Discovering Actual Delivery Locations from Mis-Annotated Couriers' Trajectories
2022 IEEE 38TH INTERNATIONAL CONFERENCE ON DATA ENGINEERING (ICDE 2022)(2022)
摘要
Delivery locations are fundamental data source for intelligent logistics, which can be used in route planning, arrival time estimation, parcel allocation, etc. Using the Geocoded waybill location of an address as the delivery location is not sufficient, due to wrong address parsing, coarse-grained POI database, or different preferences of customers. To mitigate the insufficiency of Geocoding, some methods have been proposed, which utilize couriers' locations when waybills are confirmed to be delivered for delivery location inference. Nevertheless, these methods highly rely on the quality of couriers' annotations and fail when couriers confirm deliveries with delays. We propose to infer actual delivery locations of addresses from couriers' trajectories. This idea lies on an observation that the semantics of delivering a parcel are well captured by couriers' trajectories (e.g., a stay point would be generated when a delivery occurs), which holds even couriers confirm deliveries with delays. Specifically, we design Delivery Location Inference under Mis-Annotation (DLInfMA), which (1) generates location candidates from stay points in couriers' trajectories; (2) extracts features from both an address and its location candidates; and (3) uses an attention-based neural network model LocMatcher to predict the delivery location for each address. Experiments on two real-world datasets from JD Logistics as well as synthetic datasets demonstrate the effectiveness, robustness and scalability of DLInfMA. We also present a deployed system along with two applications based on DLInfMA.
更多查看译文
关键词
trajectory data mining, volunteered geographic information, toponym resolution
AI 理解论文
溯源树
样例
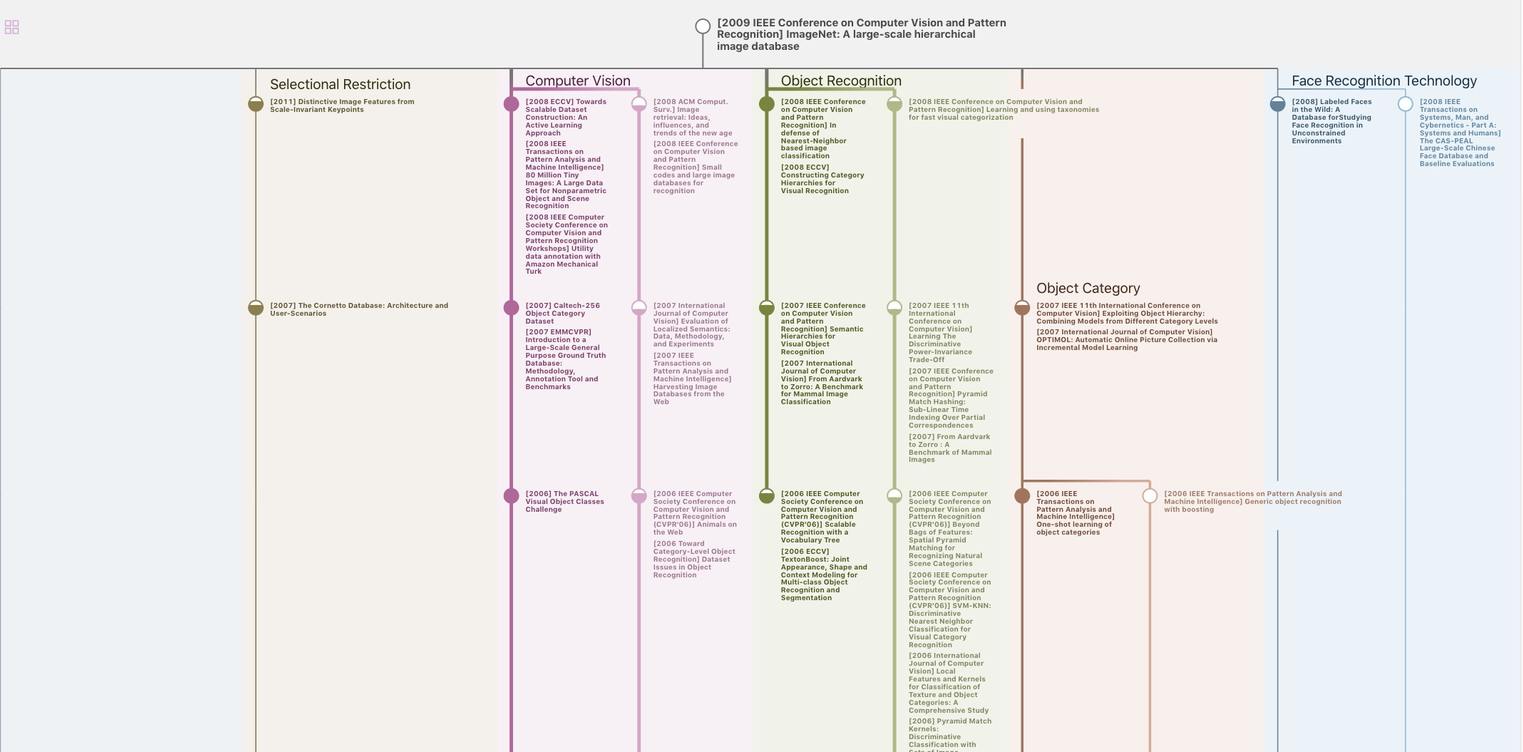
生成溯源树,研究论文发展脉络
Chat Paper
正在生成论文摘要