Using real-time feedback of L5/S1 compression force based on markerless optical motion capture to improve the lifting technique in manual materials handling
International Journal of Industrial Ergonomics(2022)
摘要
Challenges in manual materials handling (MMH) are posed in particular by the requirements for continuous repetition and individual feedback. Low effort in MMH instructions is accordingly a relevant factor. The combination of a markerless motion capture system with a biomechanical model providing visual MMH instructions by individual real-time feedback of the compression force of the intervertebral disc in L5/S1 (CF) could tackle these challenges. However, this raises the question of whether this approach provides appropriate MMH instructions to improve the lifting technique in MMH. Results of an experiment with 22 young male participants indicate that visual MMH instructions with such individual real-time feedback have significant advantages in improving the lifting technique by reducing those factors associated with lower back pain compared to instructions with a reference paper-based tutorial or a baseline without instructions. Thus, peak and mean CF and peak trunk flexion, for example, were significantly lower when lifting with individual real-time feedback of CF compared to other conditions tested. Hence, the results suggest that it may be sensible to improve the lifting technique by such an approach of MMH instructions and integrate it in MMH training programs or on-the-job training in order to reduce or prevent lower back pain.
Relevance to industry
Using real-time feedback of the compression force of the intervertebral disc of L5/S1 based on markerless optical motion capture can improve the lifting technique in manual materials handling. This may be integrated into MMH training programs or on-the-job training to reduce or prevent low back pain.
更多查看译文
关键词
Motion capture,Manual materials handling training,Lifting technique,Real-time feedback,Biomechanical analyses,Compression force
AI 理解论文
溯源树
样例
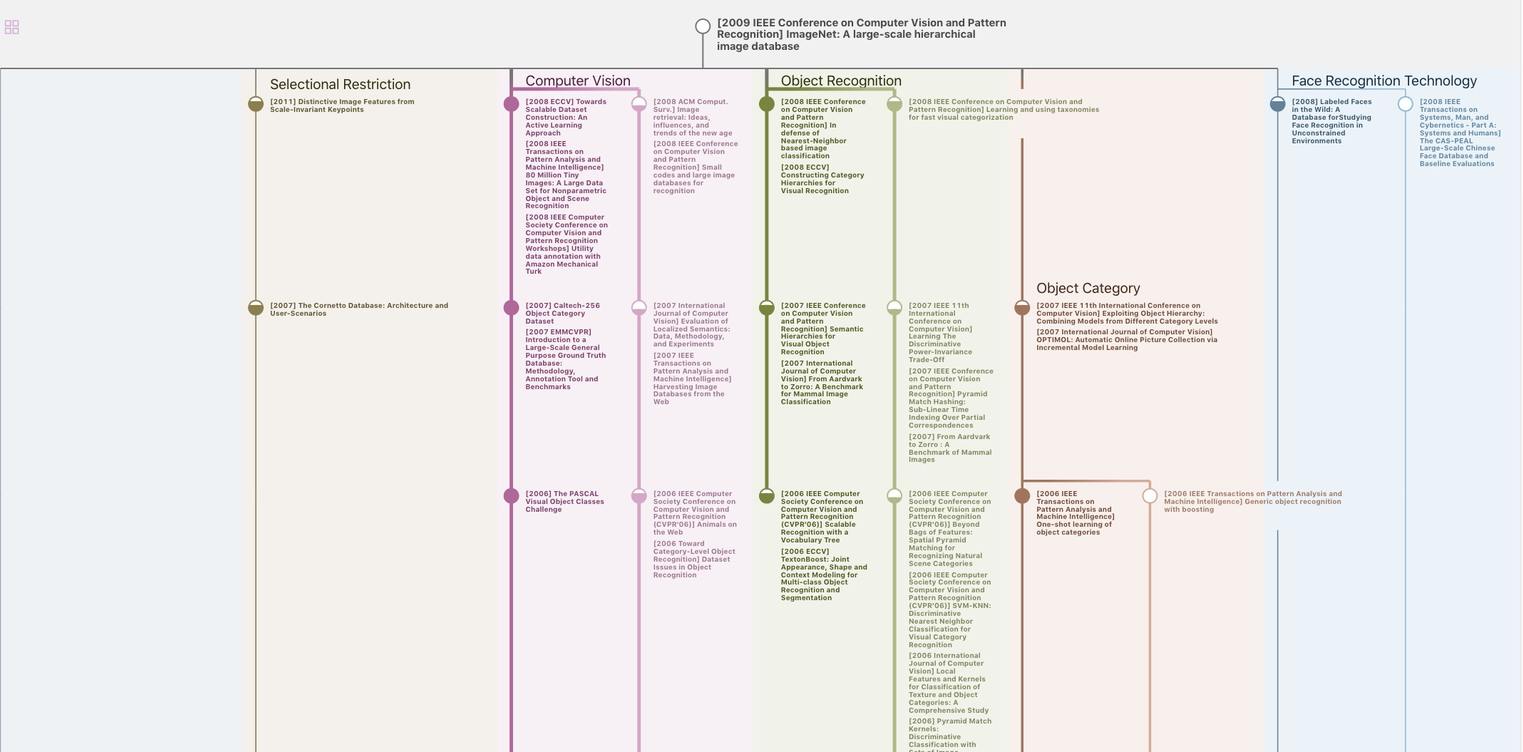
生成溯源树,研究论文发展脉络
Chat Paper
正在生成论文摘要