Auter: Automatically Tuning Multi-layer Network Buffers in Long-Distance Shadowsocks Networks
IEEE Conference on Computer Communications (INFOCOM)(2022)
摘要
To bypass network censorship, Shadowsocks is often deployed on long-distance transnational networks; however, such proxy networks are usually plagued by high latency, high packet loss rate, and unstable bandwidth. Most existing tuning solutions rely on hand-tuned heuristics, which cannot work well in the volatile Shadowsocks networks due to the labor intensive and time-consuming properties. In this paper, we propose Auter, which automatically tunes multi-layer buffer parameters with reinforcement learning (RL) to improve the performance of Shadowsocks in long-distance networks. The key insight behind Auter is that different network environments require different sizes of buffers to achieve sufficiently good performance. Hence, Auter continuously learns a tuning policy from volatile network states and dynamically alter sizes of multi-buffers for high network performance. We prototype Auter and evaluate its effectiveness under various real networks. Our experimental results show that Auter can effectively improve network performance, up to 40.5% throughput increase in real networks. Besides, we demonstrate that Auter outperforms all the existing tuning schemes.
更多查看译文
关键词
networks,multi-layer,long-distance
AI 理解论文
溯源树
样例
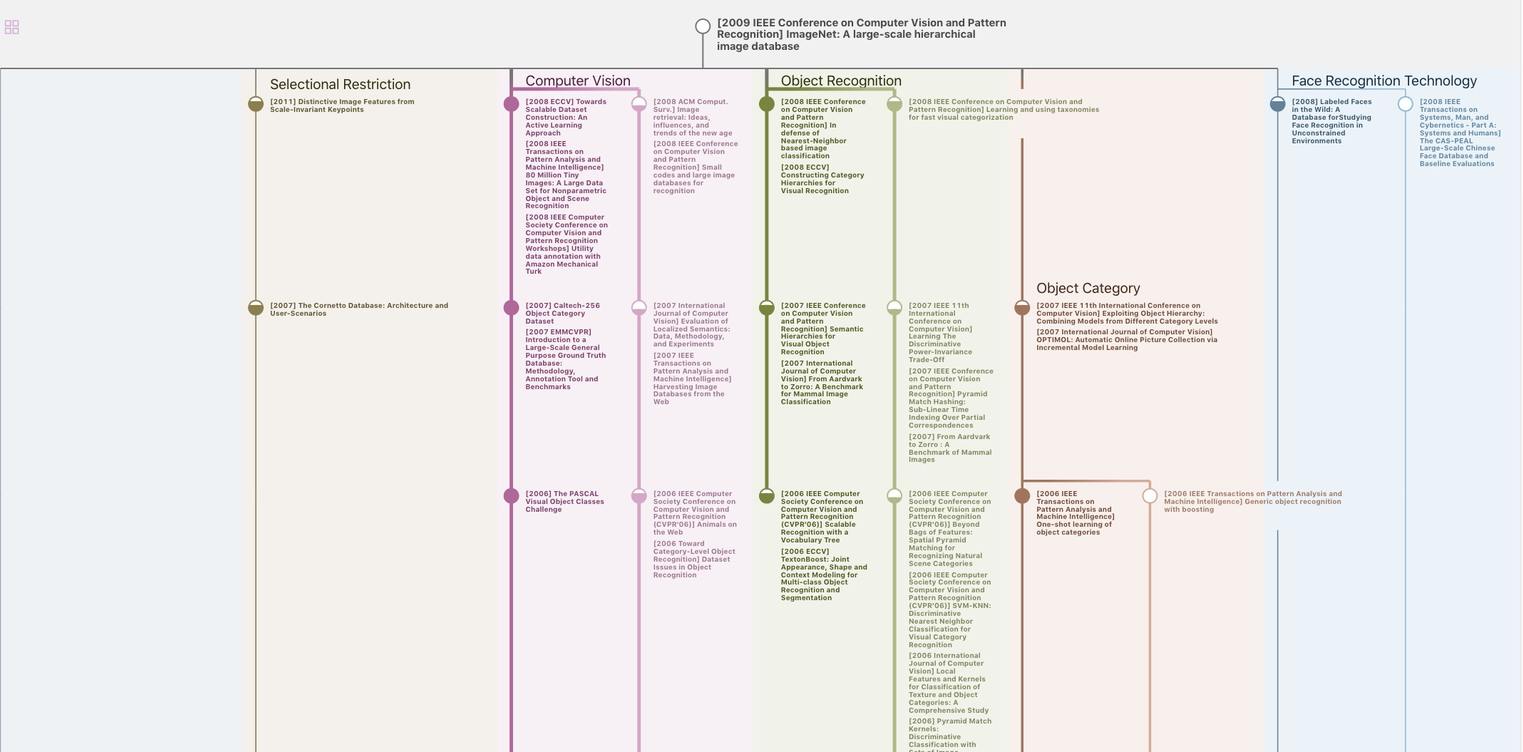
生成溯源树,研究论文发展脉络
Chat Paper
正在生成论文摘要