Separate then Constrain: A Hierarchical Network for End-to-End Triples Extraction
ADVANCES IN KNOWLEDGE DISCOVERY AND DATA MINING, PAKDD 2022, PT I(2022)
摘要
In recent years, end-to-end triples extraction based on multi-task learning has achieved promising performance. The existing methods typically use the same sentence representation generated by pretrained language models to address different subtasks. They are either hard to capture the subtask-specific features, or hard to make deep associations among different subtasks. In this paper, we propose a Separate then Constrain Network (SCN) that contains two main layers, i.e., separation layer and constraint layer. Specifically, separation layer first transfers the sentence representation into three different subtask spaces, respectively. Then, constraint layer further refines all sentence representations by simulating the inherent dependencies among three parts of a triple. In addition, to alleviate the negative impact of the error entity prediction on relation classification, we design a simple but effective way, called Entity-Derivate Checker. On three public datasets, SCN shows significant improvement over existing methods.
更多查看译文
关键词
Triples extraction, Joint model, Hierarchical network
AI 理解论文
溯源树
样例
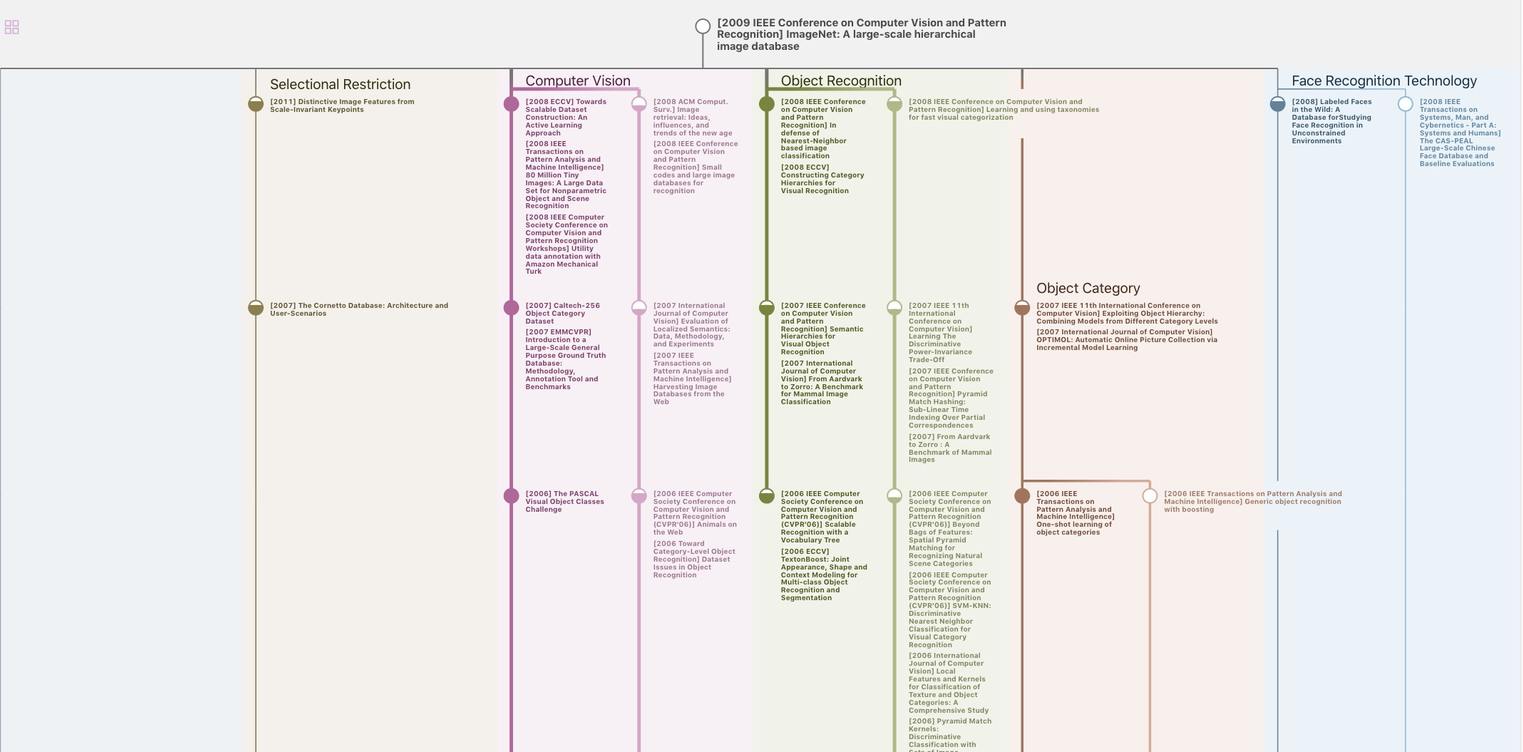
生成溯源树,研究论文发展脉络
Chat Paper
正在生成论文摘要