A Cloud Native SLA-Driven Stochastic Federated Learning Policy for 6G Zero-Touch Network Slicing
IEEE International Conference on Communications (ICC)(2022)
摘要
Sixth-generation (6G)-enabled massive network slicing is a strong enabler for the expected pervasive digitalization of the vertical market. In such a context, artificial intelligence (AI)-driven zero-touch network automation should present a high degree of scalability and sustainability, especially when deployed in live production networks wherein the collected monitoring datasets at different points are non-independent and identically distributed (non-IID). This paper presents a new cloud-native service-level agreement (SLA)-driven stochastic policy to guarantee a scalable and fast operation of constrained federated learning (FL)-based analytic engines that perform statistical slice-level resource provisioning at RAN-Edge in a non-IID setup. Both simulated and cloud-native emulated scenarios are implemented to demonstrate the superiority of the solution in reducing SLA violation, convergence time and computation cost compared to different FL baselines, showcasing thereby a higher scalability.
更多查看译文
关键词
6G,cloud-native,federated learning,game theory,proxy-Lagrangian,resource allocation,SLA,stochastic policy,ZSM
AI 理解论文
溯源树
样例
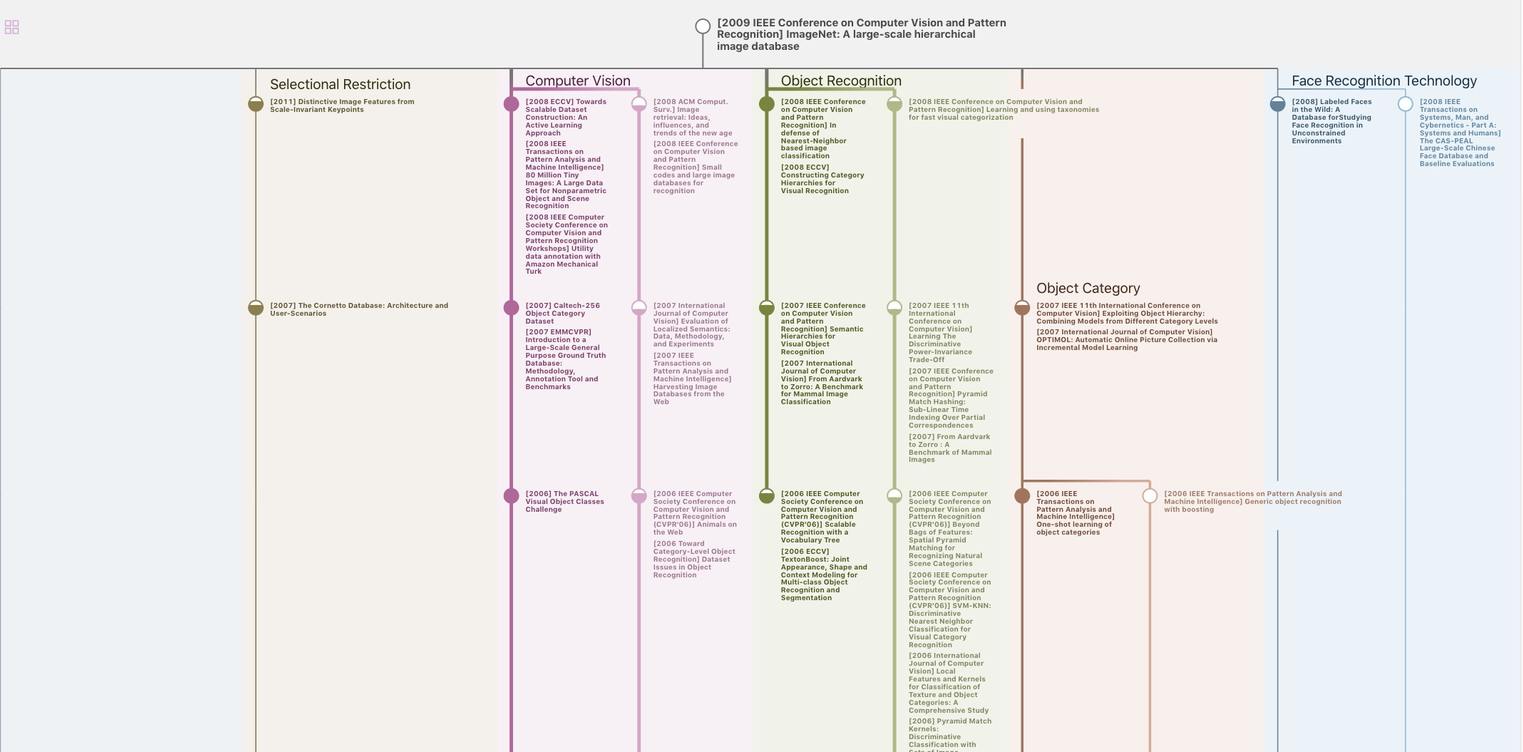
生成溯源树,研究论文发展脉络
Chat Paper
正在生成论文摘要