Prototype contrastive learning for point-supervised temporal action detection
Expert Systems with Applications(2023)
摘要
Detecting temporal actions in a video with only single-frame annotation in each action instance or segment, a.k.a., point-level supervision, has emerged as a more challenging task, compared to fully-supervised setting where per-frame annotations are available. Generally, it faces the label sparsity problem and the serious class-imbalance problem which are not fully explored in previous works. To address them, this paper develops an efficient pseudo-label generation approach to yield more positive samples and negative samples for providing supervision, i.e., the Prototype Contrastive Learning (PCL) based point-supervised temporal action detection framework. PCL aims at explicitly discovering the class relations between labeled and unlabeled frames by adopting prototype learning, and generates pseudo labels by estimating the semantic similarity of pair-wise frames in the embedding space. Meanwhile, it imposes the class relation constraint onto the action and background prototypes by introducing contrastive representation learning, i.e., the prototypes in distinct classes are pushed away and those within the same class are pulled closer. This allows learning the discriminative representations of prototypes that well comply with the data distribution of video frames. These prototype representations are treated as the hidden pattern proxies of different classes, and their semantic relations help to generate pseudo labels for unlabeled frames. Empirical studies on three benchmarks including GTEA, BEOID, and THUMOS14, have demonstrated the favorable performance of the proposed method.
更多查看译文
关键词
Point-level supervision,Prototype learning,Contrastive learning,Pseudo-label learning,Temporal action detection
AI 理解论文
溯源树
样例
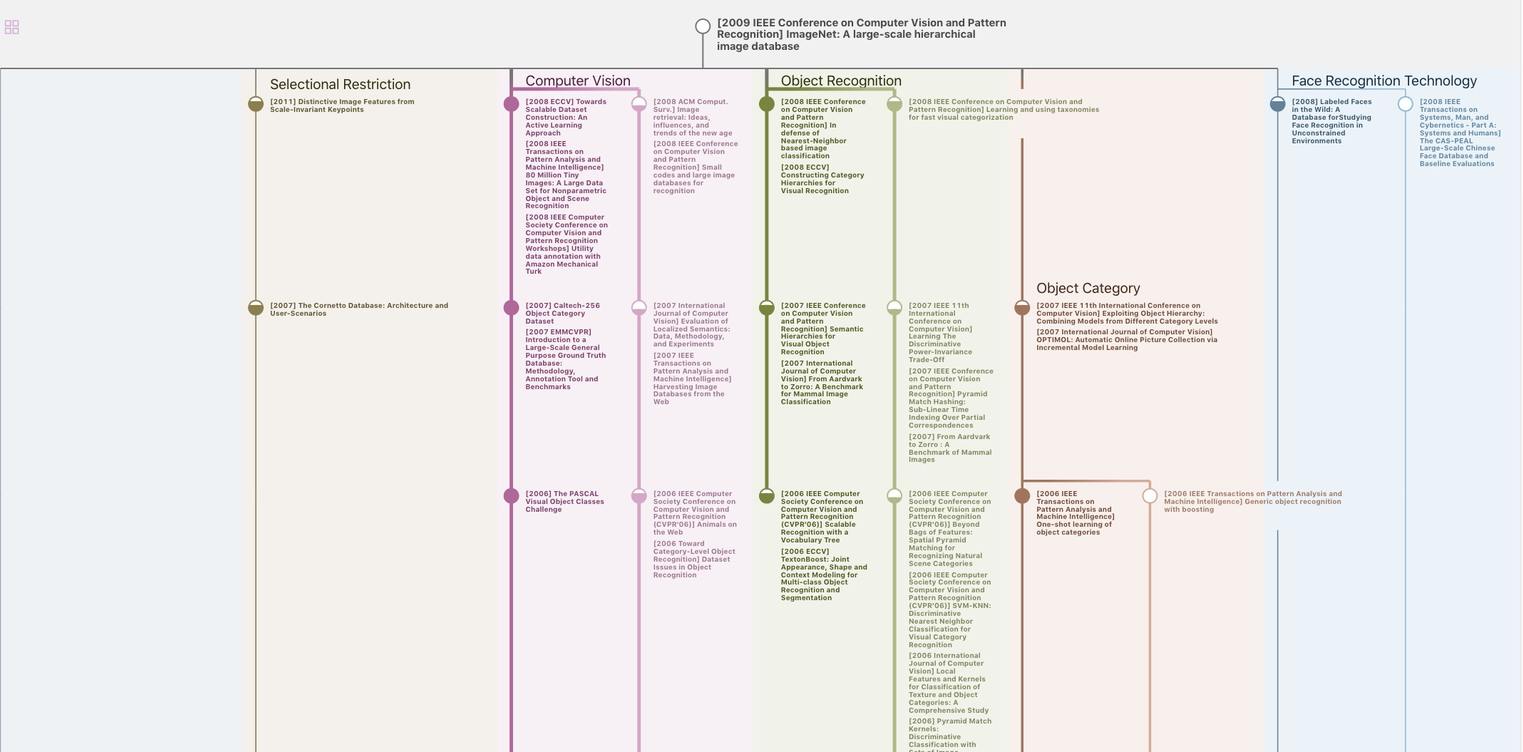
生成溯源树,研究论文发展脉络
Chat Paper
正在生成论文摘要