EliMRec: Eliminating Single-modal Bias in Multimedia Recommendation
International Multimedia Conference(2022)
摘要
ABSTRACTThe main idea of multimedia recommendation is to introduce the profile content of multimedia documents as an auxiliary, so as to endow recommenders with generalization ability and gain better performance. However, recent studies using non-uniform datasets roughly fuse single-modal features into multi-modal features and adopt the strategy of directly maximizing the likelihood of user preference scores, leading to the single-modal bias. Owing to the defect in architecture, there is still room for improvement for recent multimedia recommendation. In this paper, we propose EliMRec, a generic and modal-agnostic framework to eliminate the single-modal bias in multimedia recommendation. From our observation, biased predictive reasoning is influenced directly by the single modality rather than considering the all given multiple views of the item. Through the novel perspective of causal inference, we manage to explain the single-modal issue and exploit the inner working of multi-modal fusion. To eliminate single-modal bias, we enhance the bias-capture ability of a general multimedia recommendation framework and imagine several counterfactual worlds that control one modality variant with other modality fixed or blank. Truth to be told, counterfactual analysis enables us to identify and eliminate bias lying in the direct effect from single-modal features to the preference score. Extensive experiments on real-world datasets demonstrate that our method significantly improves over several state-of-the-art baselines like LightGCN and MMGCN. Codes are available at https://github.com/Xiaohao-Liu/EliMRec.
更多查看译文
关键词
multimedia recommendation,single-modal
AI 理解论文
溯源树
样例
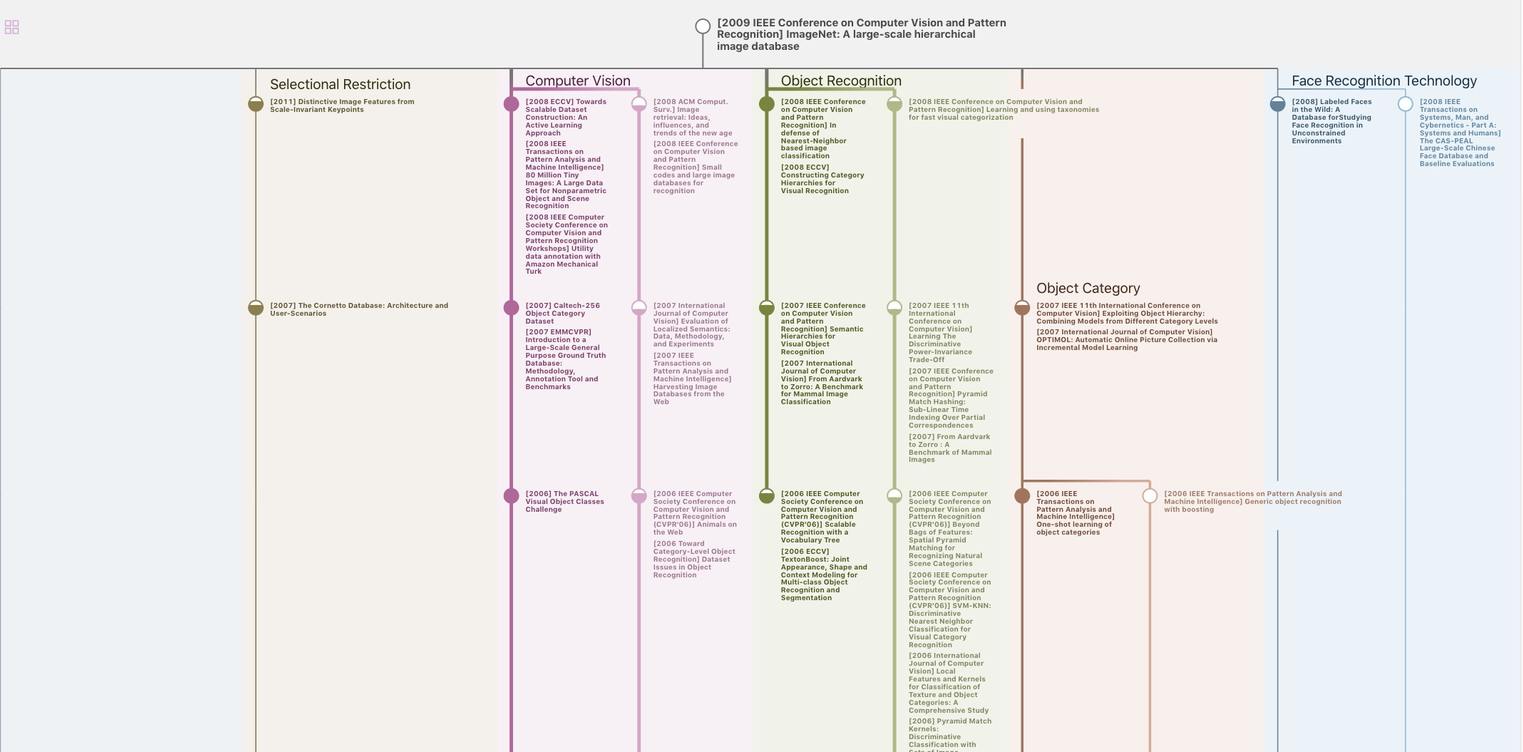
生成溯源树,研究论文发展脉络
Chat Paper
正在生成论文摘要