Worker Selection for On-Demand Crowdsourcing
2022 International Conference on Computer Communications and Networks (ICCCN)(2022)
摘要
The ubiquity of mobile devices allows mobile users to participate in crowdsourcing anywhere, anytime. One potential application is to crowdsource photos/videos on demand to search for interested targets. Crowdsourced photos/videos have much better coverage compared to surveillance cameras, and thus help improve the effectiveness of target search. However, broadcasting the crowdsourcing task to all mobile users can significantly increase the cost in terms of resource and incentive budget. To reduce cost, the crowdsourcing server selects a subset of participating workers, and there are many challenges on worker selection. For example, due to occlusions in the photo/video scene, each worker only covers part of the area with certain probability. Due to the non-deterministic nature of this problem, we study two kinds of optimization problems: max-coverage which maximizes the probability of finding the target given a cost, and min-selection which minimizes the number of workers given the required probability of finding the target. Considering that workers may report exact locations or coarse-grained locations, we formalize four probability-based optimization problems for worker selection, and develop optimal or efficient approximation algorithms to solve them. The effectiveness of the proposed algorithms is evaluated and validated via extensive trace-driven simulations and a real-world demo.
更多查看译文
关键词
target search,mobile users,incentive budget,crowdsourcing server,worker selection,min-selection,on-demand crowdsourcing,mobile devices,probability-based optimization,coarse-grained locations,trace-driven simulations,max-coverage
AI 理解论文
溯源树
样例
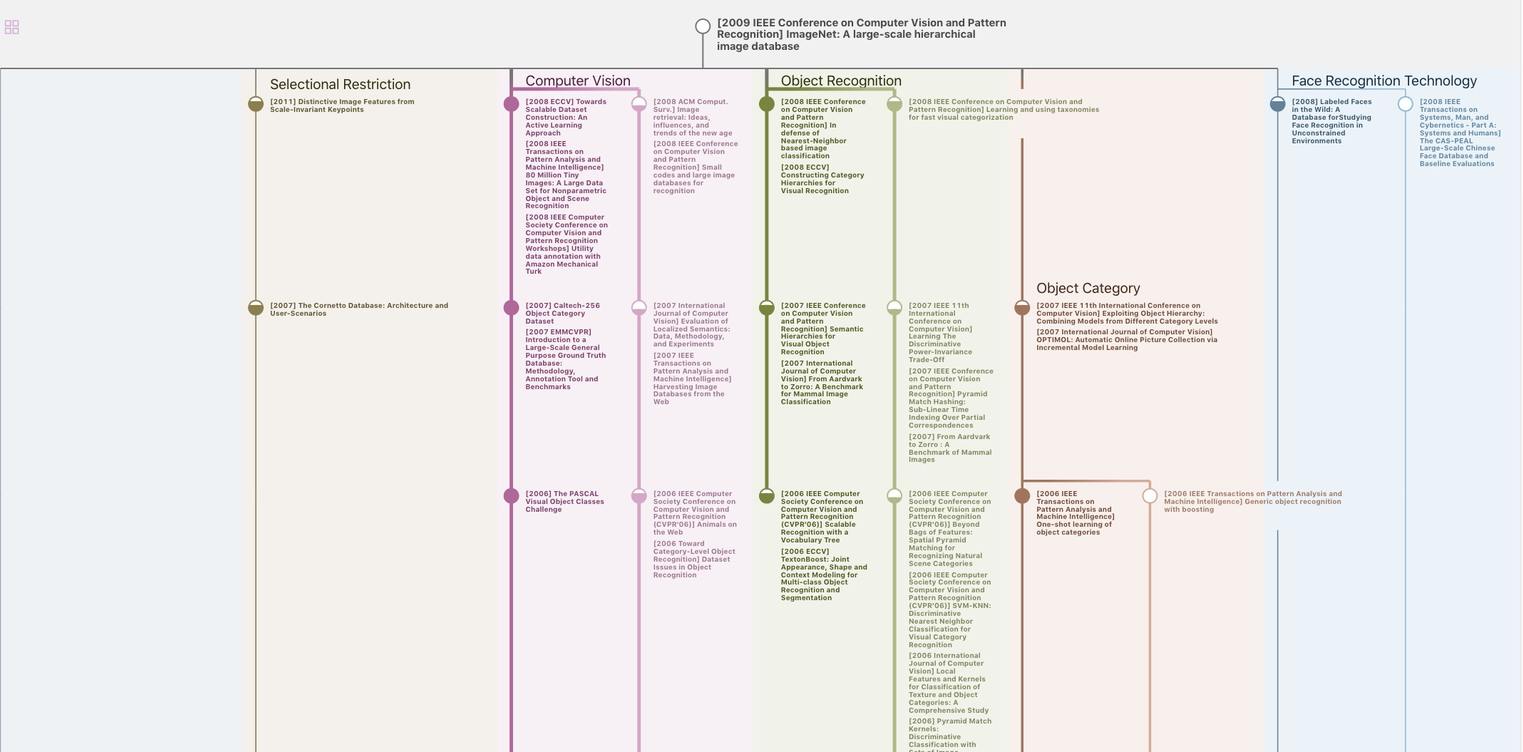
生成溯源树,研究论文发展脉络
Chat Paper
正在生成论文摘要