Improving Air Pollution Prediction System through Multimodal Deep Learning Model Optimization
Applied Sciences(2022)
摘要
Many forms of air pollution increase as science and technology rapidly advance. In particular, fine dust harms the human body, causing or worsening heart and lung-related diseases. In this study, the level of fine dust in Seoul after 8 h is predicted to prevent health damage in advance. We construct a dataset by combining two modalities (i.e., numerical and image data) for accurate prediction. In addition, we propose a multimodal deep learning model combining a Long Short Term Memory (LSTM) and Convolutional Neural Network (CNN). An LSTM AutoEncoder is chosen as a model for numerical time series data processing and basic CNN. A Visual Geometry Group Neural Network (VGGNet) (VGG16, VGG19) is also chosen as a CNN model for image processing to compare performance differences according to network depth. The VGGNet is a standard deep CNN architecture with multiple layers. Our multimodal deep learning model using two modalities (i.e., numerical and image data) showed better performance than a single deep learning model using only one modality (numerical data). Specifically, the performance improved up to 14.16% when the VGG19 model, which has a deeper network, was used rather than the VGG16 model.
更多查看译文
关键词
weather forecast, time series data, cropped image data, LSTM, CNN, VGGNet, multimodal deep learning
AI 理解论文
溯源树
样例
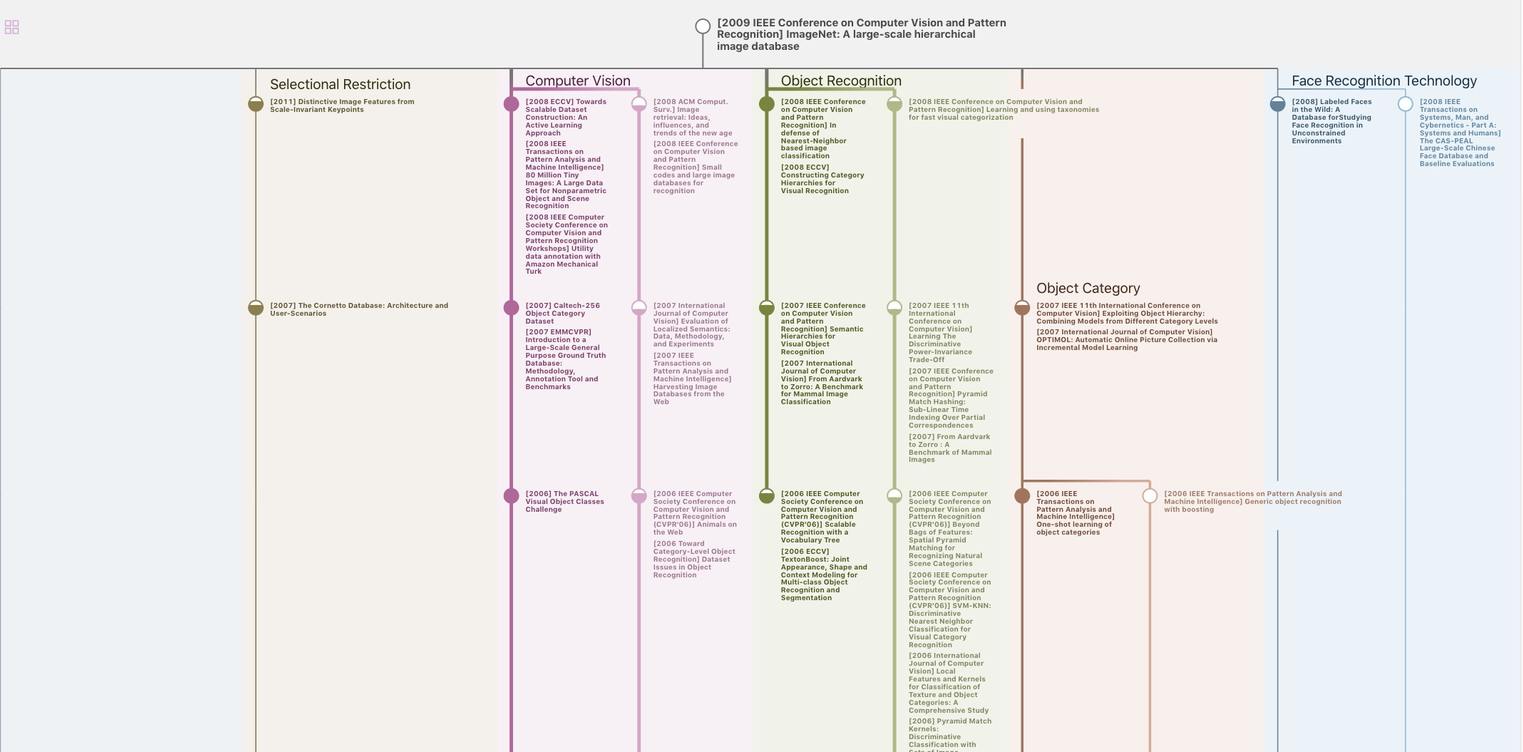
生成溯源树,研究论文发展脉络
Chat Paper
正在生成论文摘要