Video-zilla: An Indexing Layer for Large-Scale Video Analytics
PROCEEDINGS OF THE 2022 INTERNATIONAL CONFERENCE ON MANAGEMENT OF DATA (SIGMOD '22)(2022)
摘要
Pervasive deployment of surveillance cameras today poses enormous scalability challenges to video analytics systems operating over many camera feeds. Currently, there are few indexing tools to organize video feeds beyond what is provided by a standardfi le system. Recent video analytic systems implement application-specific frame profiling and sampling techniques to reduce the number of raw videos processed, leveraging frame-level redundancy or manually labeled spatial-temporal correlation between cameras. This paper presents Video-zilla, a standalone indexing layer between video query systems and a video store to organize video data. We propose a video data unit abstraction, semantic video stream (SVS), based on a notion of distance between objects in the video. SVS implicitly captures scenes, which is missing from current video content characterization and a middle ground between individual frames and an entire camera feed. We then build a hierarchical index that exposes the semantic similarity both within and across camera feeds, such that Video-zilla can quickly cluster video feeds based on their content semantics without manual labeling. We implement and evaluate Video-zilla in three use cases: object identification queries, clustering for training specialized DNNs, and archival services. In all three cases, Video-zilla reduces the time complexity of inter-camera video analytics from linear with the number of cameras to sublinear, and reduces query resource usage by up to 14x compared to using frame-level or spatial-temporal similarity built into existing query systems.
更多查看译文
关键词
edge computing, video analytics
AI 理解论文
溯源树
样例
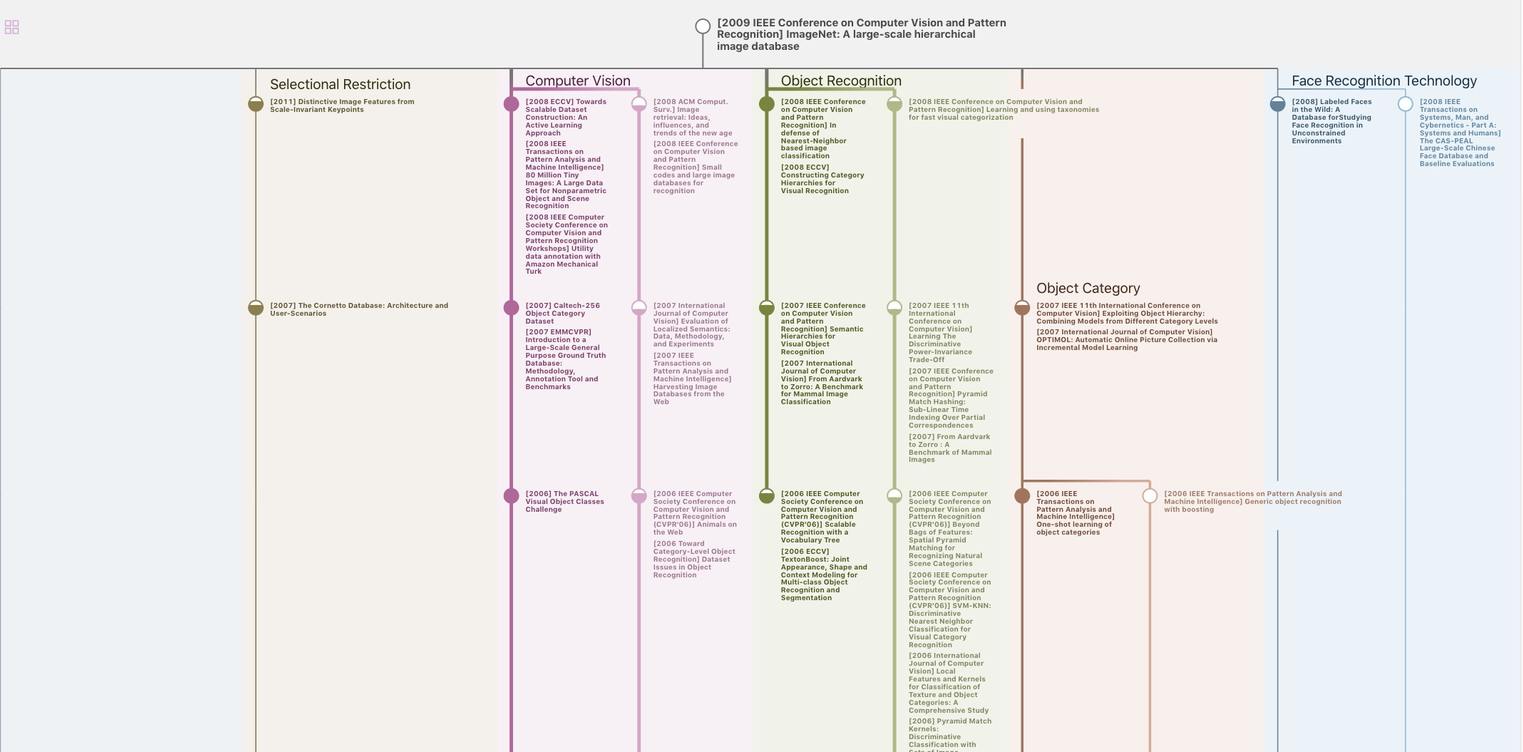
生成溯源树,研究论文发展脉络
Chat Paper
正在生成论文摘要