GenSLMs: Genome-scale language models reveal SARS-CoV-2 evolutionary dynamics
biorxiv(2023)
摘要
We seek to transform how new and emergent variants of pandemic-causing viruses, specifically SARS-CoV-2, are identified and classified. By adapting large language models (LLMs) for genomic data, we build genome-scale language models (GenSLMs) which can learn the evolutionary landscape of SARS-CoV-2 genomes. By pre-training on over 110 million prokaryotic gene sequences and fine-tuning a SARS-CoV-2-specific model on 1.5 million genomes, we show that GenSLMs can accurately and rapidly identify variants of concern. Thus, to our knowledge, GenSLMs represents one of the first whole-genome scale foundation models which can generalize to other prediction tasks. We demonstrate scaling of GenSLMs on GPU-based supercomputers and AI-hardware accelerators utilizing 1.63 Zettaflops in training runs with a sustained performance of 121 PFLOPS in mixed precision and peak of 850 PFLOPS. We present initial scientific insights from examining GenSLMs in tracking evolutionary dynamics of SARS-CoV-2, paving the path to realizing this on large biological data.
更多查看译文
关键词
SARS-CoV-2,COVID-19,HPC,AI,large language models,whole-genome analyses
AI 理解论文
溯源树
样例
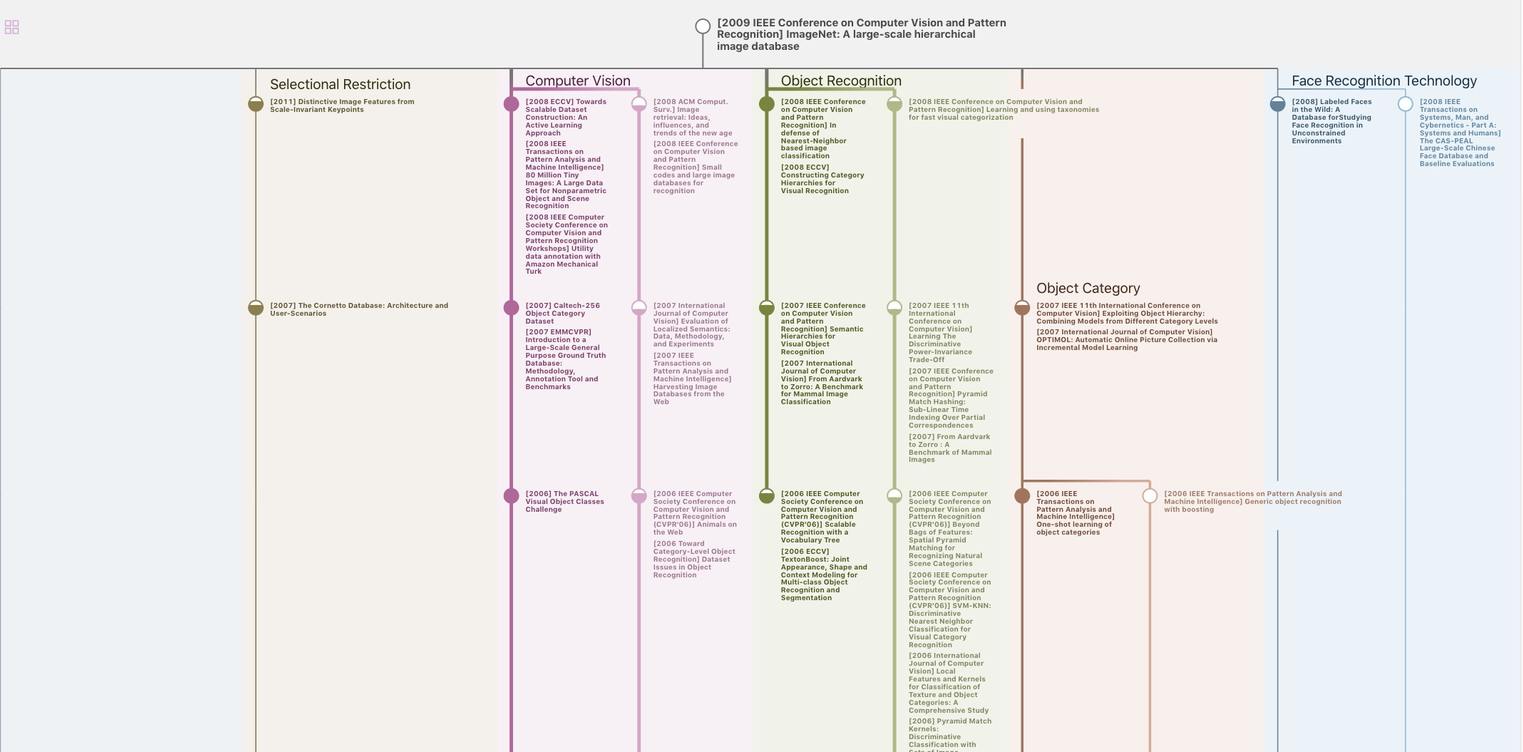
生成溯源树,研究论文发展脉络
Chat Paper
正在生成论文摘要