HDPG: hyperdimensional policy-based reinforcement learning for continuous control
Design Automation Conference (DAC)(2022)
摘要
Traditional robot control or more general continuous control tasks often rely on carefully hand-crafted classic control methods. These models often lack the self-learning adaptability and intelligence to achieve human-level control. On the other hand, recent advancements in Reinforcement Learning (RL) present algorithms that have the capability of human-like learning. The integration of Deep Neural Networks (DNN) and RL thereby enables autonomous learning in robot control tasks. However, DNN-based RL brings both high-quality learning and high computation cost, which is no longer ideal for currently fast-growing edge computing scenarios. In this paper, we introduce HDPG, a highly efficient policy based RL algorithm using I lyperdimensional Computing. Hyperdimensional computing is a lightweight brain-inspired learning methodology; its holistic representation of information leads to a well-defined set of hardware-friendly high-dimensional operations. Our HDPG fully exploits the efficient HOC for high-quality state value approximation and policy gradient update. In our experiments, we use HDPG for robotics tasks with continuous action space and achieve significantly higher rewards than DNN-based RL. Our evaluation also shows that HDPG achieves 4.7x faster and 5.3x higher energy efficiency than DNN-based RI, running on embedded FPGA.
更多查看译文
AI 理解论文
溯源树
样例
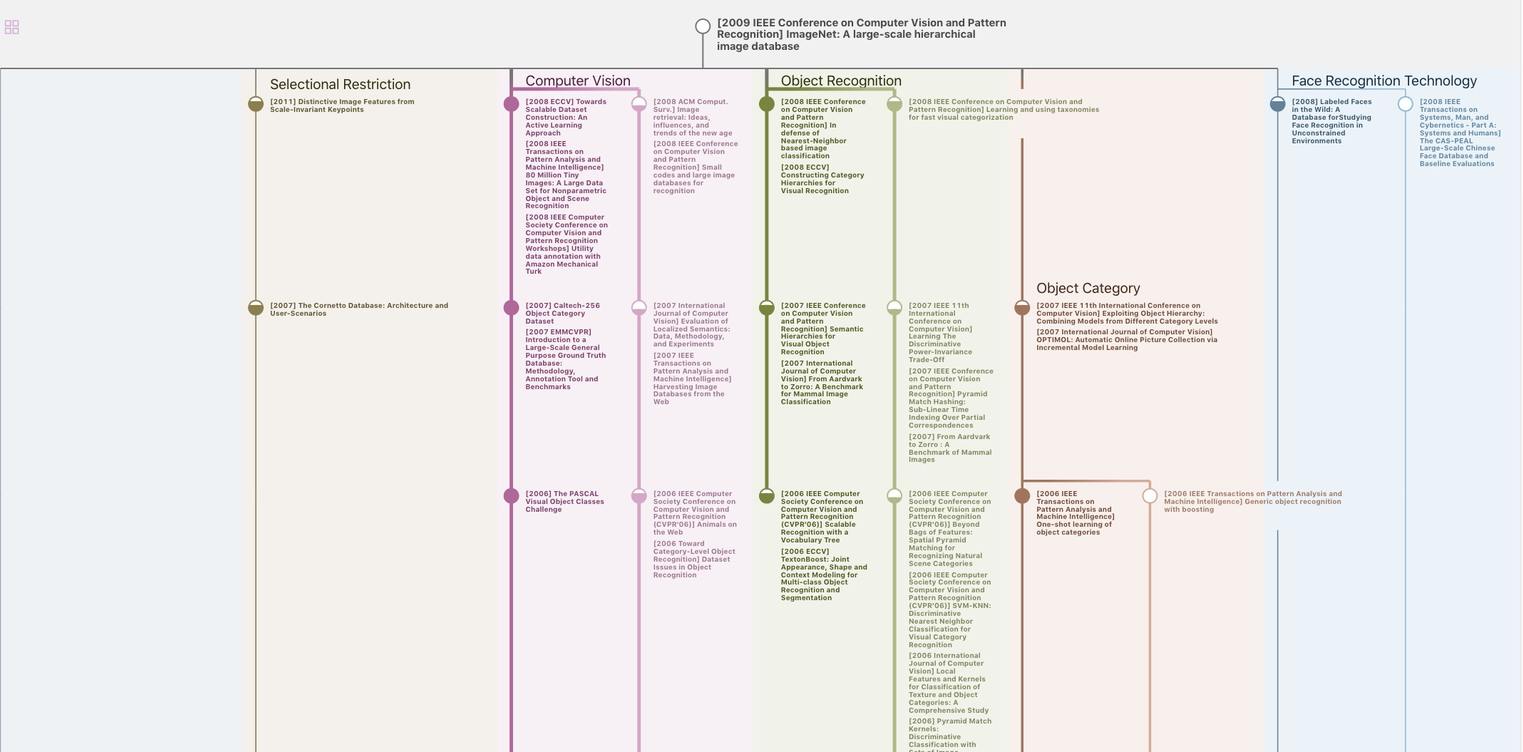
生成溯源树,研究论文发展脉络
Chat Paper
正在生成论文摘要