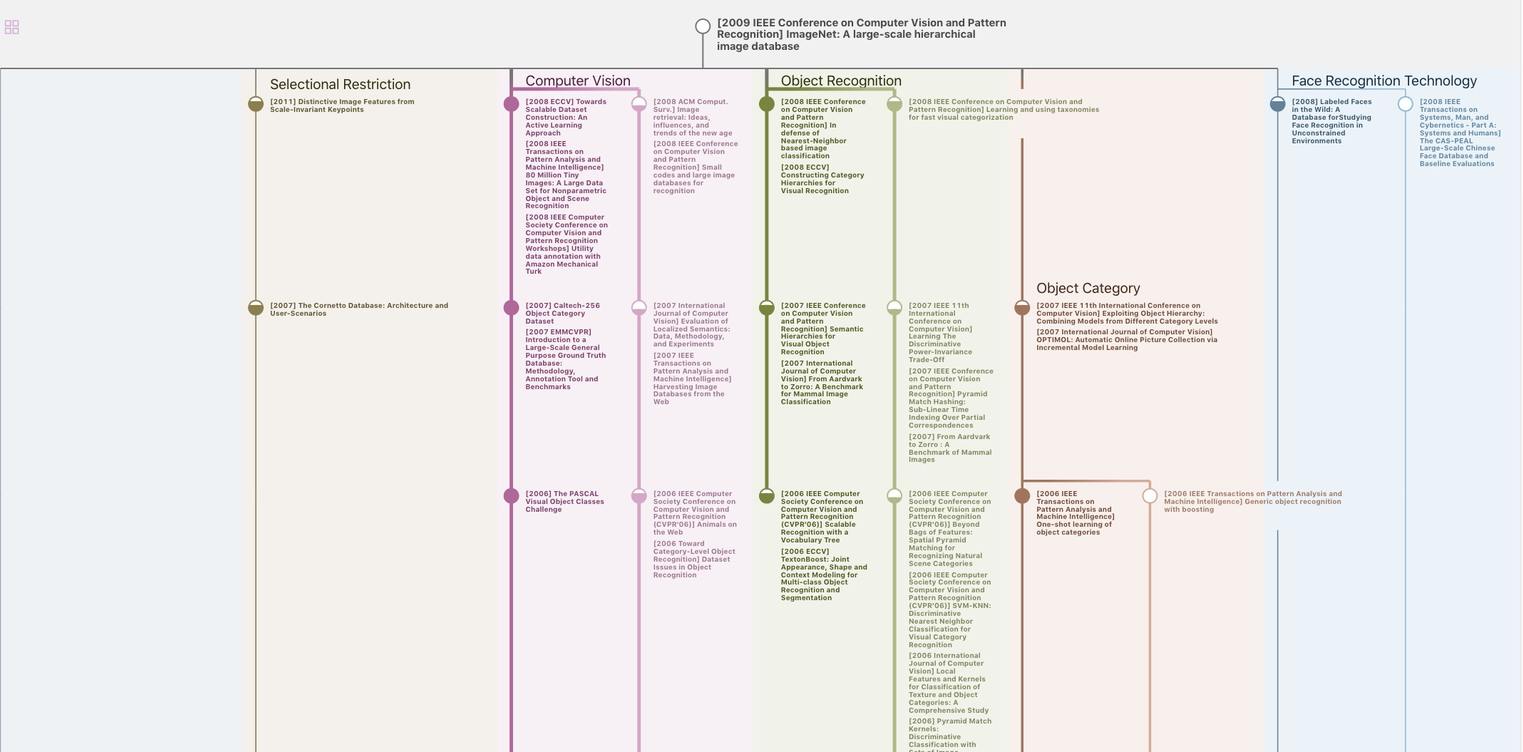
生成溯源树,研究论文发展脉络
Chat Paper
正在生成论文摘要