Nonparameteric Event-Triggered Learning With Applications to Adaptive Model Predictive Control
IEEE Transactions on Automatic Control(2023)
摘要
In this article, an event-triggered online learning problem for Lipschitz continuous systems with nonlinear model mismatch is considered, with the aim of building a data-efficient nonparameteric estimation approach for learning-based control. The system considered is composed of known linear dynamics and unknown nonlinearity, and the main focus of this work includes the design and analysis of event-triggered learning mechanisms, and the application of the learning method to adaptive model predictive control (MPC). First, a sample grid-based event-triggering mechanism and a prediction uncertainty-based event-triggering mechanisms are designed on the basis of the lazily adapted constant kinky inference framework. Then, the properties of the designed event-triggered learning methods are analyzed, and it is proved that the proposed approach provides error-bounded predictions with limited computational complexity. Third, a tube-based adaptive MPC design approach is developed utilizing the proposed event-triggered learning approach, and the closed-loop stability of the adaptive MPC is analyzed and proved based on the properties of the event-triggered learning algorithms. Implementation issues are discussed, and the effectiveness of the results is illustrated by numerical examples and comparative simulations.
更多查看译文
关键词
Adaptive model predictive control (MPC),event-based estimation,event-triggered learning,nonparameteric estimation
AI 理解论文
溯源树
样例
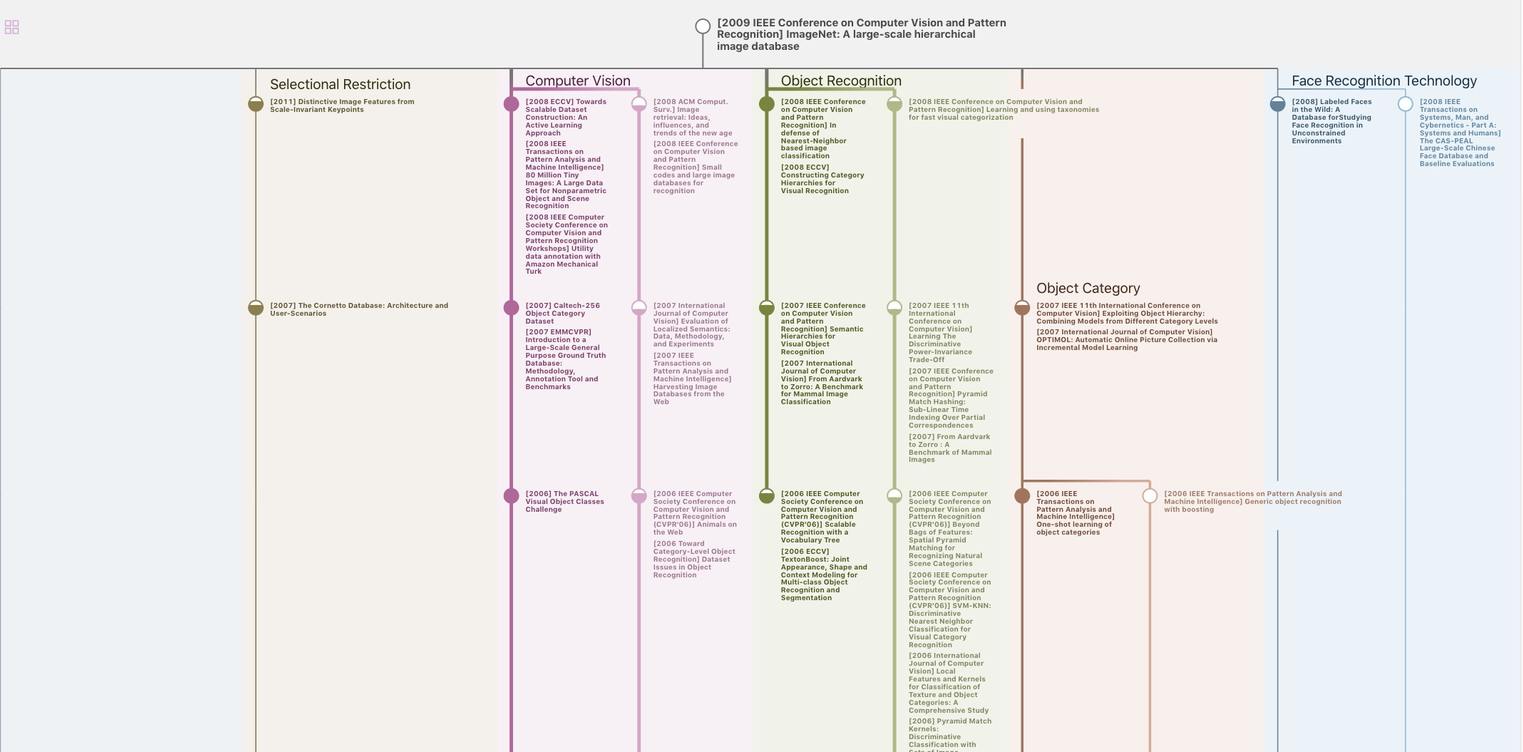
生成溯源树,研究论文发展脉络
Chat Paper
正在生成论文摘要