Learning to Mitigate Extreme Distribution Bias for Few-Shot Object Detection
IEEE International Conference on Multimedia and Expo (ICME)(2022)
摘要
Few-shot object detection is an important but challenging task where only a few instances of novel categories are available. The widely used approach is to pretrain a detector on base classes with abundant samples and then fine-tune it for novel classes. Due to the extreme data imbalance between base and novel classes, the detection performance of novel classes degrades with the distribution bias. To overcome this limitation, we propose a distribution calibration strategy and a class discrimination regularization method for better few-shot detection. Based on theoretical analysis on decision margins of base and novel classes, the decision area of novel classes is enlarged to balance the prediction probability. On the other hand, to increase the separability of inter-class distributions, the similarity between class-specific representations is minimized. Extensive experiments on PASCAL VOC and MS COCO datasets verify the effectiveness and generalization ability of our method to improve few-shot object detection.
更多查看译文
关键词
Few-shot Object Detection,Distribution Bias,Decision Margin
AI 理解论文
溯源树
样例
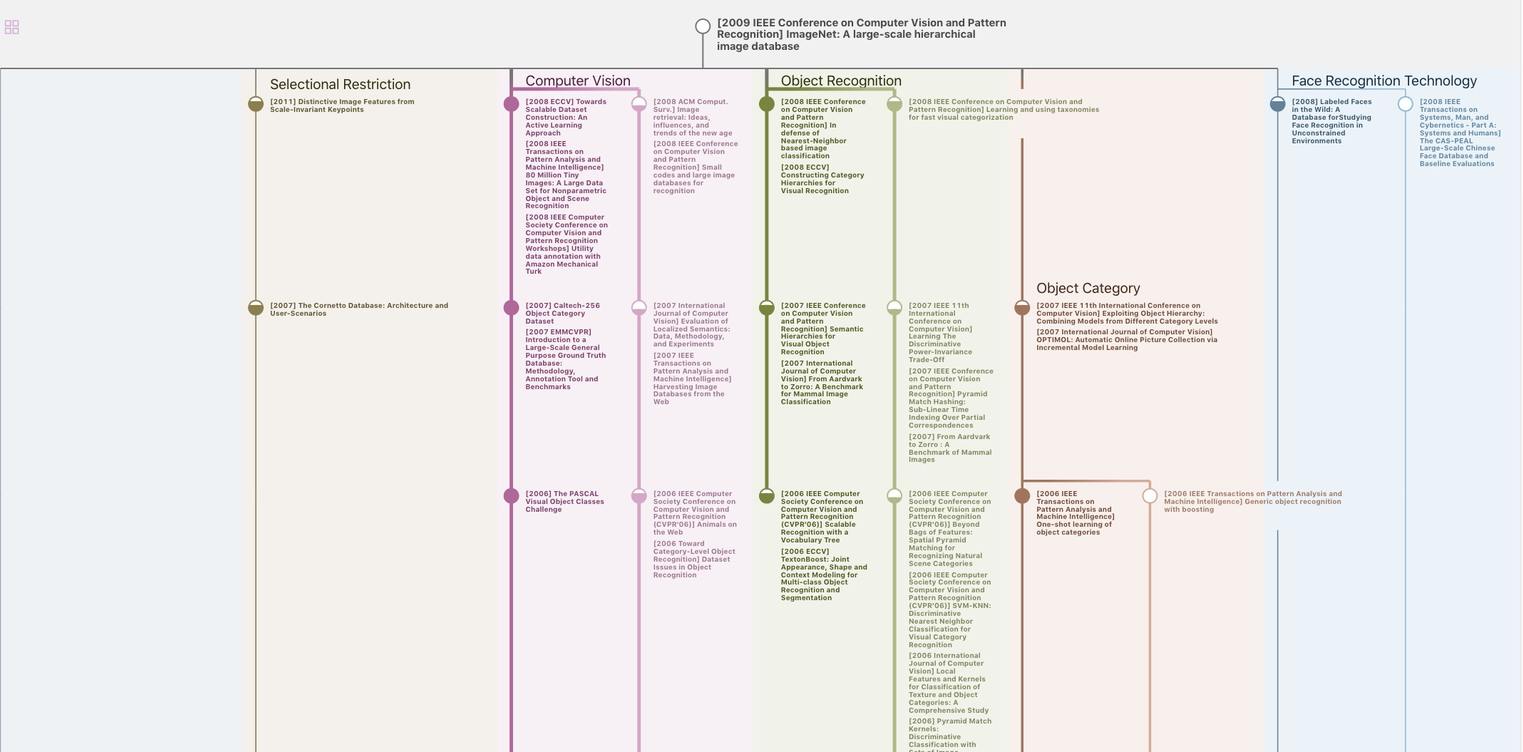
生成溯源树,研究论文发展脉络
Chat Paper
正在生成论文摘要