Development of Wiener-Hammerstein Models Parameterized using Orthonormal Basis Filters and Deep Neural Network
IFAC-PapersOnLine(2022)
摘要
Many chemical or biochemical processes exhibit strongly nonlinear dynamic behavior in the desired region of operations. To develop effective monitoring and control schemes for such systems, it is necessary to develop a reliable model that captures dynamics as well as the steady-state behavior over a wide range of operations. In this work, it is proposed to develop a block-oriented Wiener-Hammerstein model parameterized using generalized orthonormal basis filters and deep neural network (GOBF DNN). A two-step procedure is developed to select the generalized orthonormal basis filters (GOBF) pole locations and estimate the deep neural network (DNN) parameters. The e¢cacy of the proposed modeling strategy is demonstrated using the simulation study on a benchmark continuously operated fomenter system. The proposed GOBF DNN model is able to capture the dynamic and steady-state behavior of the plant over a wide range of operations. Comparison of performances based on the dynamic as well as the steady-state indices clearly underscores the advantages of using a DNN over a shallow neural net and a NARX model developed using DNN.
更多查看译文
关键词
Block Oriented Model,Weiner-Hammerstein Model,Deep Neural Network,Generalized Orthonormal Basis Filters
AI 理解论文
溯源树
样例
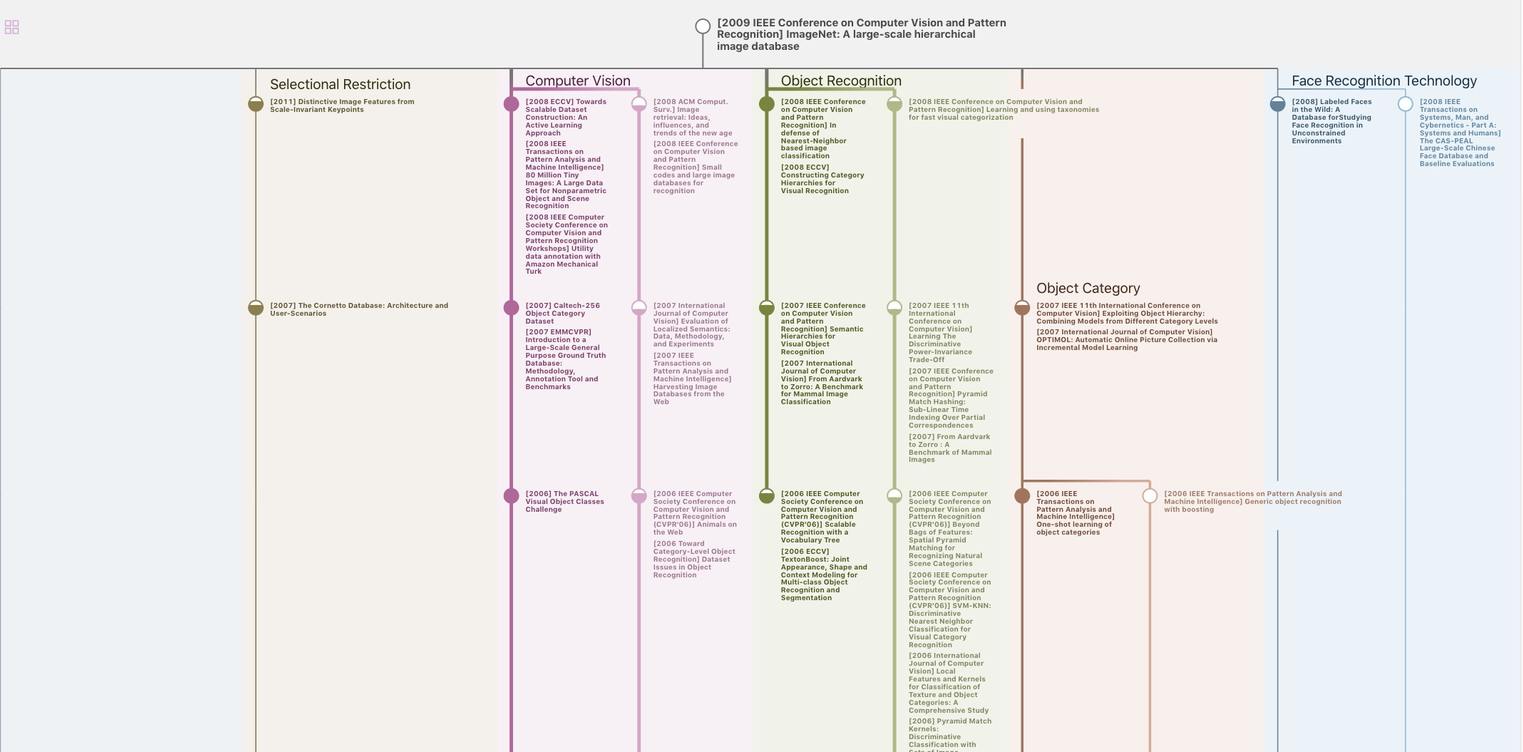
生成溯源树,研究论文发展脉络
Chat Paper
正在生成论文摘要