Manifold clustering optimized by adaptive aggregation strategy
Knowledge and Information Systems(2022)
摘要
Different from general spherical datasets, manifold datasets have a more complex spatial manifold structure, which makes it difficult to distinguish sample points on different manifold structures by Euclidean distance. Although the density peak clustering (DPC, two parameters: the cut-off ratio dc and the number of class centers C ) algorithm can search for density peaks quickly and assign sample points, it cannot identify clusters effectively with complex manifold structures due to the sample similarity measurement only based on Euclidean distance. To solve these problems, this paper proposes a Manifold Clustering optimized by Adaptive Aggregation Strategy (MC-AAS, two parameters: the number of nearest neighbors k and the threshold ratio of core points p ). Firstly, it introduces a novel manifold similarity measurement based on the shared nearest neighbors and redefines the local density of sample points by summing the manifold similarity. Secondly, the core points are determined by the statistical characteristics of local density, and the local sub-clusters of manifold structural datasets are obtained by means of the nearest neighbor connection of the core points. And then, the initial clusters are merged on the basis of the statistical test of boundary density and the silhouette coefficient of adjacent subclass to realize the identification of manifold structural datasets. Finally, based on three evaluation metrics: Adjusted Mutual Information, Adjusted Rand Index and Fowlkes-Mallows Index, we conduct extensive experiments on synthetic datasets and real-world datasets. The experimental results indicate that, compared with current methods, the MC-AAS algorithm achieves a better clustering effect in identifying complex manifold datasets and has better robustness.
更多查看译文
关键词
Complex manifold,Shared neighbors,Neighbor connection,Boundary density,Statistical test
AI 理解论文
溯源树
样例
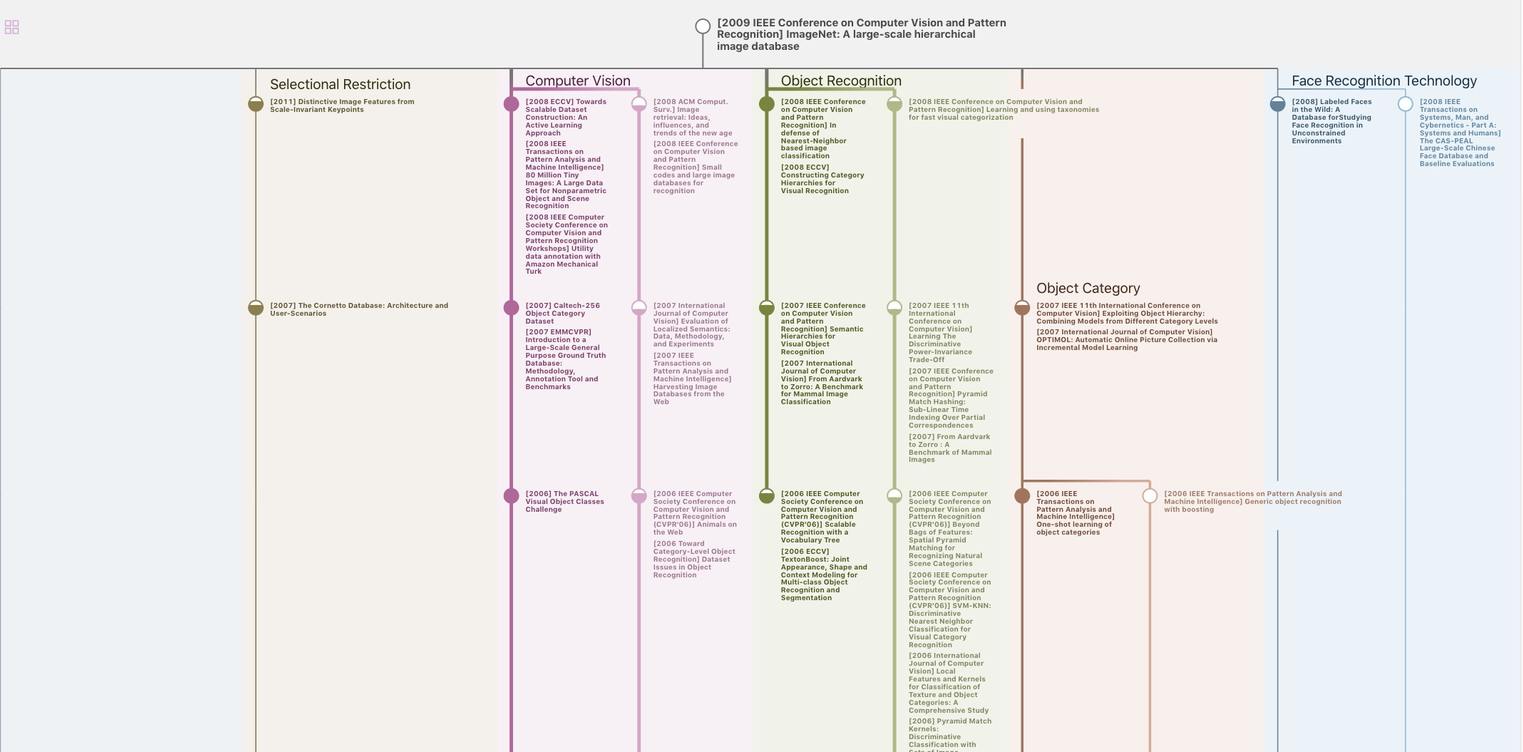
生成溯源树,研究论文发展脉络
Chat Paper
正在生成论文摘要