COMPOFF: A Compiler Cost model using Machine Learning to predict the Cost of OpenMP Offloading
2022 IEEE International Parallel and Distributed Processing Symposium Workshops (IPDPSW)(2022)
摘要
The HPC industry is inexorably moving towards an era of extremely heterogeneous architectures, with more devices configured on any given HPC platform and potentially more kinds of devices, some of them highly specialized. Writing a separate code suitable for each target system for a given HPC application is not practical. The better solution is to use directive-based parallel programming models such as OpenMP. OpenMP provides a number of options for offloading a piece of code to devices like GPUs. To select the best option from such options during compilation, most modern compilers use analytical models to estimate the cost of executing the original code and the different offloading code variants. Building such an analytical model for compilers is a difficult task that necessi-tates a lot of effort on the part of a compiler engineer. Recently, machine learning techniques have been successfully applied to build cost models for a variety of compiler optimization problems. In this paper, we present COMPOFF, a cost model that statically estimates the Cost of OpenMP OFFloading using a neural network model. We used six different transformations on a parallel code of Wilson Dslash Operator to support GPU offloading, and we predicted their cost of execution on different GPUs using COMPOFF during compile time. Our results show that this model can predict offloading costs with a root mean squared error in prediction of less than 0.5 seconds. Our preliminary findings indicate that this work will make it much easier and faster for scientists and compiler developers to port legacy HPC applications that use OpenMP to new heterogeneous computing environment.
更多查看译文
关键词
openmp,gpu,machine learning,cost model
AI 理解论文
溯源树
样例
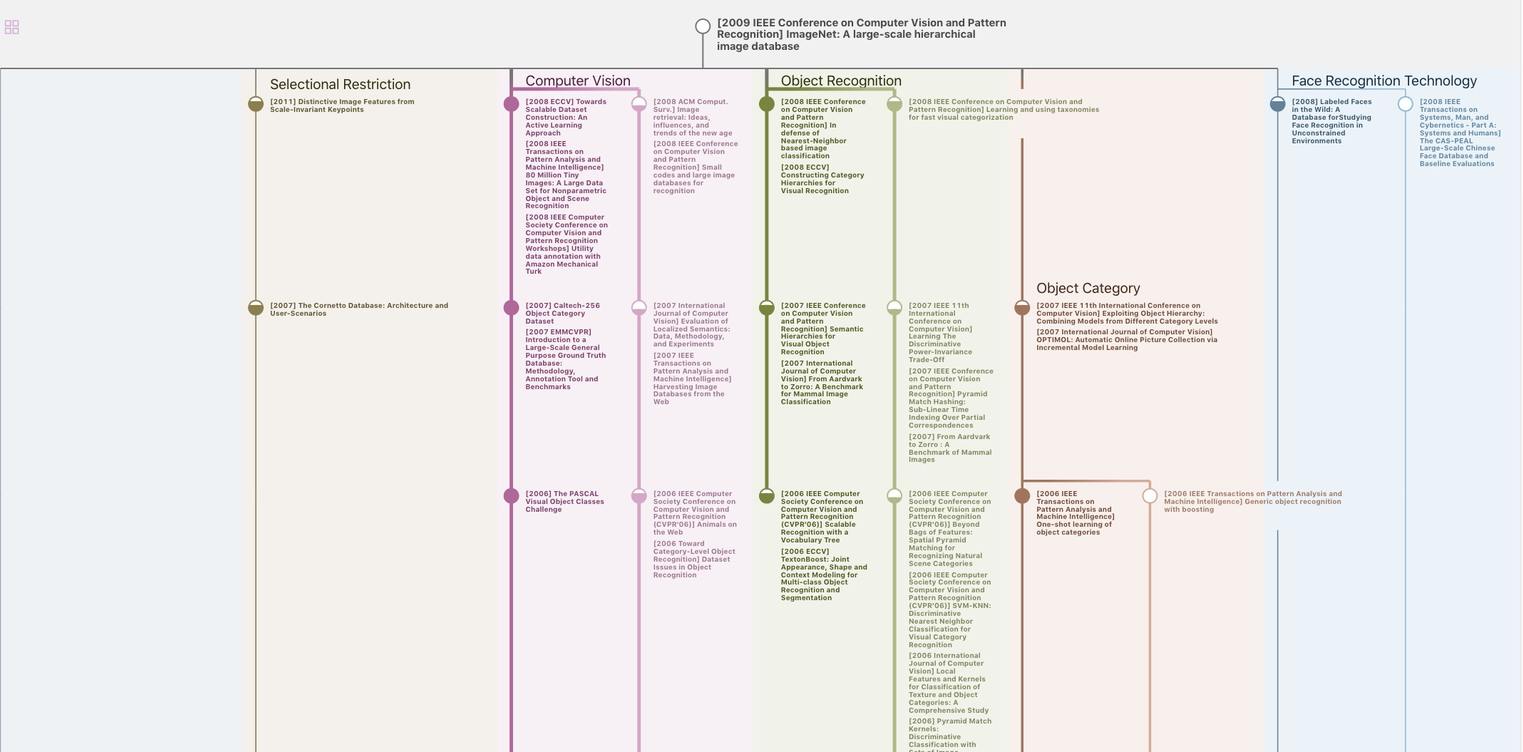
生成溯源树,研究论文发展脉络
Chat Paper
正在生成论文摘要