Machine Learning Methods for Survival Analysis with Clinical and Transcriptomics Data of Breast Cancer.
Methods in molecular biology (Clifton, N.J.)(2023)
摘要
Breast cancer is one of the most common cancers in women worldwide, which causes an enormous number of deaths annually. However, early diagnosis of breast cancer can improve survival outcomes enabling simpler and more cost-effective treatments. The recent increase in data availability provides unprecedented opportunities to apply data-driven and machine learning methods to identify early-detection prognostic factors capable of predicting the expected survival and potential sensitivity to treatment of patients, with the final aim of enhancing clinical outcomes. This tutorial presents a protocol for applying machine learning models in survival analysis for both clinical and transcriptomic data. We show that integrating clinical and mRNA expression data is essential to explain the multiple biological processes driving cancer progression. Our results reveal that machine-learning-based models such as random survival forests, gradient boosted survival model, and survival support vector machine can outperform the traditional statistical methods, i.e., Cox proportional hazard model. The highest C-index among the machine learning models was recorded when using survival support vector machine, with a value 0.688, whereas the C-index recorded using the Cox model was 0.677. Shapley Additive Explanation (SHAP) values were also applied to identify the feature importance of the models and their impact on the prediction outcomes.
更多查看译文
关键词
Breast cancer,Data integration,Interpretability,Machine learning,Survival analysis
AI 理解论文
溯源树
样例
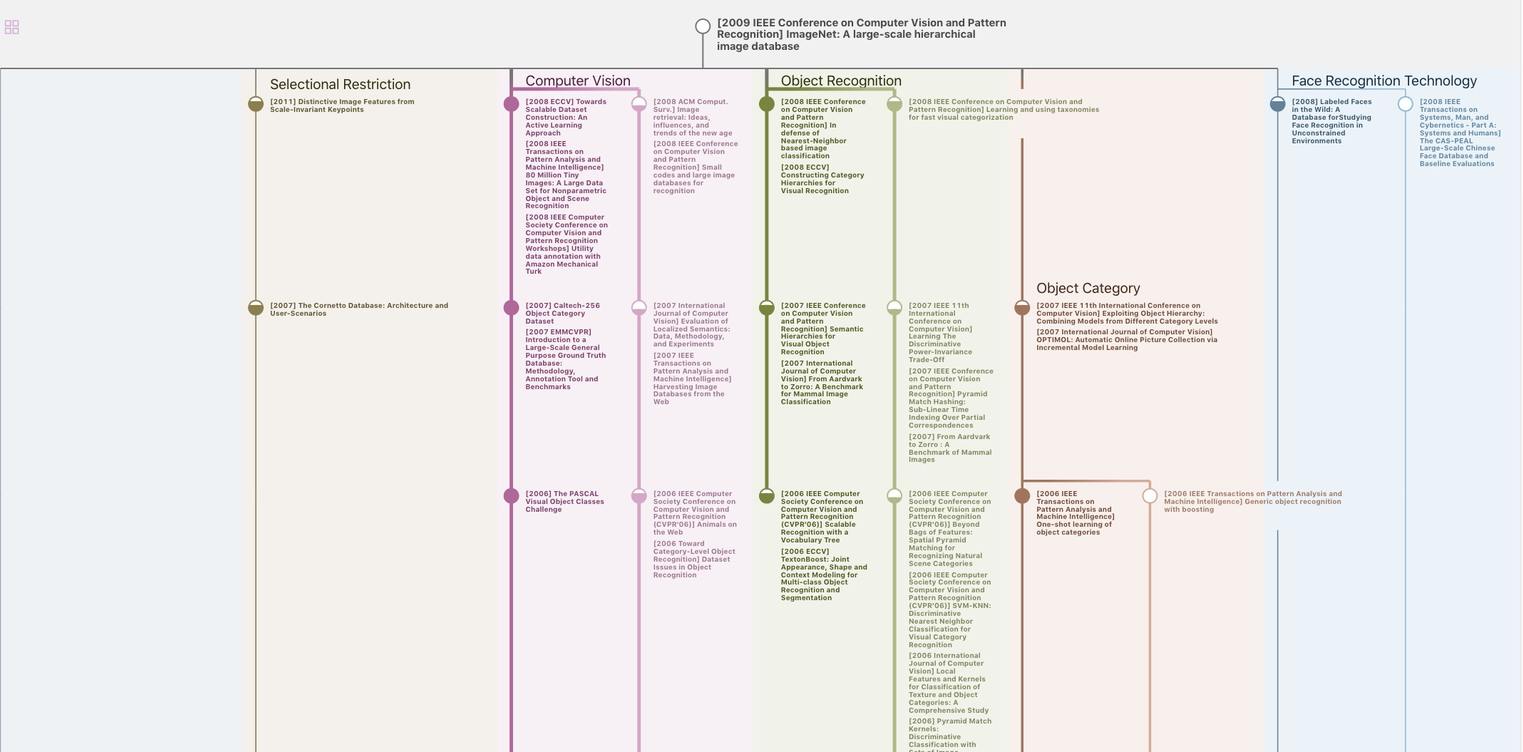
生成溯源树,研究论文发展脉络
Chat Paper
正在生成论文摘要