Masked inverse folding with sequence transfer for protein representation learning
biorxiv(2022)
摘要
Self-supervised pretraining on protein sequences has led to state-of-the art performance on protein function and fitness prediction. However, sequence-only methods ignore the rich information contained in experimental and predicted protein structures. Meanwhile, inverse folding methods reconstruct a protein’s amino-acid sequence given its structure, but do not take advantage of sequences that do not have known structures. In this study, we train a masked inverse folding protein language model parameterized as a structured graph neural network. We then show that using the outputs from a pretrained sequence-only protein masked language model as input to the inverse folding model further improves pretraining perplexity. We evaluate both of these models on downstream protein engineering tasks and analyze the effect of using information from experimental or predicted structures on performance.
### Competing Interest Statement
The authors have declared no competing interest.
更多查看译文
关键词
protein representation learning,inverse folding,sequence transfer
AI 理解论文
溯源树
样例
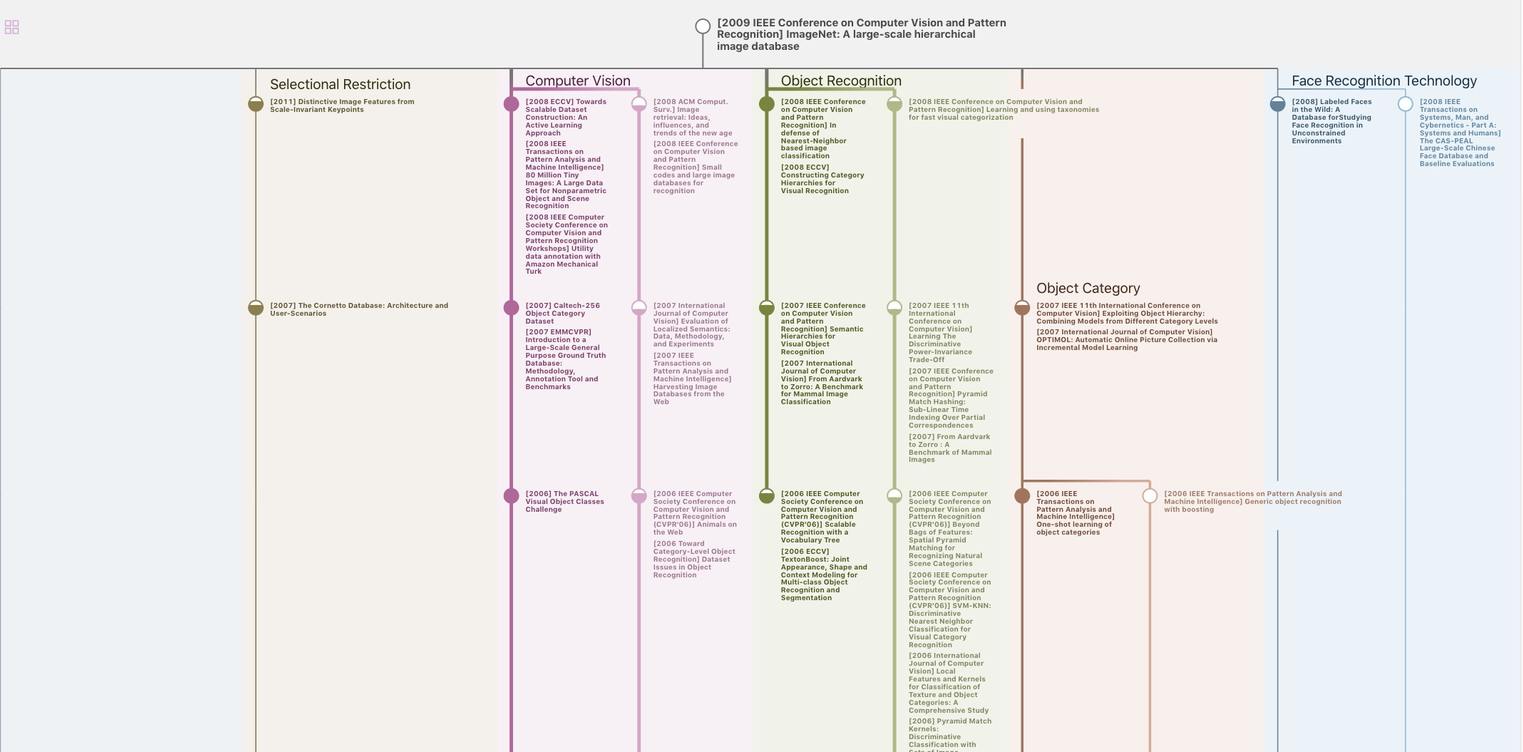
生成溯源树,研究论文发展脉络
Chat Paper
正在生成论文摘要