PRIOR: Deep Reinforced Adaptive Video Streaming with Attention-Based Throughput Prediction
PROCEEDINGS OF THE 32ND WORKSHOP ON NETWORK AND OPERATING SYSTEMS SUPPORT FOR DIGITAL AUDIO AND VIDEO, NOSSDAV 2022(2022)
摘要
Video service providers have deployed dynamic video bitrate adaptation services to fulfill user demands for higher video quality. However, fluctuations and instability of network conditions inhibit the performance promotion of adaptive bitrate (ABR) algorithms. Existing rule-based approaches fail to guarantee accurate throughput estimates, and learning-based algorithms are considerably sensitive to the variability of network. Therefore, how to gain effective and stable throughput estimates has become one of the critical challenges to further enhancing ABR methods. To eliminate this concern, we propose PRIOR, an ABR algorithm that fuses an effective throughput prediction module and a state-of-the-art multi-agent reinforcement learning method to provide a high quality of experience (QoE). PRIOR aims to maximize the QoE metric by straightforwardly utilizing accurate throughput estimates rather than past throughput measurements. Specifically, PRIOR employs a light-weighted prediction module with attention mechanism to obtain effective future throughput. Considering the excellent features introduced by the HTTP/3 protocol, we apply PRIOR to trace-driven simulations and real-world scenarios over HTTP/1.1 and HTTP/3. Trace-driven emulation illustrates that PRIOR outperforms existing ABR schemes over HTTP/1.1 and HTTP/3, and our prediction module can also reinforce the performance of other ABR algorithms. Extensive results on real-world evaluation demonstrate the superiority of PRIOR over existing state-of-the-art ABR schemes.
更多查看译文
关键词
Throughput Prediction,Bitrate Adaptation,Reinforcement Learning,HTTP/3 Protocol
AI 理解论文
溯源树
样例
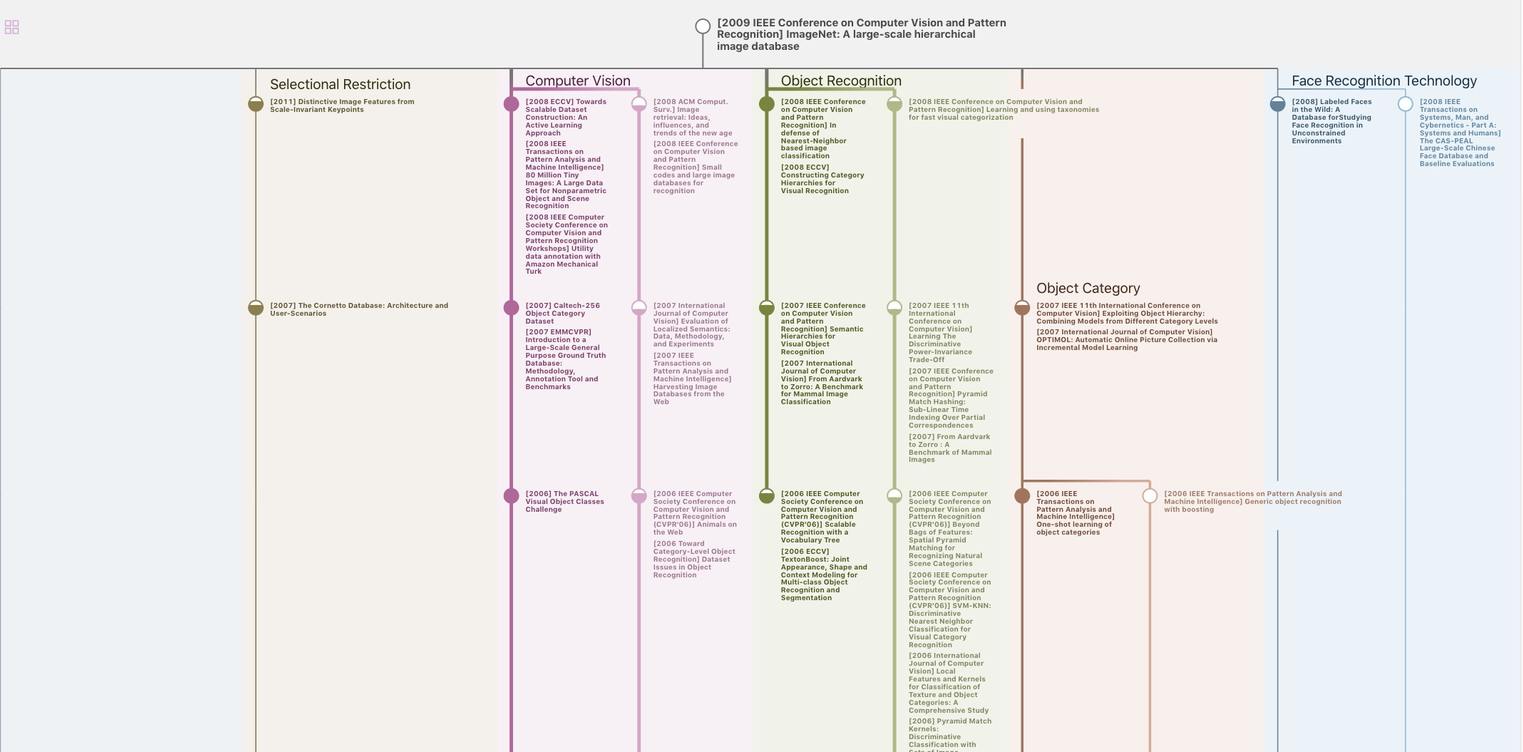
生成溯源树,研究论文发展脉络
Chat Paper
正在生成论文摘要