Diversify Search Results Through Graph Attentive Document Interaction
International Conference on Database Systems for Advanced Applications (DASFAA)(2022)
摘要
The goal of search result diversification is to retrieve diverse documents to meet as many different information needs as possible. Graph neural networks provide a feasible way to capture the sophisticated relationship between candidate documents, while existing graph-based diversification methods require an extra model to construct the graph, which will bring about the problem of error accumulation. In this paper, we propose a novel model to address this problem. Specifically, we maintain a document interaction graph for the candidate documents of each query to model the diverse information interactions between them. To extract latent diversity features, we adopt graph attention networks (GATs) to update the representation of each document by aggregating its neighbors with learnable weights, which enables our model not dependent on knowing the graph structure in advance. Finally, we simultaneously compute the ranking score of each candidate document with the extracted latent diversity features and the traditional relevance features, and the ranking can be acquired by sorting the scores. Experimental results on TREC Web Track benchmark datasets show that the proposed model outperforms existing state-of-the-art models.
更多查看译文
关键词
Search result diversification,Graph attention networks,Document interaction
AI 理解论文
溯源树
样例
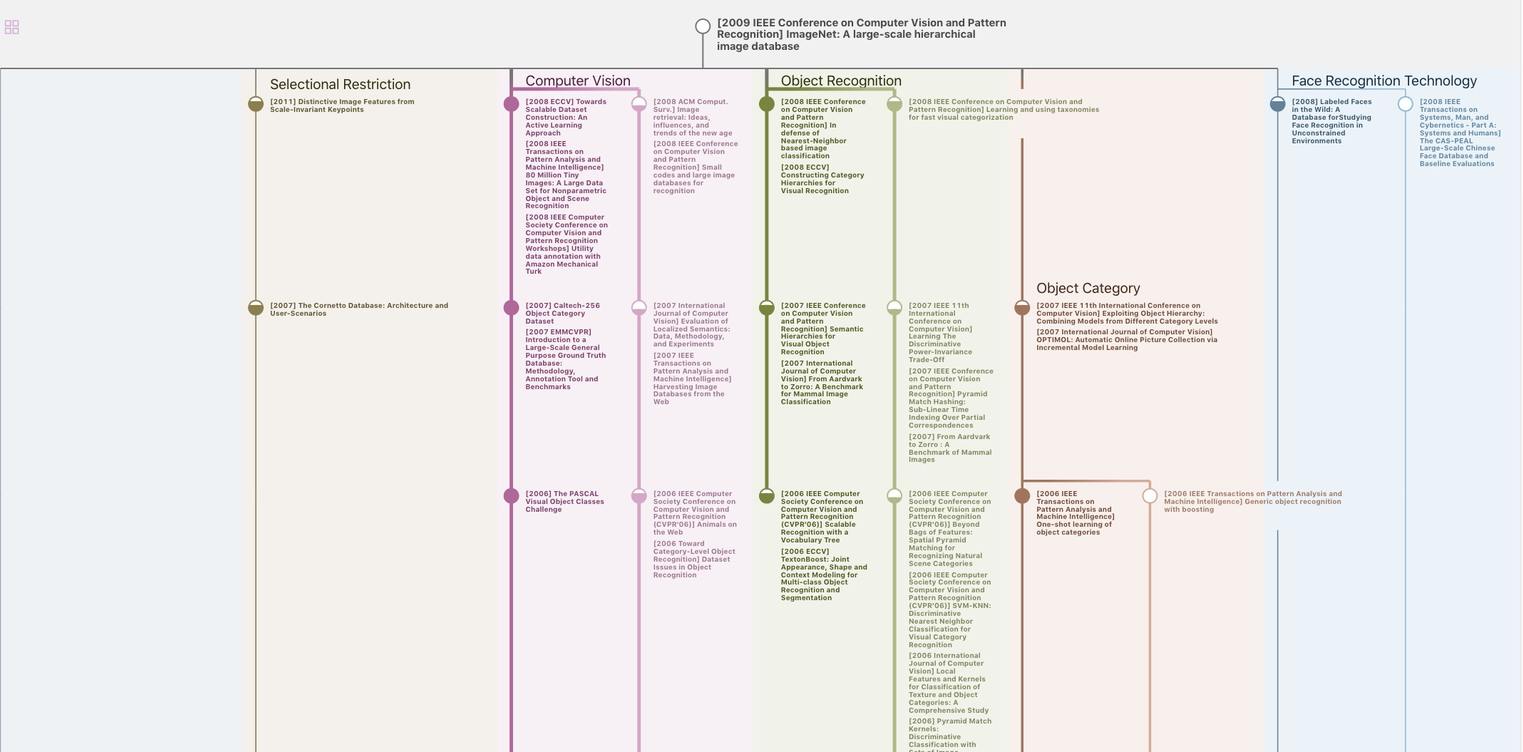
生成溯源树,研究论文发展脉络
Chat Paper
正在生成论文摘要