Model-Based Imitation Learning for Urban Driving
NeurIPS 2022(2022)
摘要
An accurate model of the environment and the dynamic agents acting in it offers great potential for improving motion planning. We present MILE: a Model-based Imitation LEarning approach to jointly learn a model of the world and a policy for autonomous driving. Our method leverages 3D geometry as an inductive bias and learns a highly compact latent space directly from high-resolution videos of expert demonstrations. Our model is trained on an offline corpus of urban driving data, without any online interaction with the environment. MILE improves upon prior state-of-the-art by 31% in driving score on the CARLA simulator when deployed in a completely new town and new weather conditions. Our model can predict diverse and plausible states and actions, that can be interpretably decoded to bird's-eye view semantic segmentation. Further, we demonstrate that it can execute complex driving manoeuvres from plans entirely predicted in imagination. Our approach is the first camera-only method that models static scene, dynamic scene, and ego-behaviour in an urban driving environment. The code and model weights are available at https://github.com/wayveai/mile.
更多查看译文
关键词
Autonomous Driving,Imitation Learning,World Models
AI 理解论文
溯源树
样例
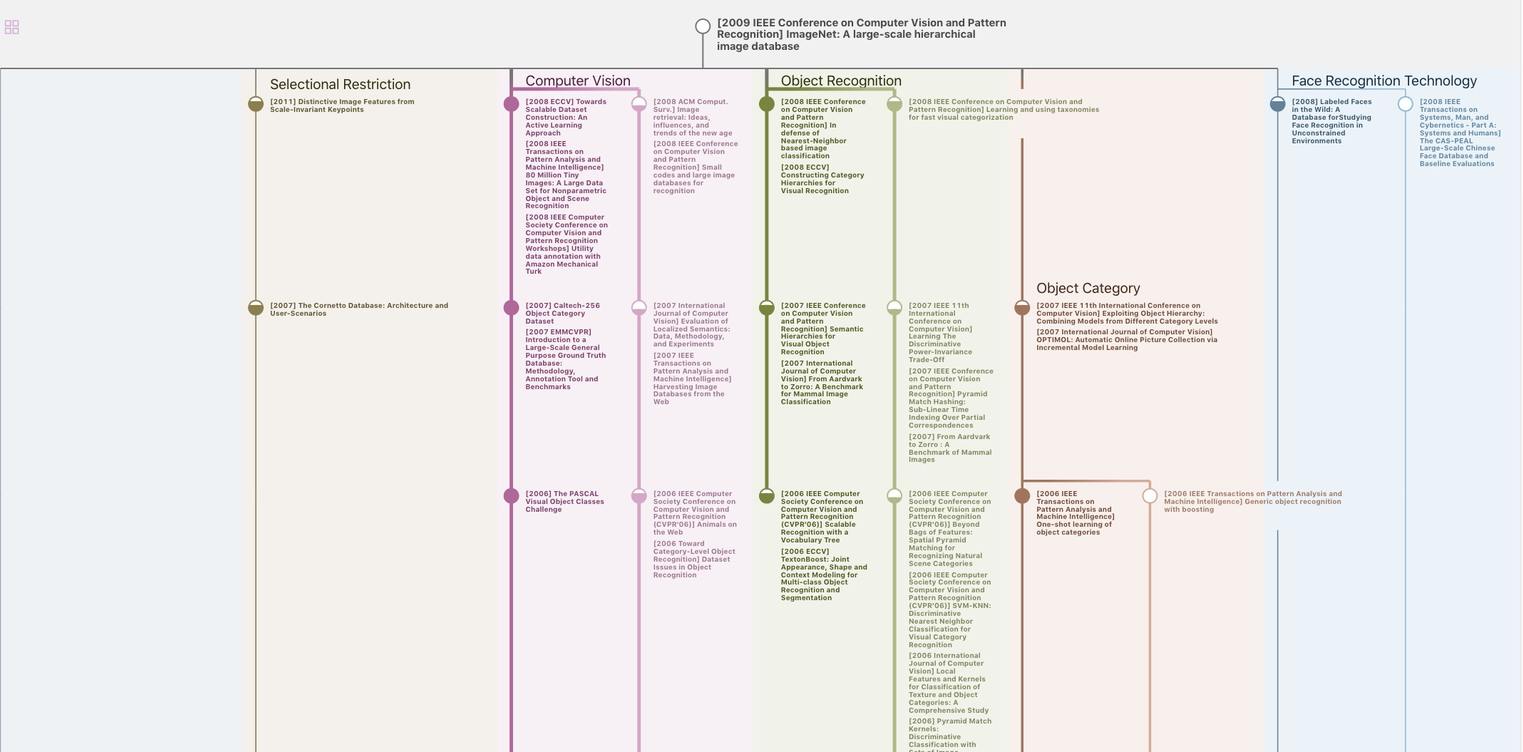
生成溯源树,研究论文发展脉络
Chat Paper
正在生成论文摘要