Efficient Comorbidity Analysis in Brain Disorders Reveals Better Diagnosis.
IEEE International Conference on High Performance Computing and Communications(2021)
摘要
Nowadays, brain disorders are gaining momentum faster than ever. Early detection of these disorders would be helpful in the treatment process. Also, detecting some comorbid brain disorders would be expensive and time-consuming. With advancements in machine learning (ML) and Artificial intelligence, these brain disorders and their comorbidities can be detected in the early stage. Different techniques of machine learning are used to detect Autism Spectrum Disorder (ASD), Attention deficit hyperactivity disorder (ADHD), Intellectual Disability (ID), and other brain disorders. This paper focuses on predicting ASD, ADHD, ID, and their Comorbidities via multi-stage analytical and prediction modelling. The first stage involves efficient data pre-processing. The next stage is a comorbidity analysis phase via logistic regression. In this analysis, logistic regression was applied to recognize health-related variables which are associated with ASD+ADHD+ID. These variables are Vision Test, Brain Injury, Anxiety, Down Syndrome, Blood Disorder, and Cystic Fibrosis. In the third stage, machine learning methods predict ASD, ADHD, ID for better diagnosis. For this purpose, SVM, KNN, and MLP are used. To evaluate these models, accuracy, precision, recall, and F1-score are selected.
更多查看译文
关键词
Comorbidities,Brain Disorders,Comorbidities Analysis,Machine learning,Diagnosis,Evaluation
AI 理解论文
溯源树
样例
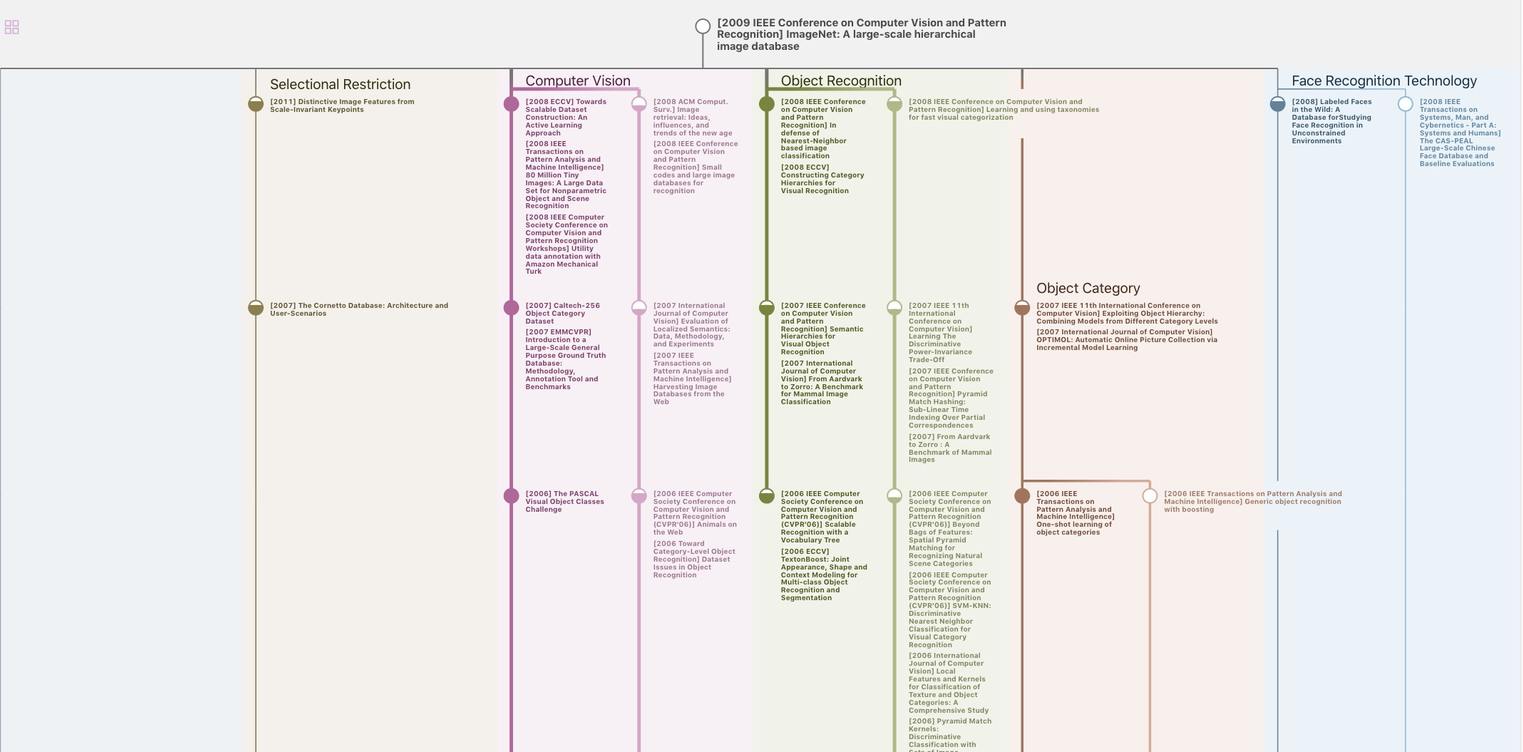
生成溯源树,研究论文发展脉络
Chat Paper
正在生成论文摘要