A Reinforcement Learning Approach to Estimating Long-term Treatment Effects
arxiv(2022)
摘要
Randomized experiments (a.k.a. A/B tests) are a powerful tool for estimating treatment effects, to inform decisions making in business, healthcare and other applications. In many problems, the treatment has a lasting effect that evolves over time. A limitation with randomized experiments is that they do not easily extend to measure long-term effects, since running long experiments is time-consuming and expensive. In this paper, we take a reinforcement learning (RL) approach that estimates the average reward in a Markov process. Motivated by real-world scenarios where the observed state transition is nonstationary, we develop a new algorithm for a class of nonstationary problems, and demonstrate promising results in two synthetic datasets and one online store dataset.
更多查看译文
关键词
reinforcement learning,off-policy evaluation,A/B testing
AI 理解论文
溯源树
样例
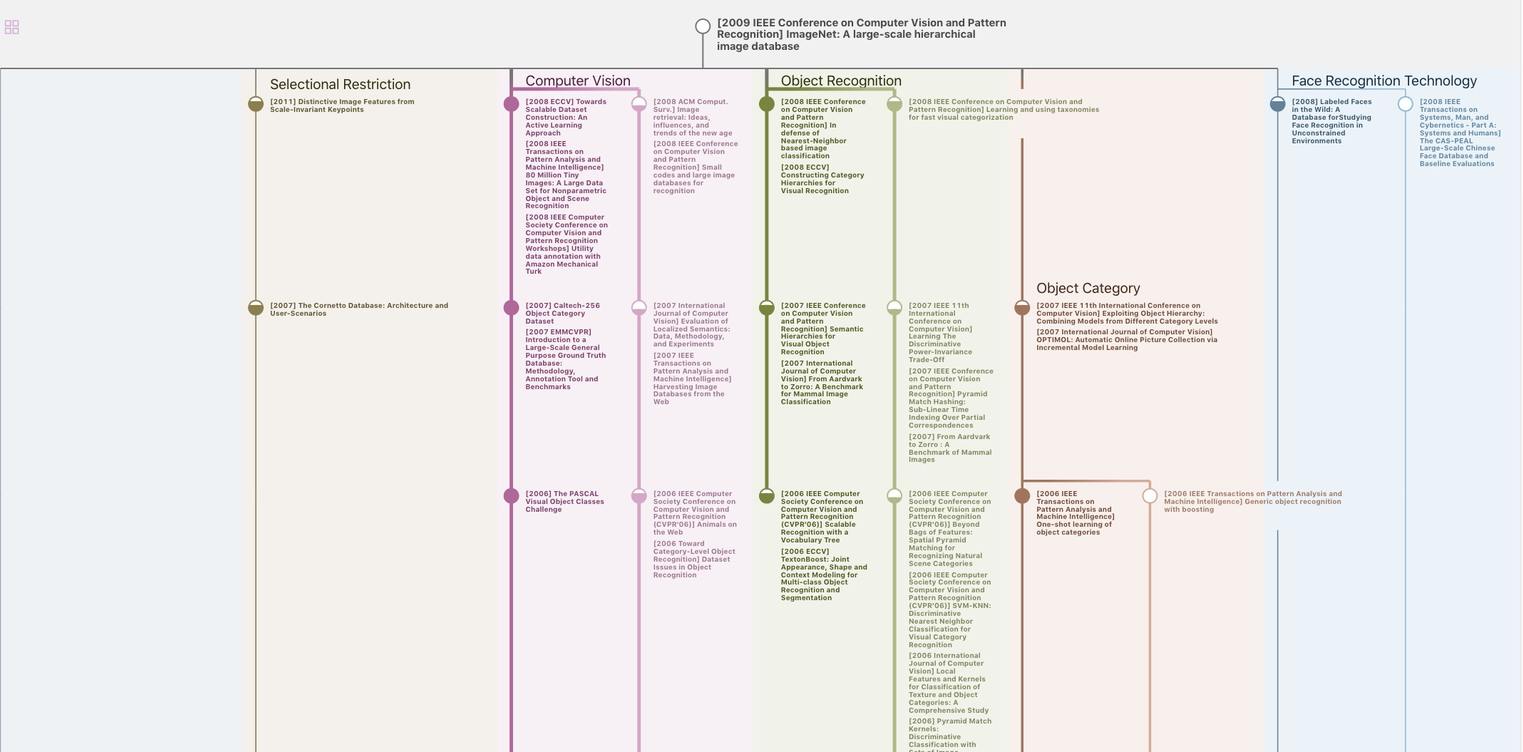
生成溯源树,研究论文发展脉络
Chat Paper
正在生成论文摘要