Noise-Tolerant Learning with Silhouette Coefficient for Unsupervised Person Re-Identification
2022 IEEE International Conference on Multimedia and Expo (ICME)(2022)
摘要
Unsupervised person re-identification (re-ID) attracts growing attention due to its broad prospects in practical applications. State-of-the-art unsupervised re-ID approaches combine clustering-based pseudo-label prediction with feature fine-tuning. However, pseudo labels generated directly by clustering are not always reliable and inevitably contain noisy labels. To tackle these challenges, we propose a novel noise inhibition framework to estimate the confidence of each pseudo label and actively correct noisy labels. By introducing the silhouette coefficient, our method can estimate the pseudo-label confidence without any extra model or data, and calculate the correction matrix to correct clustering results directly. However, the silhouette coefficient is usually applied on the hyper-parameters selection of clustering algorithms. In order to make the silhouette coefficient more suitable for estimation and correction tasks, we calibrate the Jaccard distance matrix to alleviate the negative influence of the cluster size on the silhouette coefficient. Our proposed method brings significant improvement and achieves the state-of-the-art performance on benchmark datasets.
更多查看译文
关键词
unsupervised person re-ID,clustering,silhouette coefficient
AI 理解论文
溯源树
样例
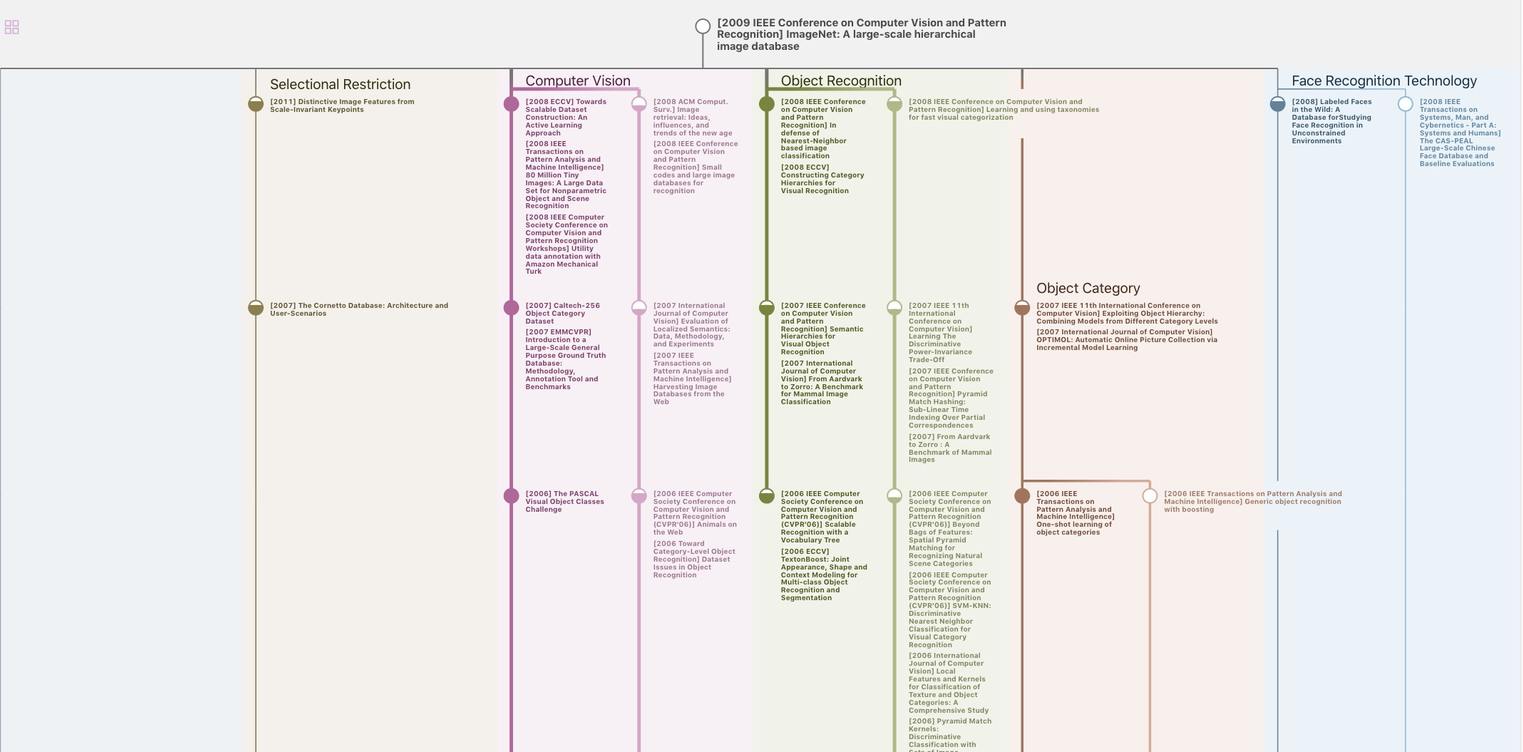
生成溯源树,研究论文发展脉络
Chat Paper
正在生成论文摘要