Material-Guided Siamese Fusion Network for Hyperspectral Object Tracking
IEEE International Conference on Acoustics, Speech, and Signal Processing (ICASSP)(2022)
摘要
Hyperspectral videos (HSVs) have more potential in target tracking than color videos thanks to the material identification capability provided by abundant spectral bands. Due to limited HSVs for training, most current hyperspectral trackers are based on hand-crafted features rather than deeply learned ones, resulting in poor tracking performance. This paper introduces a material-guided Siamese fusion network (SiamF) for hyperspectral object tracking to make up this gap. Belonging to the Siamese tracker family and SiamF aims to model the appearance of hyperspectral objects using backbone networks trained on color images. Specifically, SiamF splits each hyperspectral frame into multiple groups of false-color images according to their band importance. Then SiamF employs a hyperspectral feature fusion (HFF) module with a dense connection architecture to integrate the extracted features from different layers and band groups, producing a multi-scale multilevel spatial-spectral representation of the targets. Instead of direct addition or concatenation, HFF employs global-local channel attention for feature fusion, so that yielded features capture the global and local structure of a specific object. Moreover, online spatial and material classifiers are developed to inject spatial and material appearance changes information into SiamF for adaptively online tracking. Experimental results demonstrate our tracker outperforms alternative methods.
更多查看译文
关键词
Hyperspectral object tracking,feature fusion,material unmixing,appearance modelling
AI 理解论文
溯源树
样例
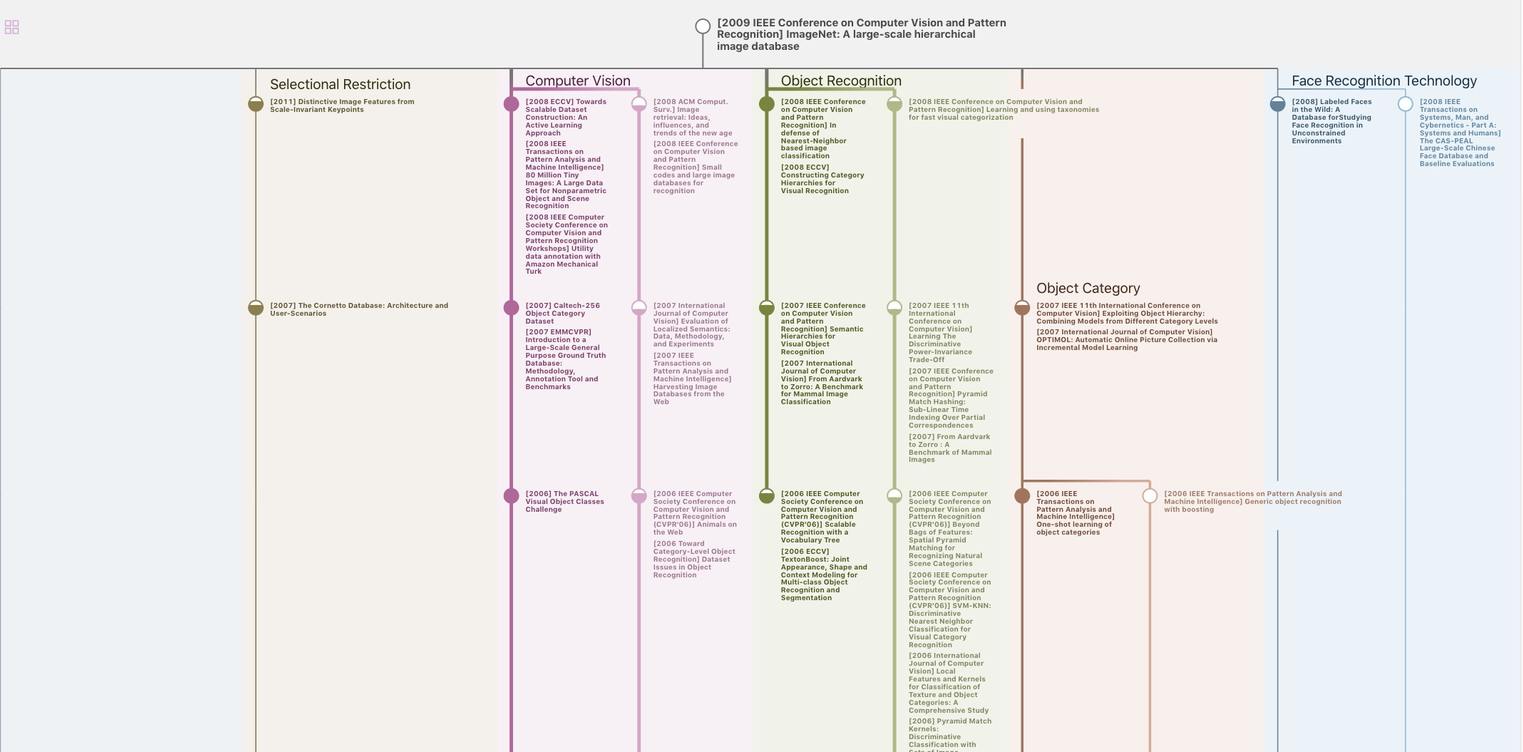
生成溯源树,研究论文发展脉络
Chat Paper
正在生成论文摘要