Prediction Intervals for Learned Cardinality Estimation: An Experimental Evaluation
2022 IEEE 38TH INTERNATIONAL CONFERENCE ON DATA ENGINEERING (ICDE 2022)(2022)
摘要
Cardinality estimation is a fundamental and challenging problem in query optimization. Recently, a number of learned models have been proposed for this task. Often, these models significantly outperform traditional approaches in terms of accuracy. One of the stumbling blocks that prevents their increased adoption is that the learned models do not quantify the uncertainty of their estimates. It is desirable to associate each cardinality estimate of the model with a prediction interval that will contain the true cardinality with an user-specified probability. The size of the prediction interval encodes the uncertainty allowing the query optimizer to make an informed decision. For example, knowing that the cardinality of a query q lies between 1 - 3% of the relation size with high probability is more informative than a single point estimate of 2%. While there has been some prior work on deriving bounds for traditional methods (such as sampling or histograms), they are not directly applicable for the learned models for cardinality estimation. In this paper, we conduct a systematic investigation of potential approaches for obtaining prediction intervals. We enumerate the list of desirable properties such as the ability to wrap around a learned model without significant internal modification and providing bounds with theoretical guarantees in a distribution agnostic manner among others. Based on an extensive literature survey, we identify four practical and high quality approaches for uncertainty quantification that satisfies these criteria. They span a wide spectrum in terms of theoretical guarantees, width of prediction interval and time taken for computing the prediction intervals. We conduct extensive experimental analysis of the efficacy of these approaches over three diverse and representative cardinality estimation algorithms. Our experiments covers diverse workloads involving both point and range queries and highlights the inherent trade-offs. Our results show that it is possible to obtain accurate prediction intervals in an efficient manner thereby opening up new avenues for future research.
更多查看译文
关键词
cardinality estimation,prediction intervals,conformal prediction,query optimization
AI 理解论文
溯源树
样例
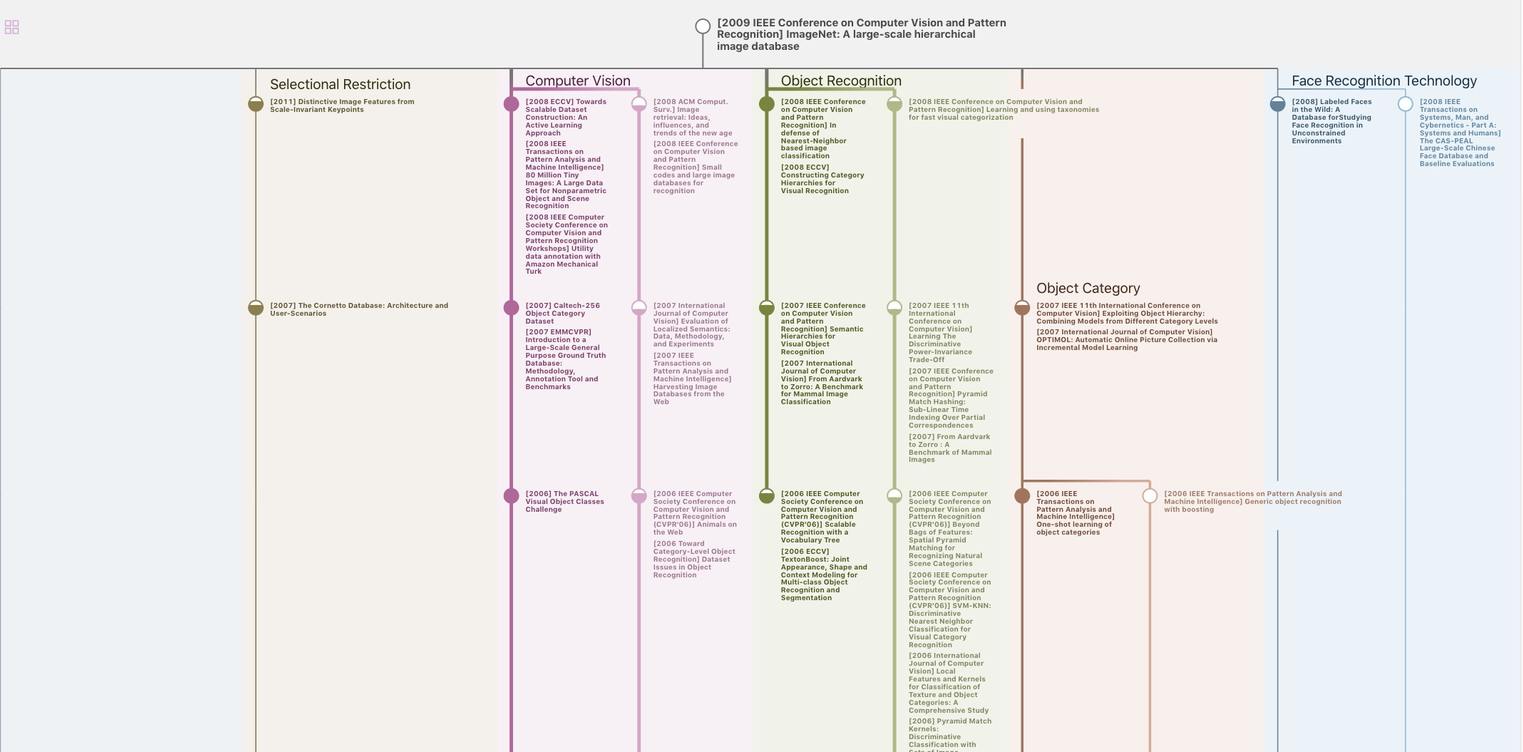
生成溯源树,研究论文发展脉络
Chat Paper
正在生成论文摘要