Patch-based Knowledge Distillation for Lifelong Person Re-Identification
International Multimedia Conference(2022)
摘要
ABSTRACTThe task of lifelong person re-identification aims to match a person across multiple cameras given continuous data streams. Similar to other lifelong learning tasks, it severely suffers from the so-called catastrophic forgetting problem, which refers to the notable performance degradation on previously-seen data after adapting the model to some newly incoming data. To alleviate it, a few existing methods have utilized knowledge distillation to enforce consistency between the original and adapted models. However, the effectiveness of such a strategy can be largely reduced facing the data distribution discrepancy between seen and new data. The hallmark of our work is using adaptively-chosen patches (rather than whole images as in other works) to pilot the forgetting-resistant distillation. Specifically, the technical contributions of our patch-based new solution are two-fold: first, a novel patch sampler is proposed. It is fully differentiable and trained to select a diverse set of image patches that stay crucial and discriminative under streaming data. Secondly, with those patches we curate a novel knowledge distillation framework. Valuable patch-level knowledge within individual patch features and mutual relations is well preserved by the two newly introduced distillation modules, further mitigating catastrophic forgetting. Extensive experiments on twelve person re-identification datasets clearly validate the superiority of our method over state-of-the-art competitors by large performance margins.
更多查看译文
关键词
knowledge distillation,person,patch-based,re-identification
AI 理解论文
溯源树
样例
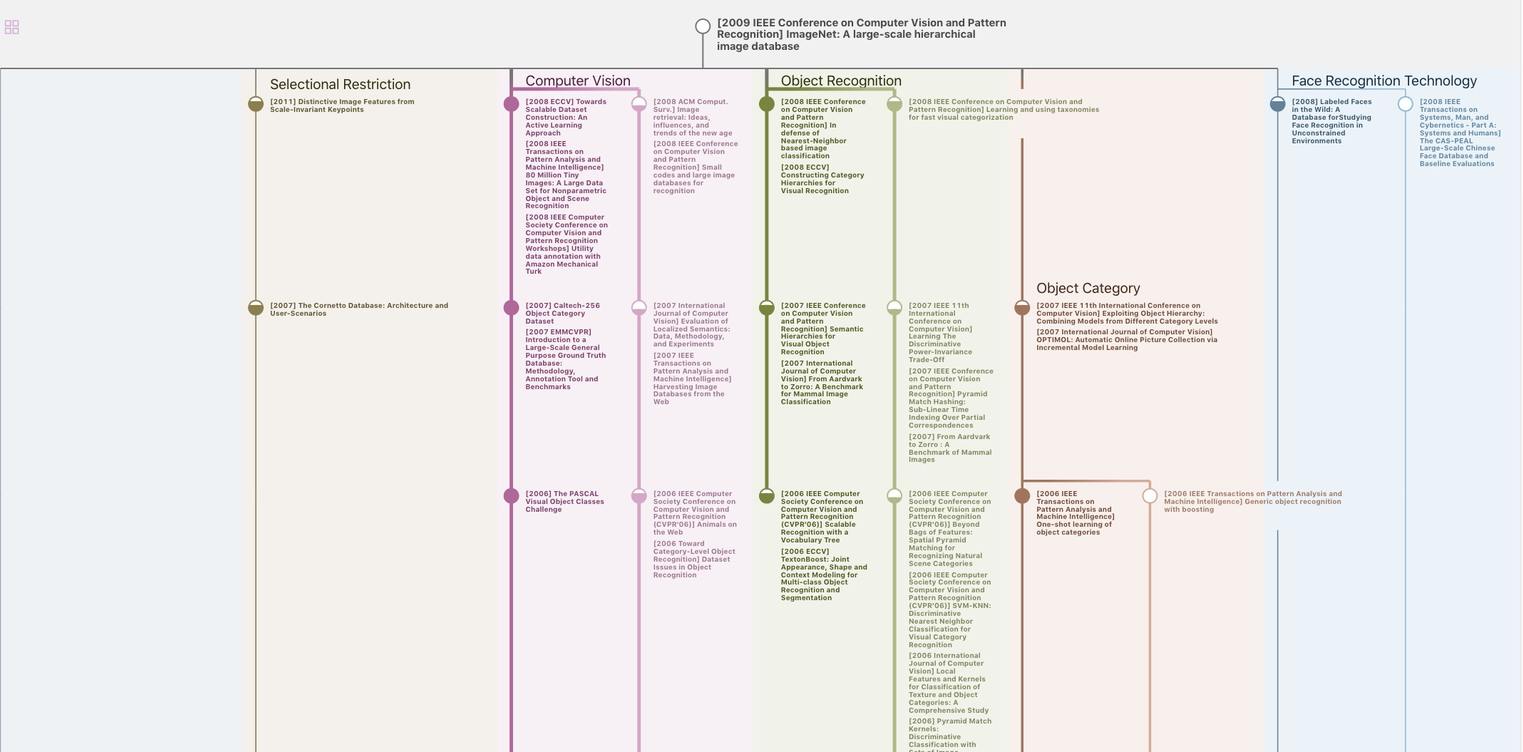
生成溯源树,研究论文发展脉络
Chat Paper
正在生成论文摘要