Functionality matters in netlist representation learning
Design Automation Conference(2022)
摘要
BSTRACTLearning feasible representation from raw gate-level netlists is essential for incorporating machine learning techniques in logic synthesis, physical design, or verification. Existing message-passing-based graph learning methodologies focus merely on graph topology while overlooking gate functionality, which often fails to capture underlying semantic, thus limiting their generalizability. To address the concern, we propose a novel netlist representation learning framework that utilizes a contrastive scheme to acquire generic functional knowledge from netlists effectively. We also propose a customized graph neural network (GNN) architecture that learns a set of independent aggregators to better cooperate with the above framework. Comprehensive experiments on multiple complex real-world designs demonstrate that our proposed solution significantly outperforms state-of-the-art netlist feature learning flows.
更多查看译文
关键词
representation,learning,functionality
AI 理解论文
溯源树
样例
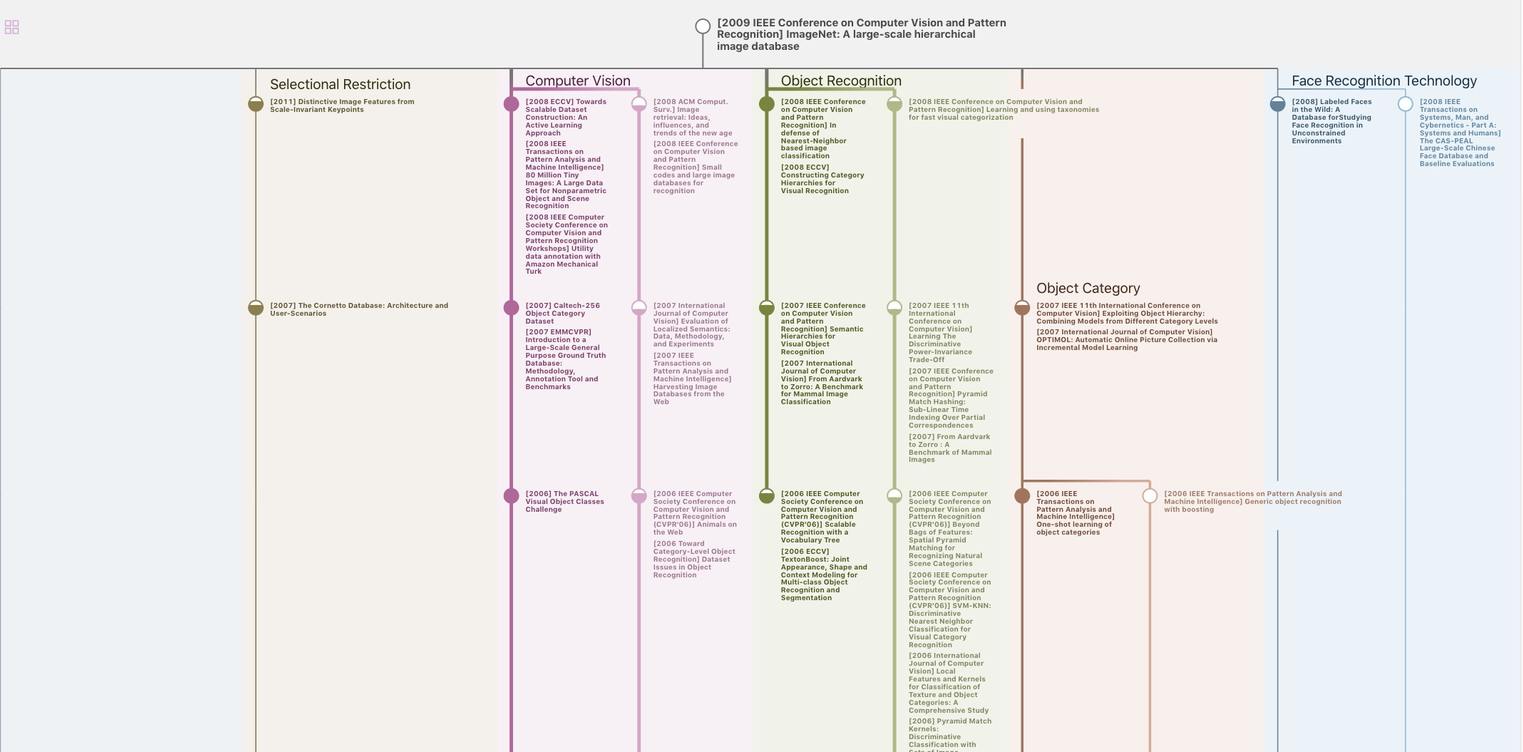
生成溯源树,研究论文发展脉络
Chat Paper
正在生成论文摘要