Deep Discriminant Non-negative Matrix Factorization Method for Image Clustering
INTELLIGENT COMPUTING THEORIES AND APPLICATION (ICIC 2022), PT I(2022)
摘要
Non-negative matrix factorization (NMF) is a single-layer decomposition algorithm for image data analysis and cannot reveal the intrinsic hierarchical-structure information hidden under the data. Moreover, it extracts features with weak discriminant power due to merely using unlabeled data. These flaws cause the undesirable performance of NMF in image clustering. To address the problems of NMF, this paper presents a novel deep discriminant NMF (DDNMF) approach via neural networks (NN). Our DDNMF model is established by employing the loss function of neural networks and the supervised regularization terms. The optimization problem of DDNMF is solved utilizing gradient descent method (GDM). The proposed DDNMF algorithm is proven to be convergent and stable. Some related NMF-based algorithms are selected for comparison on two facial image datasets. The experimental results confirm the effectiveness and superior performance of our DDNMF method in image clustering tasks.
更多查看译文
关键词
Non-negative matrix factorization, Neural networks, Deep feature extraction, Image clustering
AI 理解论文
溯源树
样例
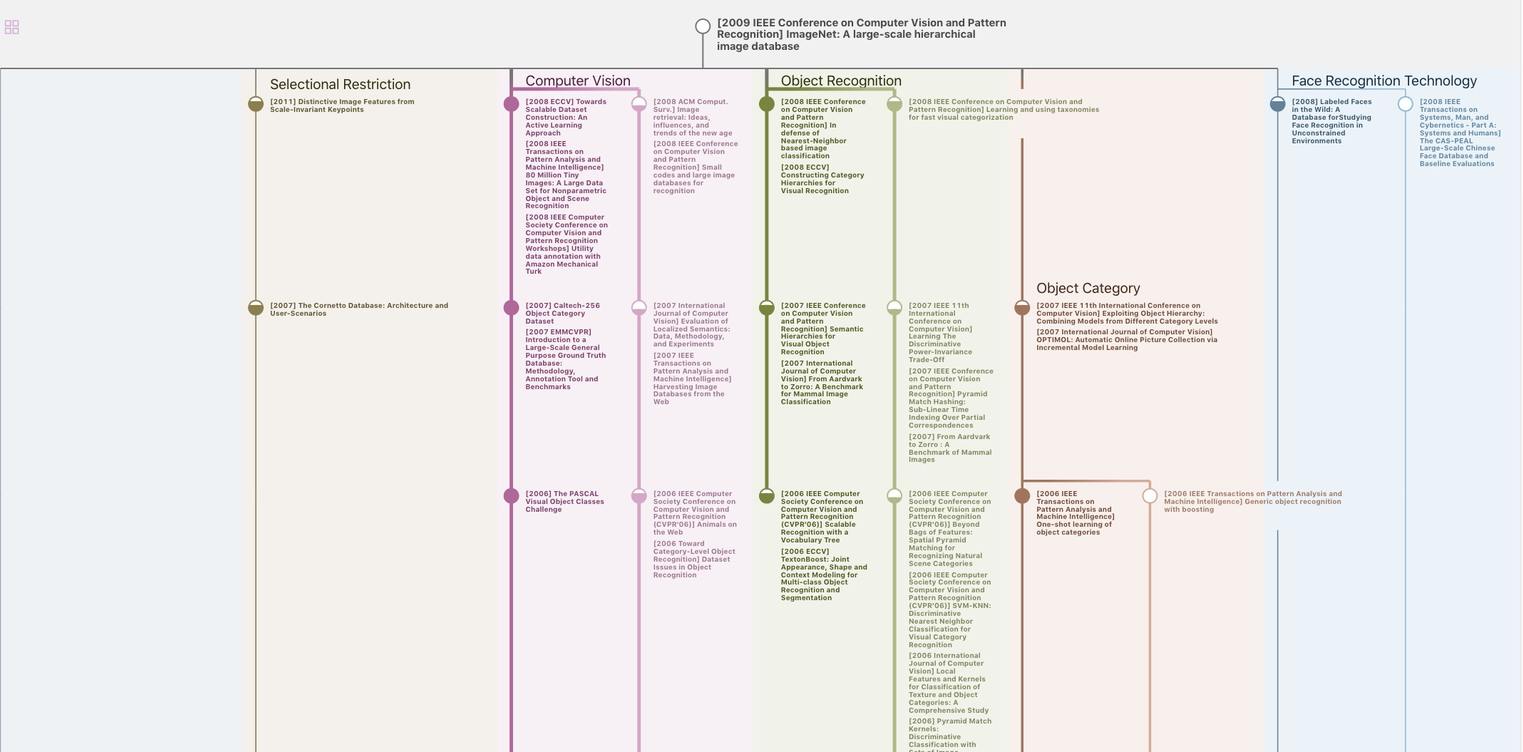
生成溯源树,研究论文发展脉络
Chat Paper
正在生成论文摘要