Bridging Formal Methods and Machine Learning with Global Optimisation
IEEE International Conference on Formal Engineering Methods (ICFEM)(2022)
摘要
Formal methods and machine learning are two research fields with drastically different foundations and philosophies. Formal methods utilise mathematically rigorous techniques for the specification, development and verification of software and hardware systems. Machine learning focuses on pragmatic approaches to gradually improve a parameterised model by observing a training data set. While historically the two fields lack communication, this trend has changed in the past few years with an outburst of research interest in the robustness verification of neural networks. This paper will briefly review these works, and focus on the urgent need for broader, and more in-depth, communication between the two fields, with the ultimate goal of developing learning-enabled systems with not only excellent performance but also acceptable safety and security. We present a specification language, MLS, and show that it can express a set of known safety and security properties, including generalisation, uncertainty, robustness, data poisoning, backdoor, model stealing, membership inference, model inversion, interpretability, and fairness. To verify MLS properties, we promote the global optimisation based methods, which have provable guarantees on the convergence to the optimal solution. Many of them have theoretical bounds on the gap between current solutions and the optimal solution.
更多查看译文
关键词
formal methods,global optimisation,machine learning
AI 理解论文
溯源树
样例
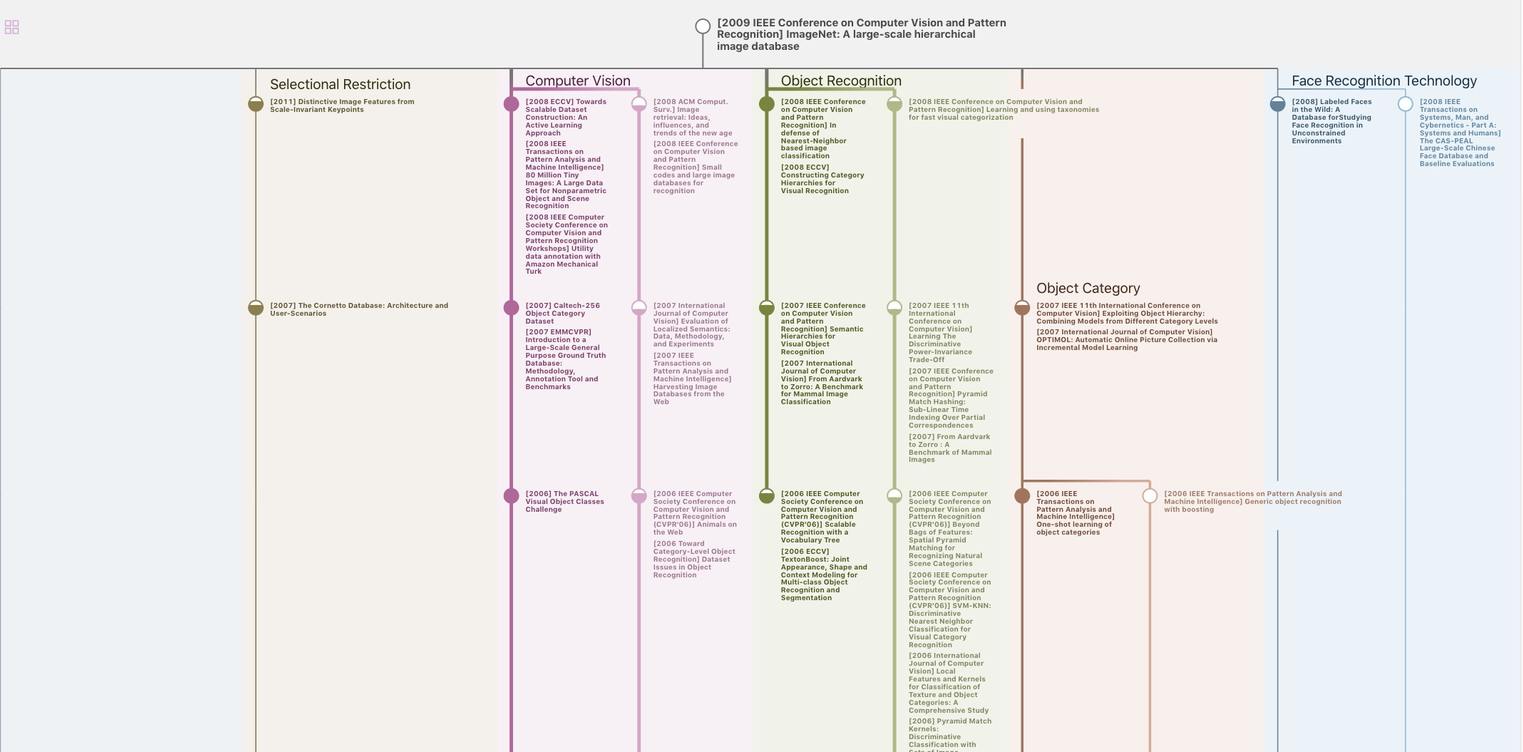
生成溯源树,研究论文发展脉络
Chat Paper
正在生成论文摘要