Environmental measurements and genetic effects for cancer survival integration data
2022 IEEE International Workshop on Metrology for Living Environment (MetroLivEn)(2022)
摘要
The identification of genetic and environment relations can guide health and medical interventions to understand the mechanisms of complex diseases on patients’ survival. Among existing tools for survival analysis, penalized variable selection methods emerged as a powerful methodology for the exploration of genes involved in cancer disorders when combined with environmental factors. Although regularization methods have many benefits for variable selection and network construction, they are limited in terms of accounting for multi-dimensional measurements. In fact, the main problem of high-dimensional data is the variety of omics profiles, their dimensionality and their intrinsic correlation, which make difficult the selection of explicative profiles and the integration of different data. In this paper, we developed a novel survival variable selection method for omic measurements taking into account environ-mental effects and genetic influences using the combination of reduction models and network-penalized Cox regression. Our model identifies regulatory genes that are involved in the disease under investigation while highlighting the relationship between survival outcomes and environmental factors. In particular, we applied our approach to a lung cancer dataset integrating gene expression data and copy number variations (CNVs) to investigate the environmental causes connected to patient-specific biological information to improve survival prediction.
更多查看译文
关键词
environmental factors,high-dimensional data,reduction analysis,variable selection,survival analysis
AI 理解论文
溯源树
样例
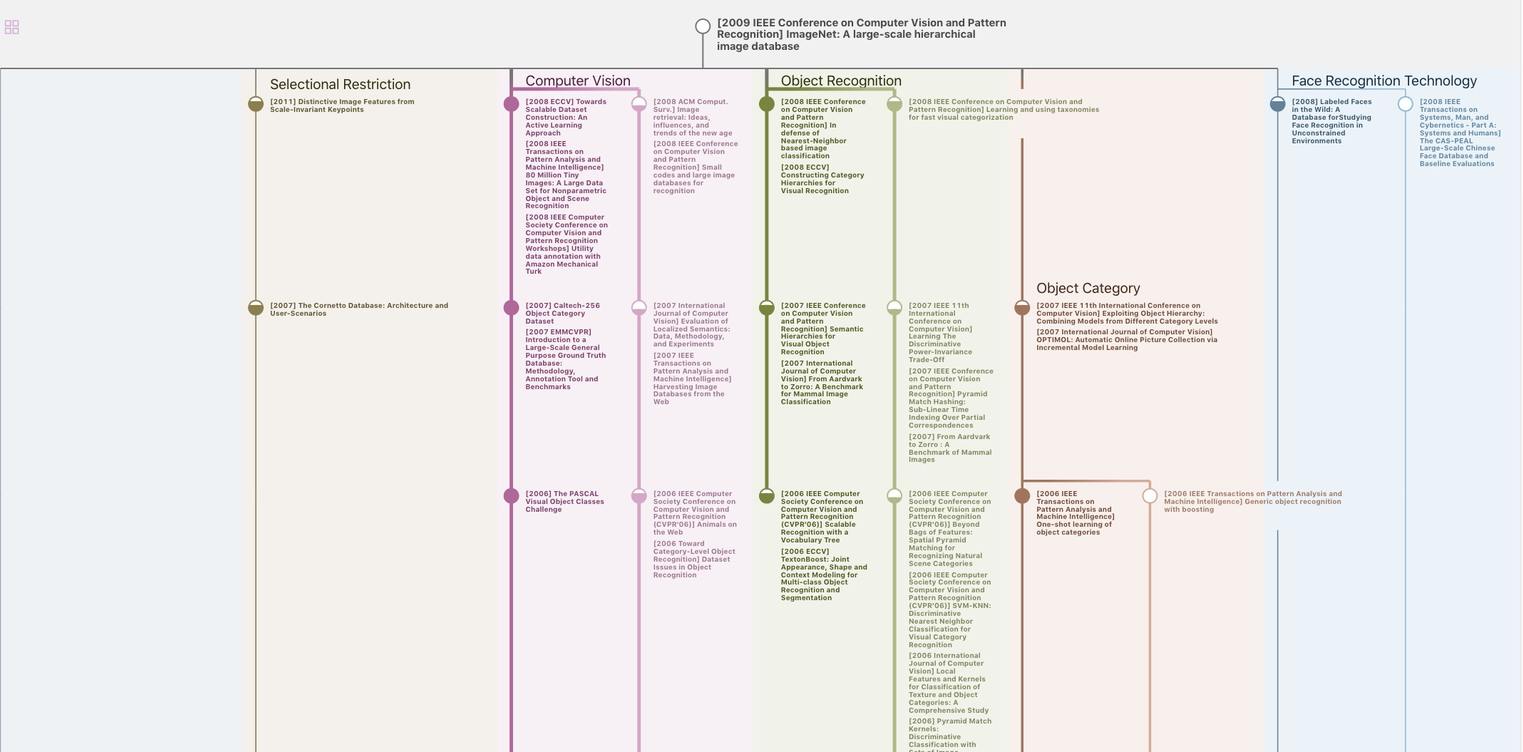
生成溯源树,研究论文发展脉络
Chat Paper
正在生成论文摘要