Query-Efficient Black-Box Adversarial Attack with Random Pattern Noises
International Conference on Information and Communication Security (ICICS)(2022)
摘要
Adversarial examples are one of the largest vulnerability of deep neural networks. An attacker can deceive the classifiers easily with the malicious inputs (called adversarial examples), which perturbations are slightly added to benign inputs. Various attack methods have been studied in both white-box and black-box settings, and some methods achieve high attack success rates even in the black-box settings; that is, the attacker is restricted to only query accesses to the target network. In this paper, we propose a simple hyperparameter-free score-based black-box l(infinity)-adversarial attack using local uniform noises and a random search. Specifically, we construct adversarial perturbations by combining local uniform noises such as vertical-wise and horizontal-wise, and incorporate this idea into the random search method to update the perturbation sequentially. We evaluate our method in terms of attack success rates and query efficiency using models that classify common datasets CIFAR-10 and ImageNet. We show that our method achieves higher attack success rates and query efficiency than previous attack methods, especially in low-query budgets on both untargeted and targeted attack settings. We also examine attacks to adversarially trained models and discuss the effect of local uniform noises on these models. Furthermore, we show that our method achieves relatively high attack success rates and query efficiency on average against input-transformation-based defense methods, and is virtually unaffected by these defense methods.
更多查看译文
关键词
Black-box adversarial attacks,AI security
AI 理解论文
溯源树
样例
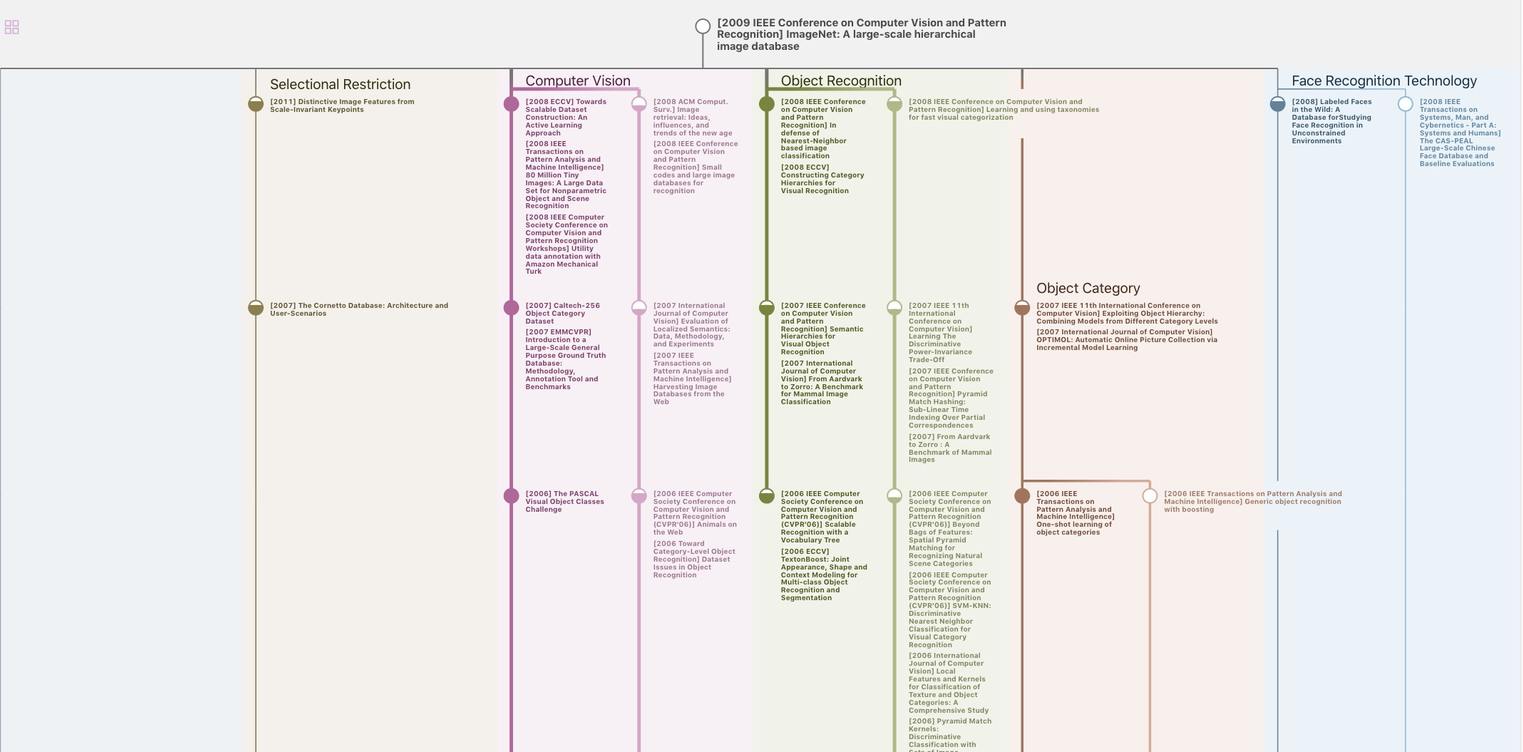
生成溯源树,研究论文发展脉络
Chat Paper
正在生成论文摘要