A Hybrid Semantic-Topic Co-encoding Network for Social Emotion Classification
ADVANCES IN KNOWLEDGE DISCOVERY AND DATA MINING, PAKDD 2022, PT I(2022)
摘要
Social emotion classification is to predict the distribution of readers' emotions evoked by a document (e.g., news article). Previous work has shown that both semantic and topical information can help improve classification performance. However, many existing topic-based neural models represent the topical feature of document with only topic probabilities, ignoring the fine-grained semantic feature of terms in each topic. Moreover, traditional RNN-based semantic networks often face the disadvantage of slow training In this paper, we propose a hybrid semantic-topic co-encoding network. It contains a semantics-driven topic encoder to compose topic embeddings, and also utilizes a forward self-attention network to exploit document semantics. Finally, the semantic and topical features of the document are adaptively integrated through a gate layer, which generates the document representation for social emotion classification. Experimental results on three public datasets show that the proposed model outperforms the state-of-the-art approaches in terms of higher accuracy and average Pearson correlation coefficient. Moreover, the proposed model runs fast and with better explainability.
更多查看译文
关键词
Sentiment analysis, Social emotion classification, Topic model, Self-attention
AI 理解论文
溯源树
样例
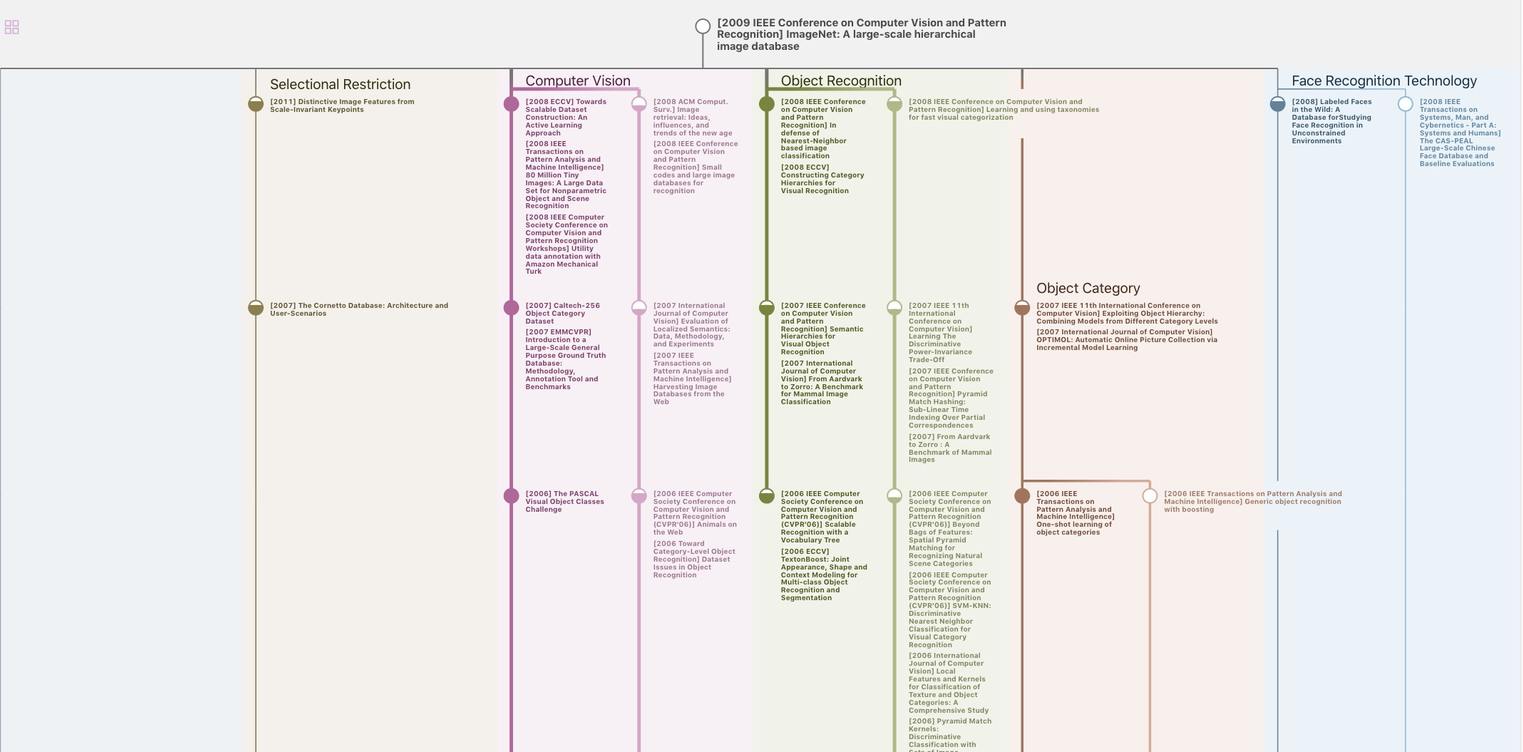
生成溯源树,研究论文发展脉络
Chat Paper
正在生成论文摘要