SaiT: Sparse Vision Transformers through Adaptive Token Pruning
arxiv(2022)
摘要
While vision transformers have achieved impressive results, effectively and efficiently accelerating these models can further boost performances. In this work, we propose a dense/sparse training framework to obtain a unified model, enabling weight sharing across various token densities. Thus one model offers a range of accuracy and throughput tradeoffs for different applications. Besides, we introduce adaptive token pruning to optimize the patch token sparsity based on the input image. In addition, we investigate knowledge distillation to enhance token selection capability in early transformer modules. Sparse adaptive image Transformer (SaiT) offers varying levels of model acceleration by merely changing the token sparsity on the fly. Specifically, SaiT reduces the computation complexity (FLOPs) by 39% - 43% and increases the throughput by 67% - 91% with less than 0.5% accuracy loss for various vision transformer models. Meanwhile, the same model also provides the zero accuracy drop option by skipping the sparsification step. SaiT achieves better accuracy and computation tradeoffs than state-of-the-art transformer and convolutional models.
更多查看译文
关键词
sparse vision transformers,adaptive token pruning
AI 理解论文
溯源树
样例
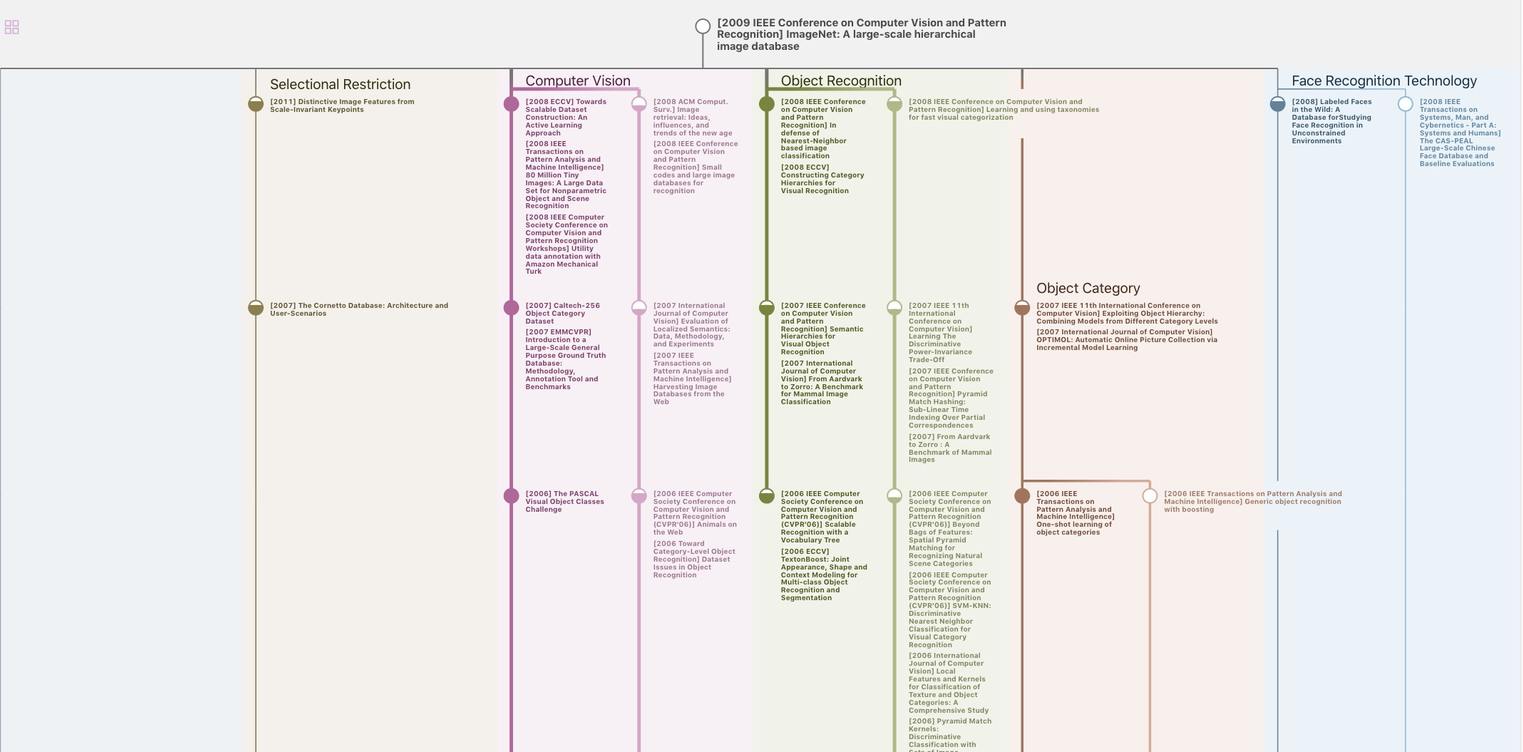
生成溯源树,研究论文发展脉络
Chat Paper
正在生成论文摘要