Interpretable Learned Image Compression: A Frequency Transform Decomposition Perspective
DCC 2022: 2022 DATA COMPRESSION CONFERENCE (DCC)(2022)
摘要
Image compression is a key problem in this age of information explosion. With the help of machine learning, recent studies have shown that learning-based image compression methods tend to surpass traditional codecs. Image compression can be split into three steps: transform, quantization, and entropy estimation. However, the transform step in traditional codecs lacks flexibility because of the strict mathematical premise while the transform in most learning-based codecs neglects its intrinsic interpretation. After observing compression degradation degree varies on different frequency bands as illustrated as Fig. 1(a), we propose an end-to-end compression model from the frequency perspective with a frequency-pyramid transform and a frequency-aware fusion module. The right of the Fig. 1(a) displays each frequency layer's component of the proposed model from low to high-frequency splits. Intuitively, we can infer that the low-frequency part contains the global structure while the high-frequency part gets finer details, satisfying the feature of human visual system (HVS). The proposed model are detailedly shown in Fig. 1(b) that independent probability estimation models are set for each frequency split. Extensive experiments are conducted to demonstrate that our model outperforms all traditional codecs (e.g., JPEG, JPEG2000, HEVC, and VVC) on MS-SSIM metric on both Kodak and CLIC2020 professional test datasets. Taking BPG-4:4:4 as the anchor, our proposed model achieves 11.6% BD-rate reduction under PSNR measurement, which is evaluated on the Kodak dataset.
更多查看译文
关键词
traditional codecs,machine learning,image compression methods,transform step,compression degradation degree,end-to-end compression model,frequency perspective,frequency pyramid,frequency layer,low frequency part,high frequency part,frequency split,high frequency splits,frequency aware fusion module,learning based codecs
AI 理解论文
溯源树
样例
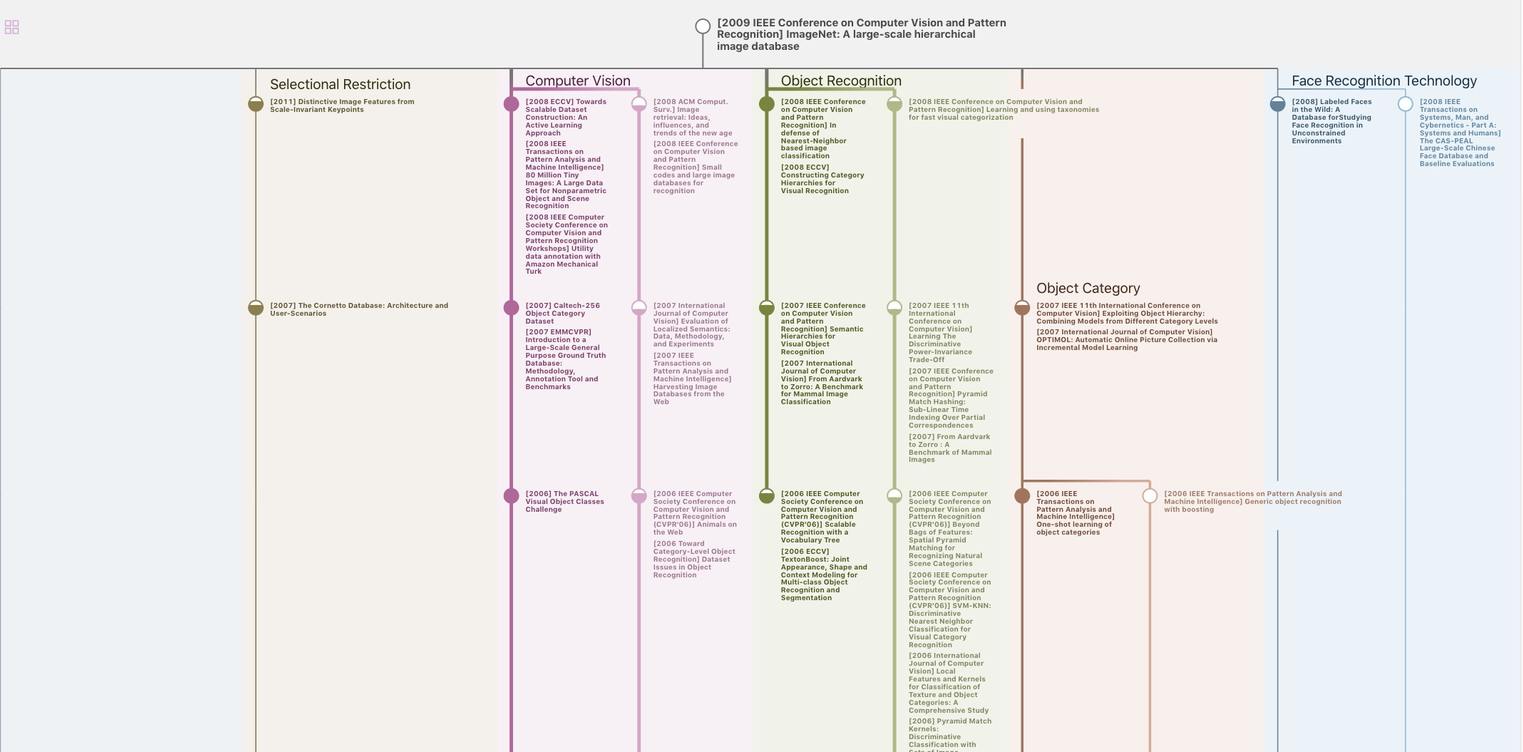
生成溯源树,研究论文发展脉络
Chat Paper
正在生成论文摘要