RINO: Robust INner and Outer Approximated Reachability of Neural Networks Controlled Systems
COMPUTER AIDED VERIFICATION (CAV 2022), PT I(2022)
摘要
We present a unified approach, implemented in the RINO tool, for the computation of inner and outer-approximations of reachable sets of discrete-time and continuous-time dynamical systems, possibly controlled by neural networks with differentiable activation functions. RINO combines a zonotopic set representation with generalized mean-value AE extensions to compute under and over-approximations of the robust range of differentiable functions, and applies these techniques to the particular case of learning-enabled dynamical systems. The AE extensions require an efficient and accurate evaluation of the function and its Jacobian with respect to the inputs and initial conditions. For continuous-time systems, possibly controlled by neural networks, the function to evaluate is the solution of the dynamical system. It is over-approximated in RINO using Taylor methods in time coupled with a set-based evaluation with zonotopes. We demonstrate the good performances of RINO compared to state-of-the art tools Verisig 2.0 and ReachNN* on a set of classical benchmark examples of neural network controlled closed loop systems. For generally comparable precision to Verisig 2.0 and higher precision than ReachNN*, RINO is always at least one order of magnitude faster, while also computing the more involved inner-approximations that the other tools do not compute.
更多查看译文
关键词
Neural networks verification, Reachability analysis, Robustness, Inner-approximation
AI 理解论文
溯源树
样例
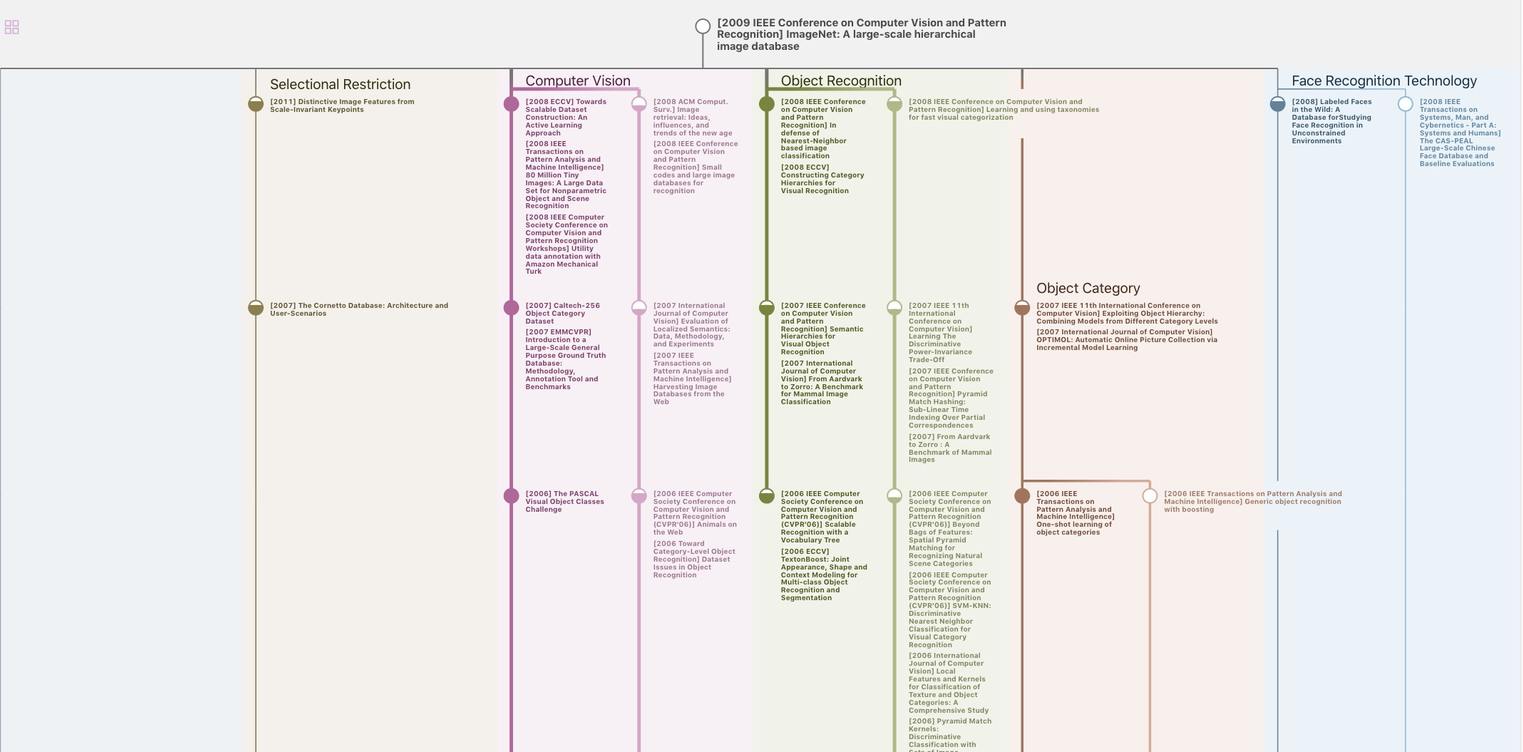
生成溯源树,研究论文发展脉络
Chat Paper
正在生成论文摘要