Learning Robust Latent Space of Basketball Player Trajectories for Tactics Analysis
IEEE International Conference on Multimedia and Expo (ICME)(2022)
摘要
Tactic analysis of trajectory data plays an important role in the field of sports science. However, the tactical labels of trajectories are usually insufficient, which limits the deep models to learn the general concept of tactics. In this work, we combine the recurrent variational autoencoder with attention module to learn a robust latent space of the basketball offensive trajectories without the need of labeled data. The learned latent space can be further applied to advanced tasks such as supervised tactics classification, unsupervised tactics clustering, and defensive trajectory generation. The experimental results show that our proposed model achieves better or competitive performance than the previous methods. Furthermore, our proposed model is the first one that focuses on zero-shot tactics clustering and the performance even outperforms the previous methods with supervised and unsupervised settings, which shows the promise of tactics analysis for trajectories in the case of limited labeled data.
更多查看译文
关键词
basketball,defensive trajectories generation,offensive tactic recognition
AI 理解论文
溯源树
样例
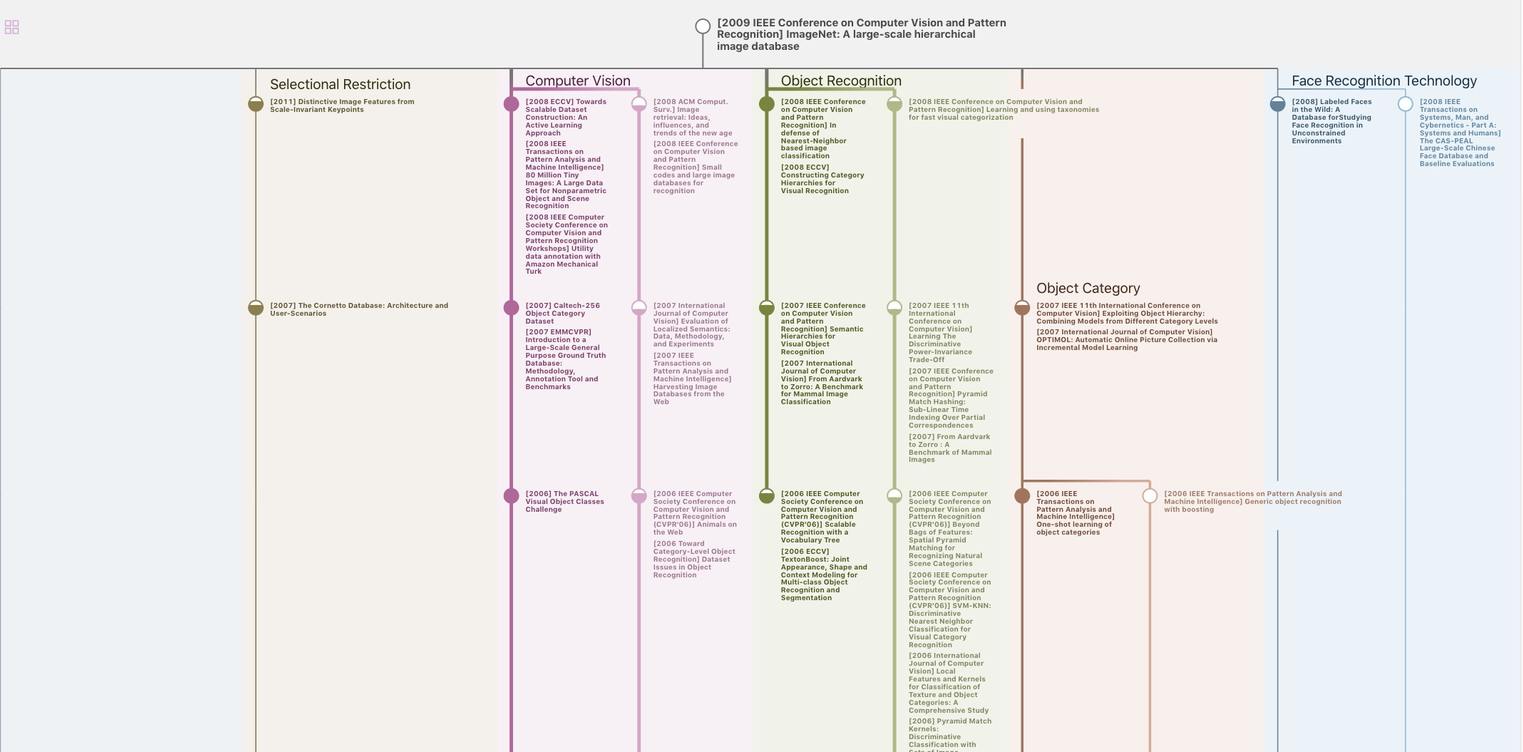
生成溯源树,研究论文发展脉络
Chat Paper
正在生成论文摘要