Utilizing BERT Intermediate Layers for Multimodal Sentiment Analysis
2022 IEEE International Conference on Multimedia and Expo (ICME)(2022)
摘要
Some recent works use pre-trained BERT to extract text features instead of the GloVe embedding representation, which greatly improves multimodal sentiment analysis. However, these works ignore BERT's intermediate layers information. The layers in BERT can capture phrase-level, syntax-level, and semantic-level information, respectively. Utilizing these levels of information in the multimodal fusion stage can lead to fine-grained fusion results and promote the potential of fine-tuning BERT on multimodal data. In this paper, we fuse middle layers information of BERT with non-verbal modalities in multiple stages via our designed hierarchical fusion structure external to BERT. In addition, the crossmodal fusion process runs the risk of discarding valid information of unimodality. We suggest distilling sentiment-relevant features from the removed information and restitute it to the network to promote sentiment analysis. Evaluating our proposed model on CMU-MOSI and CMU-MOSEI datasets, we show that it outperforms existing works and successfully fine-tunes BERT on multimodal language data.
更多查看译文
关键词
multimodal sentiment analysis,BERT,fine-tune,feature restitution
AI 理解论文
溯源树
样例
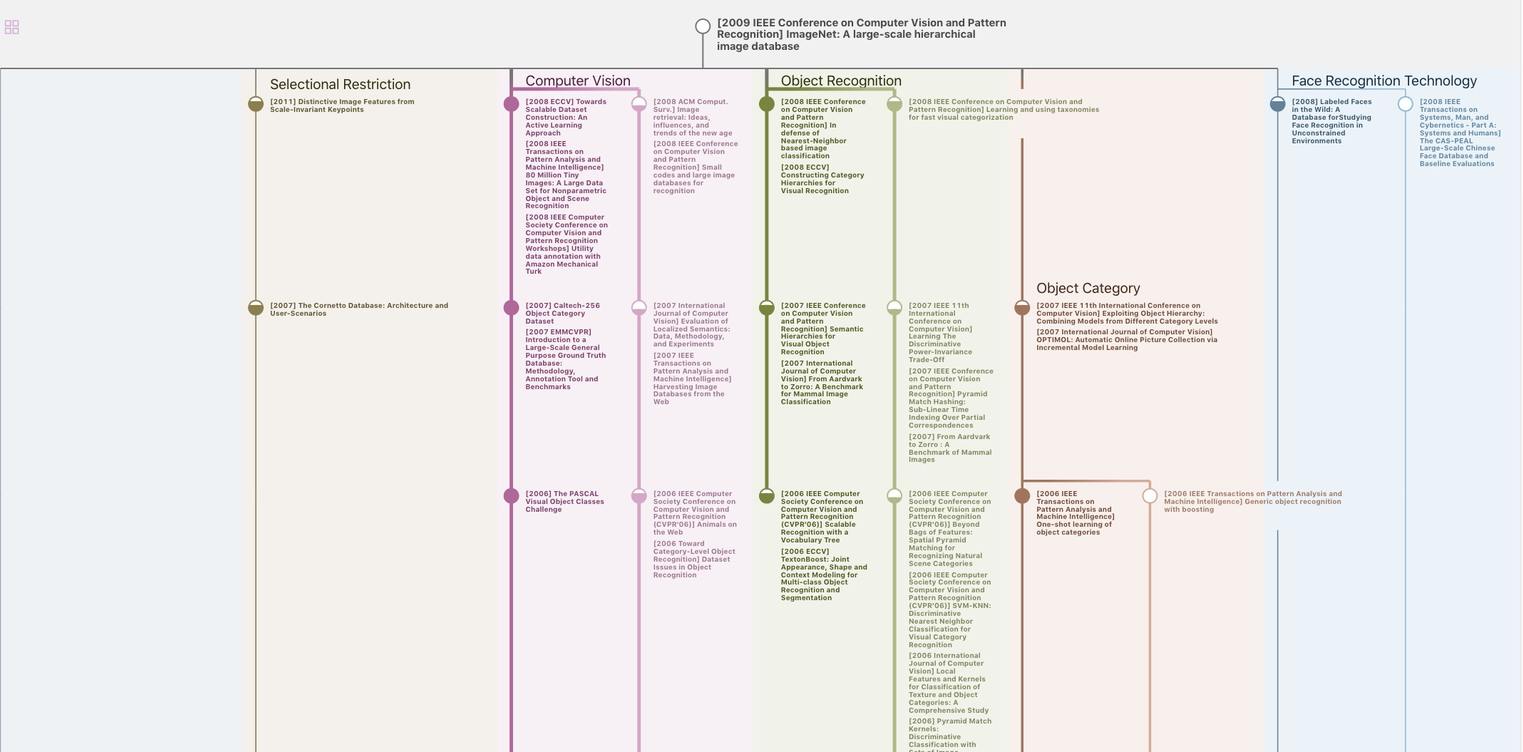
生成溯源树,研究论文发展脉络
Chat Paper
正在生成论文摘要