An energy-efficient seizure detection processor using event-driven multi-stage CNN classification and segmented data processing with adaptive channel selection
Design Automation Conference (DAC)(2022)
摘要
Recently wearable EEG monitoring devices with seizure detection processor using convolutional neural network (CNN) have been proposed to detect the seizure onset of patients in real time for alert or stimulation purpose. High energy-efficiency and accuracy are required for the seizure detection processor due to the tight energy constraint of wearable devices. However, the use of CNN and multi-channel processing nature of seizure detection result in significant energy consumption. In this work, an energy- efficient seizure detection processor is proposed, featuring multi-stage CNN classification, segmented data processing and adaptive channel selection to reduce the energy consumption while achieving high accuracy. The design has been fabricated and tested using a 55nm process technology. Compared with several state- of-the-art designs, the proposed design achieves the lowest energy per classification (0.32 mu J) with high sensitivity (97.78%) and low false positive rate per hour (0.5).
更多查看译文
关键词
Seizure Detection, Processor, Energy-Efficient, CNN
AI 理解论文
溯源树
样例
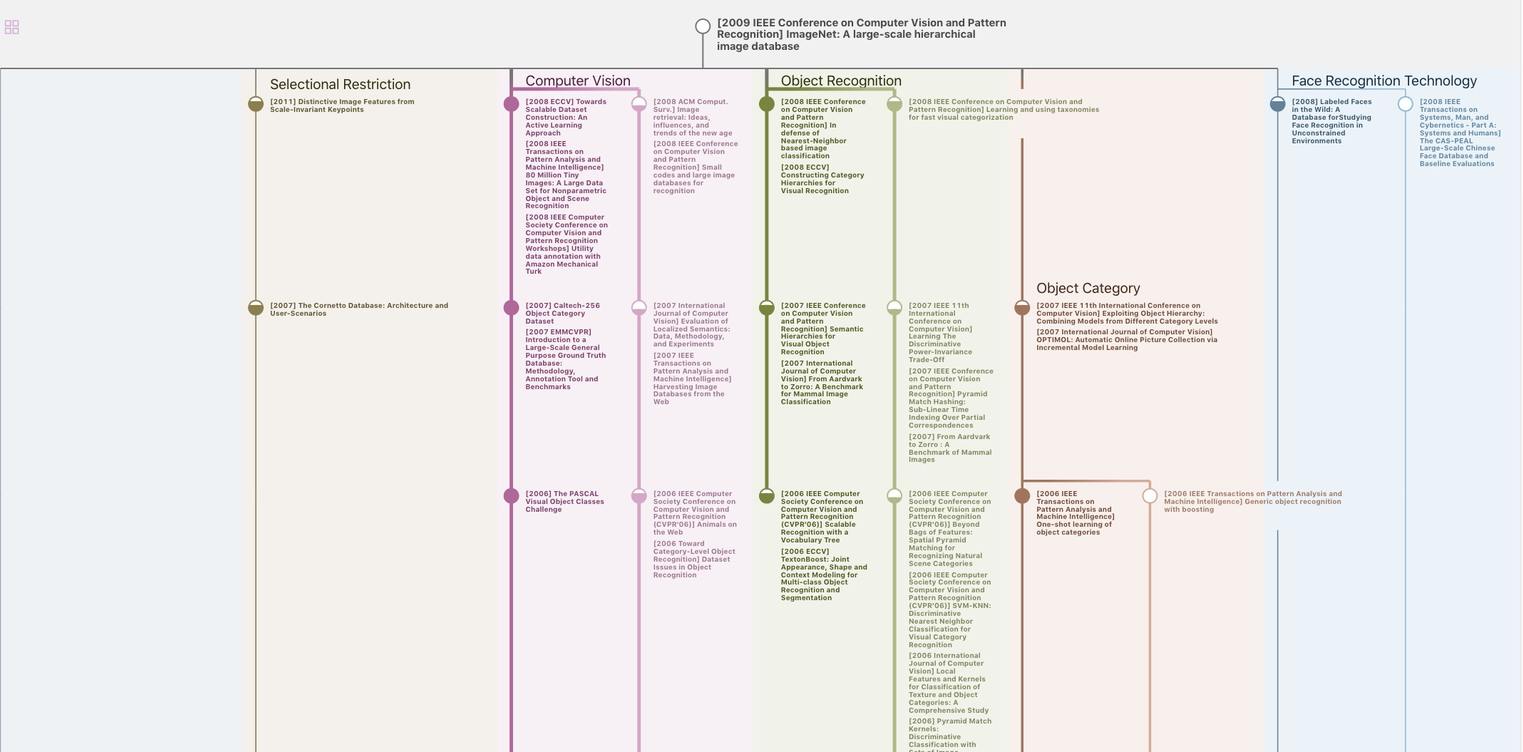
生成溯源树,研究论文发展脉络
Chat Paper
正在生成论文摘要