Depth-inspired Label Mining for Unsupervised RGB-D Salient Object Detection
International Multimedia Conference(2022)
摘要
ABSTRACTExisting deep learning-based unsupervised Salient Object Detection (SOD) methods heavily rely on the pseudo labels predicted from handcrafted features. However, the pseudo ground truth obtained only from RGB space would easily bring undesirable noises, especially in some complex scenarios. This naturally leads to the incorporation of extra depth modality with RGB images for more robust object identification, namely RGB-D SOD. Compared with the well-studied unsupervised SOD in the RGB domain, deep unsupervised RGB-D SOD is a less explored direction in the literature. In this paper, we propose to tackle this task by introducing a novel systemic design for high-quality pseudo-label mining. Our framework consists of two key components, Depth-inspired Label Generation (DLG) and Multi-source Uncertainty-aware Label Optimization (MULO). In DLG, a lightweight deep network is designed for automatically producing pseudo labels from depth maps in a self-supervised manner. Then, MULO introduces an effective pseudo label optimization strategy by learning the uncertainty of the pseudo labels from the depth domain and heuristic features. Extensive experiments demonstrate that the proposed method significantly outperforms the state-of-the-art unsupervised methods on mainstream benchmarks.
更多查看译文
关键词
object detection,label mining,depth-inspired
AI 理解论文
溯源树
样例
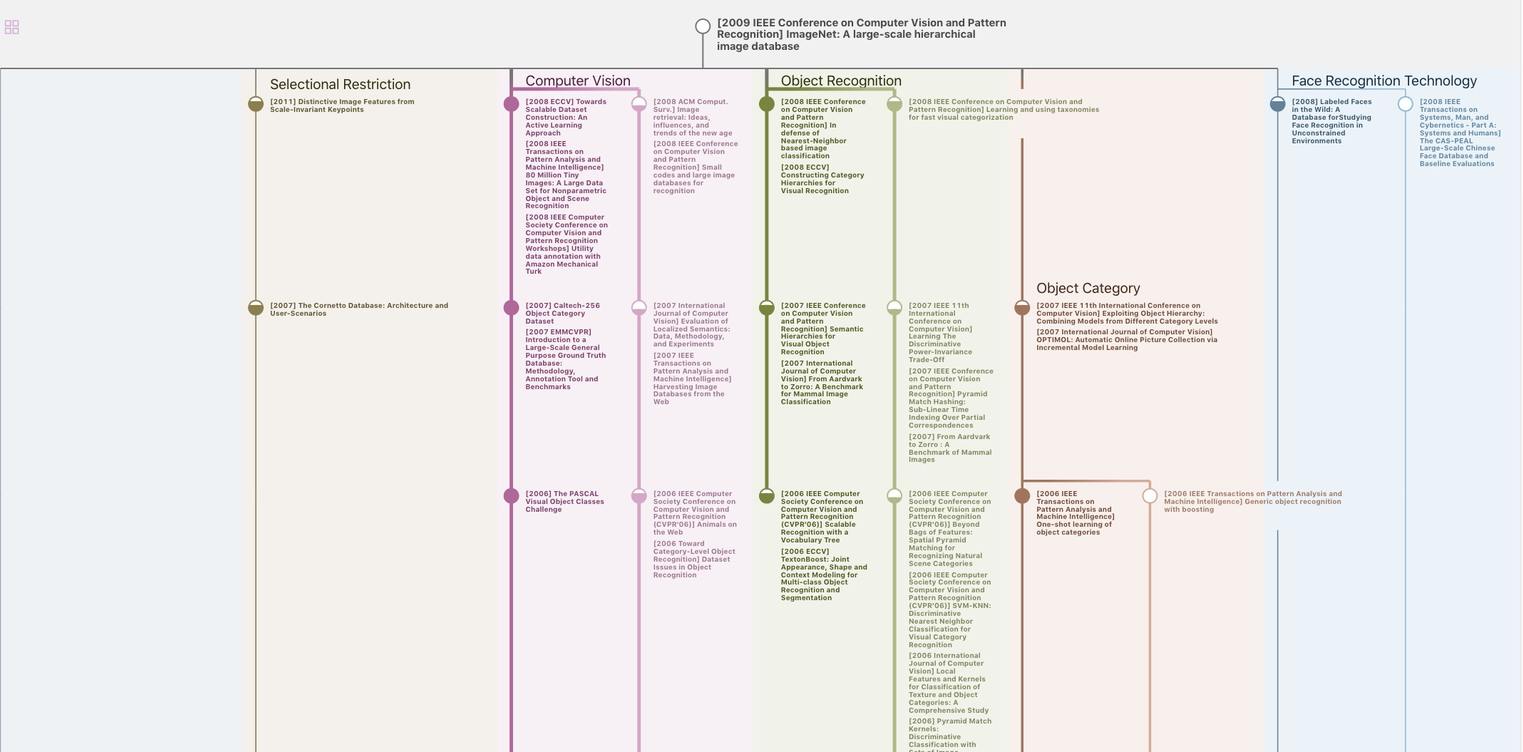
生成溯源树,研究论文发展脉络
Chat Paper
正在生成论文摘要