W-ART: Action Relation Transformer for Weakly-Supervised Temporal Action Localization
IEEE International Conference on Acoustics, Speech, and Signal Processing (ICASSP)(2022)
摘要
Weakly-supervised temporal action localization (WTAL) is a long-standing and challenging research problem in video signal analysis. It is to localize the action segments in the video given only video-level labels. The key to this task is understanding how the diverse actions interact. In this paper, we propose W-ART, a relation Transformer to explicitly capture the relationships between action segments. We devise a new effective Transformer architecture and construct new training loss functions for WTAL. Further, we propose a dedicated query mechanism to satisfy the different feature preferences between classification and localization. Thanks to these designs, our W-ART can accurately localize the diverse actions even in weakly-supervised setting. Extensive evaluation and empirical analysis show that our method outperforms the state of the arts on two challenging benchmarks, Charades and THUMOS14.
更多查看译文
关键词
Weakly-supervised Temporal Action Localization,Long-range Temporal Segment Dependency,Relationship Transformer,Weakly-supervised Query Mechanism
AI 理解论文
溯源树
样例
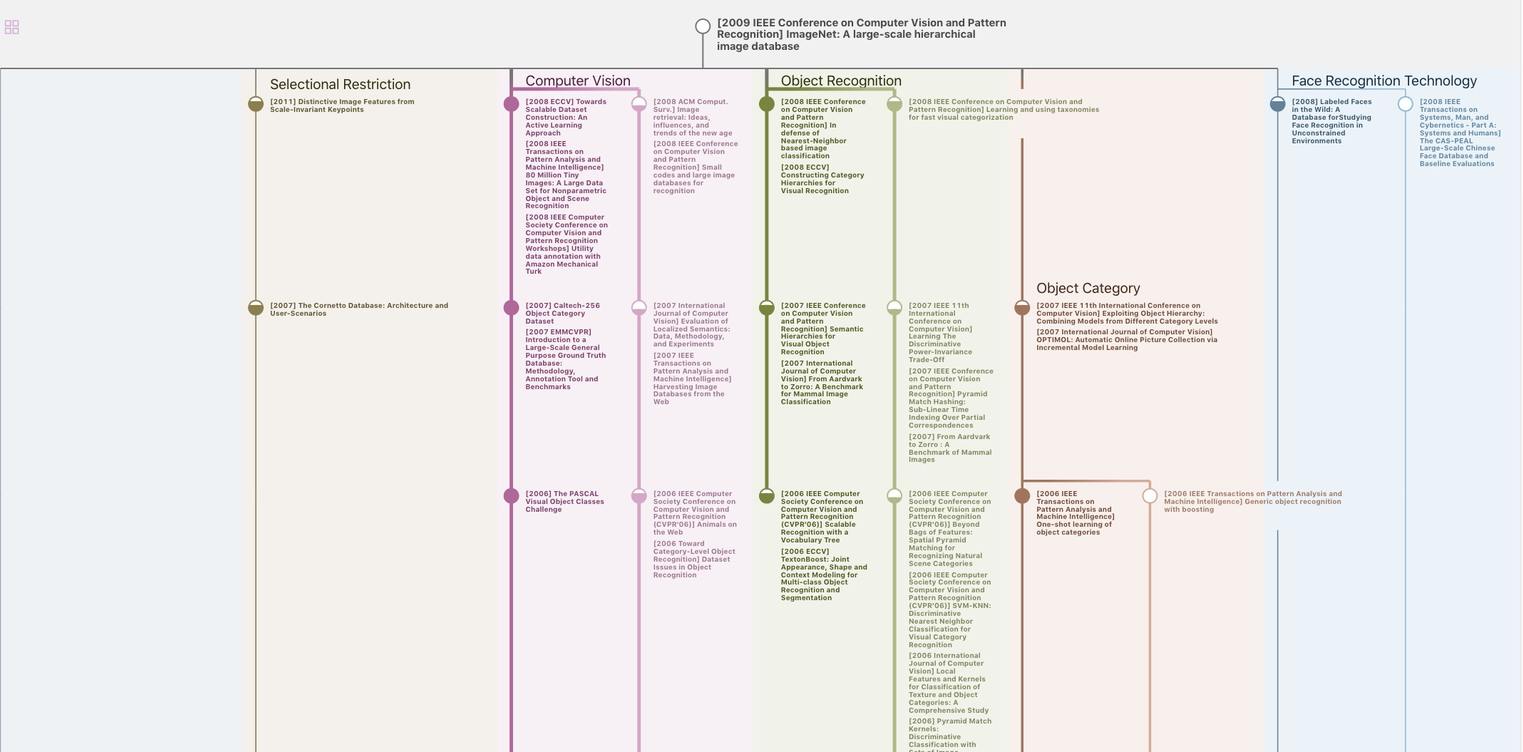
生成溯源树,研究论文发展脉络
Chat Paper
正在生成论文摘要